Cell-of-origin chromatin organization shapes the mutational landscape of cancer
- PMID: 25693567
- PMCID: PMC4405175
- DOI: 10.1038/nature14221
Cell-of-origin chromatin organization shapes the mutational landscape of cancer
Abstract
Cancer is a disease potentiated by mutations in somatic cells. Cancer mutations are not distributed uniformly along the human genome. Instead, different human genomic regions vary by up to fivefold in the local density of cancer somatic mutations, posing a fundamental problem for statistical methods used in cancer genomics. Epigenomic organization has been proposed as a major determinant of the cancer mutational landscape. However, both somatic mutagenesis and epigenomic features are highly cell-type-specific. We investigated the distribution of mutations in multiple independent samples of diverse cancer types and compared them to cell-type-specific epigenomic features. Here we show that chromatin accessibility and modification, together with replication timing, explain up to 86% of the variance in mutation rates along cancer genomes. The best predictors of local somatic mutation density are epigenomic features derived from the most likely cell type of origin of the corresponding malignancy. Moreover, we find that cell-of-origin chromatin features are much stronger determinants of cancer mutation profiles than chromatin features of matched cancer cell lines. Furthermore, we show that the cell type of origin of a cancer can be accurately determined based on the distribution of mutations along its genome. Thus, the DNA sequence of a cancer genome encompasses a wealth of information about the identity and epigenomic features of its cell of origin.
Figures
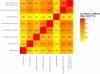
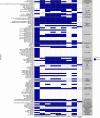
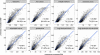
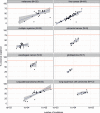
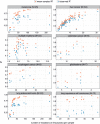
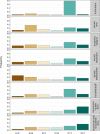
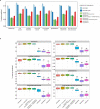
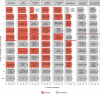
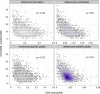
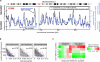
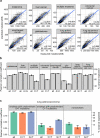
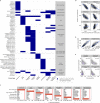
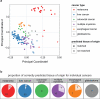
Comment in
-
Epigenetics: Chromatin marks the spot.Nat Rev Cancer. 2015 Apr;15(4):196-7. doi: 10.1038/nrc3934. Epub 2015 Mar 19. Nat Rev Cancer. 2015. PMID: 25786694 No abstract available.
Similar articles
-
Mutation Signatures Depend on Epigenomic Contexts.Trends Cancer. 2018 Oct;4(10):659-661. doi: 10.1016/j.trecan.2018.08.001. Epub 2018 Sep 6. Trends Cancer. 2018. PMID: 30292349 Review.
-
Mutalisk: a web-based somatic MUTation AnaLyIS toolKit for genomic, transcriptional and epigenomic signatures.Nucleic Acids Res. 2018 Jul 2;46(W1):W102-W108. doi: 10.1093/nar/gky406. Nucleic Acids Res. 2018. PMID: 29790943 Free PMC article.
-
Epigenomics: Roadmap for regulation.Nature. 2015 Feb 19;518(7539):314-6. doi: 10.1038/518314a. Nature. 2015. PMID: 25693562 No abstract available.
-
Explaining cancer type specific mutations with transcriptomic and epigenomic features in normal tissues.Sci Rep. 2018 Jul 30;8(1):11456. doi: 10.1038/s41598-018-29861-1. Sci Rep. 2018. PMID: 30061703 Free PMC article.
-
Epigenomic Consequences of Coding and Noncoding Driver Mutations.Trends Cancer. 2016 Oct;2(10):585-605. doi: 10.1016/j.trecan.2016.09.002. Epub 2016 Sep 30. Trends Cancer. 2016. PMID: 28741489 Review.
Cited by
-
Comprehensive analysis of cancer breakpoints reveals signatures of genetic and epigenetic contribution to cancer genome rearrangements.PLoS Comput Biol. 2021 Mar 1;17(3):e1008749. doi: 10.1371/journal.pcbi.1008749. eCollection 2021 Mar. PLoS Comput Biol. 2021. PMID: 33647036 Free PMC article.
-
Epigenetic perturbations in aging stem cells.Mamm Genome. 2016 Aug;27(7-8):396-406. doi: 10.1007/s00335-016-9645-8. Epub 2016 May 26. Mamm Genome. 2016. PMID: 27229519 Free PMC article. Review.
-
MutSpot: detection of non-coding mutation hotspots in cancer genomes.NPJ Genom Med. 2020 Jun 5;5:26. doi: 10.1038/s41525-020-0133-4. eCollection 2020. NPJ Genom Med. 2020. PMID: 32550006 Free PMC article.
-
Nucleotide diversity analysis highlights functionally important genomic regions.Sci Rep. 2016 Oct 24;6:35730. doi: 10.1038/srep35730. Sci Rep. 2016. PMID: 27774999 Free PMC article.
-
Nucleotide excision repair is impaired by binding of transcription factors to DNA.Nature. 2016 Apr 14;532(7598):264-7. doi: 10.1038/nature17661. Nature. 2016. PMID: 27075101
References
-
- Hodgkinson A, Chen Y, Eyre-Walker A. The large-scale distribution of somatic mutations in cancer genomes. Human mutation. 2012;33:136–143. doi:10.1002/humu.21616. - PubMed
-
- Schuster-Bockler B, Lehner B. Chromatin organization is a major influence on regional mutation rates in human cancer cells. Nature. 2012;488:504–507. doi:10.1038/nature11273. - PubMed
-
- Woo YH, Li WH. DNA replication timing and selection shape the landscape of nucleotide variation in cancer genomes. Nature communications. 2012;3:1004. doi:10.1038/ncomms1982. - PubMed
Publication types
MeSH terms
Substances
Associated data
- Actions
Grants and funding
LinkOut - more resources
Full Text Sources
Other Literature Sources