PRROC: computing and visualizing precision-recall and receiver operating characteristic curves in R
- PMID: 25810428
- PMCID: PMC4514923
- DOI: 10.1093/bioinformatics/btv153
PRROC: computing and visualizing precision-recall and receiver operating characteristic curves in R
Abstract
Precision-recall (PR) and receiver operating characteristic (ROC) curves are valuable measures of classifier performance. Here, we present the R-package PRROC, which allows for computing and visualizing both PR and ROC curves. In contrast to available R-packages, PRROC allows for computing PR and ROC curves and areas under these curves for soft-labeled data using a continuous interpolation between the points of PR curves. In addition, PRROC provides a generic plot function for generating publication-quality graphics of PR and ROC curves.
© The Author 2015. Published by Oxford University Press.
Figures
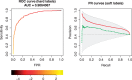
Similar articles
-
ROCR: visualizing classifier performance in R.Bioinformatics. 2005 Oct 15;21(20):3940-1. doi: 10.1093/bioinformatics/bti623. Epub 2005 Aug 11. Bioinformatics. 2005. PMID: 16096348
-
An Excel program for calculating and plotting receiver-operator characteristic (ROC) curves, histograms and descriptive statistics.Comput Biol Med. 1994 May;24(3):167-9. doi: 10.1016/0010-4825(94)90012-4. Comput Biol Med. 1994. PMID: 7924261
-
pROC: an open-source package for R and S+ to analyze and compare ROC curves.BMC Bioinformatics. 2011 Mar 17;12:77. doi: 10.1186/1471-2105-12-77. BMC Bioinformatics. 2011. PMID: 21414208 Free PMC article.
-
Receiver operating characteristic (ROC) curve: practical review for radiologists.Korean J Radiol. 2004 Jan-Mar;5(1):11-8. doi: 10.3348/kjr.2004.5.1.11. Korean J Radiol. 2004. PMID: 15064554 Free PMC article. Review.
-
A program for computing the prediction probability and the related receiver operating characteristic graph.Anesth Analg. 2010 Dec;111(6):1416-21. doi: 10.1213/ANE.0b013e3181fb919e. Epub 2010 Nov 8. Anesth Analg. 2010. PMID: 21059744 Review.
Cited by
-
Early prediction of acute pancreatitis with acute kidney injury using abdominal contrast-enhanced CT features.iScience. 2024 Sep 27;27(10):111058. doi: 10.1016/j.isci.2024.111058. eCollection 2024 Oct 18. iScience. 2024. PMID: 39435145 Free PMC article.
-
Parallel genome-scale CRISPR-Cas9 screens uncouple human pluripotent stem cell identity versus fitness.Nat Commun. 2024 Oct 17;15(1):8966. doi: 10.1038/s41467-024-53284-4. Nat Commun. 2024. PMID: 39419994 Free PMC article.
-
Normalization of short-chain fatty acid concentration by bacterial count of stool samples improves discrimination between eubiotic and dysbiotic gut microbiota caused by Clostridioides difficile infection.Gut Microbes. 2024 Jan-Dec;16(1):2415488. doi: 10.1080/19490976.2024.2415488. Epub 2024 Oct 12. Gut Microbes. 2024. PMID: 39395000 Free PMC article.
-
Identifying images in the biology literature that are problematic for people with a color-vision deficiency.Elife. 2024 Sep 11;13:RP95524. doi: 10.7554/eLife.95524. Elife. 2024. PMID: 39259199 Free PMC article.
-
Systematic identification of post-transcriptional regulatory modules.Nat Commun. 2024 Sep 9;15(1):7872. doi: 10.1038/s41467-024-52215-7. Nat Commun. 2024. PMID: 39251607 Free PMC article.
References
-
- Boyd K., et al. . (2013) Area under the precision-recall curve: point estimates and confidence intervals. In: Blockeel H., Kersting K., Nijssen S., Železný F. (ed.) Machine Learning and Knowledge Discovery in Databases. Vol. 8190 of LNCS. Springer, Berlin, pp. 451–466.
-
- Davis J., Goadrich M. (2006) The relationship between precision-recall and ROC curves. In: Proceedings of the 23rd International Conference on Machine Learning. ACM, New York, pp. 233–240.
-
- Davis J., et al. . (2005) View learning for statistical relational learning: with an application to mammography. In: Proceeding of the 19th International Joint Conference on Artificial Intelligence, pp. 677–683.
MeSH terms
LinkOut - more resources
Full Text Sources
Other Literature Sources
Research Materials