Identification of active transcriptional regulatory elements from GRO-seq data
- PMID: 25799441
- PMCID: PMC4507281
- DOI: 10.1038/nmeth.3329
Identification of active transcriptional regulatory elements from GRO-seq data
Abstract
Modifications to the global run-on and sequencing (GRO-seq) protocol that enrich for 5'-capped RNAs can be used to reveal active transcriptional regulatory elements (TREs) with high accuracy. Here, we introduce discriminative regulatory-element detection from GRO-seq (dREG), a sensitive machine learning method that uses support vector regression to identify active TREs from GRO-seq data without requiring cap-based enrichment (https://github.com/Danko-Lab/dREG/). This approach allows TREs to be assayed together with gene expression levels and other transcriptional features in a single experiment. Predicted TREs are more enriched for several marks of transcriptional activation—including expression quantitative trait loci, disease-associated polymorphisms, acetylated histone 3 lysine 27 (H3K27ac) and transcription factor binding—than those identified by alternative functional assays. Using dREG, we surveyed TREs in eight human cell types and provide new insights into global patterns of TRE function.
Figures
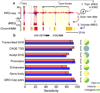
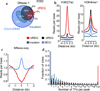
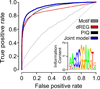
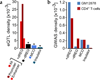
Similar articles
-
Discovering Transcriptional Regulatory Elements From Run-On and Sequencing Data Using the Web-Based dREG Gateway.Curr Protoc Bioinformatics. 2019 Jun;66(1):e70. doi: 10.1002/cpbi.70. Epub 2018 Dec 27. Curr Protoc Bioinformatics. 2019. PMID: 30589513 Free PMC article.
-
Widespread priming of transcriptional regulatory elements by incipient accessibility or RNA polymerase II pause in early embryos of the sea urchin Strongylocentrotus purpuratus.Genetics. 2023 Oct 4;225(2):iyad145. doi: 10.1093/genetics/iyad145. Genetics. 2023. PMID: 37551428 Free PMC article.
-
Mapping Transcription Regulation with Run-on and Sequencing Data Using the Web-Based tfTarget Gateway.Methods Mol Biol. 2023;2599:215-226. doi: 10.1007/978-1-0716-2847-8_15. Methods Mol Biol. 2023. PMID: 36427152
-
Identification of regulatory elements from nascent transcription using dREG.Genome Res. 2019 Feb;29(2):293-303. doi: 10.1101/gr.238279.118. Epub 2018 Dec 20. Genome Res. 2019. PMID: 30573452 Free PMC article.
-
Uncovering drug-responsive regulatory elements.Pharmacogenomics. 2015 Nov;16(16):1829-41. doi: 10.2217/pgs.15.121. Epub 2015 Nov 10. Pharmacogenomics. 2015. PMID: 26555224 Free PMC article. Review.
Cited by
-
Mapping catalytically engaged TOP2B in neurons reveals the principles of topoisomerase action within the genome.Cell Rep. 2024 Feb 27;43(2):113809. doi: 10.1016/j.celrep.2024.113809. Epub 2024 Feb 19. Cell Rep. 2024. PMID: 38377005 Free PMC article.
-
Transcription factor antagonism regulates heterogeneity in embryonic stem cell states.Mol Cell. 2022 Dec 1;82(23):4410-4427.e12. doi: 10.1016/j.molcel.2022.10.022. Epub 2022 Nov 9. Mol Cell. 2022. PMID: 36356583 Free PMC article.
-
Nascent RNA analyses: tracking transcription and its regulation.Nat Rev Genet. 2019 Dec;20(12):705-723. doi: 10.1038/s41576-019-0159-6. Epub 2019 Aug 9. Nat Rev Genet. 2019. PMID: 31399713 Free PMC article. Review.
-
A comparison of experimental assays and analytical methods for genome-wide identification of active enhancers.Nat Biotechnol. 2022 Jul;40(7):1056-1065. doi: 10.1038/s41587-022-01211-7. Epub 2022 Feb 17. Nat Biotechnol. 2022. PMID: 35177836 Free PMC article.
-
Integrating the Epigenome and Transcriptome of Hepatocellular Carcinoma to Identify Systematic Enhancer Aberrations and Establish an Aberrant Enhancer-Related Prognostic Signature.Front Cell Dev Biol. 2022 Mar 1;10:827657. doi: 10.3389/fcell.2022.827657. eCollection 2022. Front Cell Dev Biol. 2022. PMID: 35300417 Free PMC article.
References
References (supplemental)
-
- Dimitriadou E, Hornik K, Leisch F, Meyer D, Weingessel A. e1071: Misc Functions of the Department of Statistics (e1071), TU Wien. 2010
-
- Chang C-C, Lin C-J. LIBSVM. ACM Trans. Intell. Syst. Technol. 2011;2:1–27.
Publication types
MeSH terms
Substances
Associated data
- Actions
Grants and funding
LinkOut - more resources
Full Text Sources
Other Literature Sources
Molecular Biology Databases
Miscellaneous