A multi-scale approach reveals that NF-κB cRel enforces a B-cell decision to divide
- PMID: 25680807
- PMCID: PMC4358656
- DOI: 10.15252/msb.20145554
A multi-scale approach reveals that NF-κB cRel enforces a B-cell decision to divide
Abstract
Understanding the functions of multi-cellular organs in terms of the molecular networks within each cell is an important step in the quest to predict phenotype from genotype. B-lymphocyte population dynamics, which are predictive of immune response and vaccine effectiveness, are determined by individual cells undergoing division or death seemingly stochastically. Based on tracking single-cell time-lapse trajectories of hundreds of B cells, single-cell transcriptome, and immunofluorescence analyses, we constructed an agent-based multi-modular computational model to simulate lymphocyte population dynamics in terms of the molecular networks that control NF-κB signaling, the cell cycle, and apoptosis. Combining modeling and experimentation, we found that NF-κB cRel enforces the execution of a cellular decision between mutually exclusive fates by promoting survival in growing cells. But as cRel deficiency causes growing B cells to die at similar rates to non-growing cells, our analysis reveals that the phenomenological decision model of wild-type cells is rooted in a biased race of cell fates. We show that a multi-scale modeling approach allows for the prediction of dynamic organ-level physiology in terms of intra-cellular molecular networks.
Keywords: B‐lymphocyte; NF‐κB cRel; apoptosis; cell cycle; cell fate decision.
© 2015 The Authors. Published under the terms of the CC BY 4.0 license.
Figures
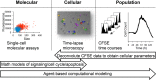
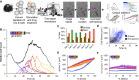
A Overview of the time-lapse microscopy experimental and analysis pipeline. B cells were purified from mouse spleen, stimulated with TLR9 agonist CpG, imaged on an environmentally controlled microscope for 6 days, and tracked using a semi-automated tracking tool to quantify generation-dependent cell statistics.
B Generational cell counts relative to initial count.
C The observed fraction of cells dividing or dying in each generation. Error bars = 1/n, where n = 85, 55, 93, 103, 125, 90, 45 for generations 0–6, respectively.
D Growth trajectories of generation 0 cells that grew by more than 350 μm3 or ended with a volume of at least 800 μm3 (blue) and trajectories of generation 0 cells that did not end with a large volume (black).
E, F Cell size trajectories as a function of % lifetime for growers (E) and non-growers (F) in each generation (colors as in B). Error bars are SD, with n = 34, 44, 60, 54, 40, 15, 2 growing cells, 51, 11, 33, 49, 85, 75, 43 non-growing cells in generations 0–6, respectively.
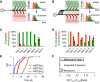
A Flowchart depicting the scenario in which the cell fate of growing cells is determined by a race between division (green) and death (red); hypothetical division and death time distributions before and after mutual censorship are shown on the right.
B Flowchart depicting the scenario in which entering the growth phase or not represents an early commitment to one fate; the independent division (green) and death (red) time distributions are shown on the right.
C–F Analysis of response (growth), division, and death statistics for WT B cells. Error bars = 1/n. Measured generational probabilities that a growing (C) or non-growing (D) cell divided (green) or died (red) within a 24-h period (12–36 h for gen 0). Measured cumulative distributions (E) for the time to start growing (blue), time to divide (green), and time to die (red) of generation 0 cells. Distributions were used to calculate the lower bound expected probability that a dying cell had started to grow (F) assuming a molecular race between fates or an early commitment to one fate, as compared to the measured probability. For further details, please see Supplementary Methods.
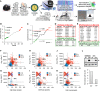
A–L Naïve purified B cells were stimulated with 250 nM CpG for 24 h and analyzed using single-cell RNA sequencing (A top). Five small and five large B cells were captured in a microfluidics chip (B), and their transcriptomes were sequenced to reveal sets of genes typically upregulated in big cells (C, red) or small cells (C, green). Pathway analysis on genes upregulated in large B cells (D, top) and small cells (D, bottom) was performed. (E) Transcription factor motif enrichment analysis on the genes upregulated in large cells (E, top) and small cells (E, bottom) was performed and filtered to show only significantly upregulated (P < 0.05) and known NF-κB target genes or NF-κB itself. NF-κB cRel abundances of purified naïve B cells stimulated with 250 nM CpG for 24 h were obtained by quantifying average fluorescence in fixed B cells stained with anti-cRel antibody conjugated to fluorophore, or anti-BclXL antibody bound to a fluorescent secondary antibody (A bottom). The 0-h average fluorescence was used to determine significant upregulation of NF-κB cRel (F), growth regulator Myc (H), and anti-apoptotic regulator BclXL (J) at 24 h (P < 0.05). Immunoblot for p-S6 (arrow), a downstream target of mTORc1, with anti-tubulin control after 24 h CpG stimulation in WT and cRel-deficient B cells and gel quantification is shown (L). NF-κB cRel-deficient cells were used to approximate the technical noise (G) or dependence of Myc (I), BclXL (K), and mTORc1 (L) on NF-κB cRel. Quadrants in (F–K) indicate fraction of cells at 24 h compared to 0 h. Growth was manually defined as a cell area > 100 pixels to avoid cell selection bias in images.
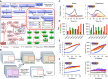
A Established ordinary differential equation models for NF-κB signaling (Alves et al, 2014), apoptosis (Loriaux & Hoffmann, 2012), and the cell cycle (Conradie et al, 2010) were implemented and combined into one integrated model. Blue, green, and red colors represent NF-κB, apoptosis, and cell-cycle modules, respectively, while bolded species represent active IKK (input), cleaved PARP (death readout), and cdh1 abundance (mitosis readout).
B Instances of the integrated model were incorporated into cellular agents, extrinsic noise was introduced to mimic cell-to-cell variability, and the agent-based model was solved one generation at a time, with division resulting in the creation of two new agents, and death resulting in the removal of the agent from the population.
C–F A comparison of agent-based modeling solutions to the time-lapse microscopy dataset is shown. Cell counts normalized to start count (C), fraction of cells dividing or dying in each generation (D), average size of growers in each generation as a function of % lifetime (E), and average size of non-growers in each generation as a function of % lifetime (F) are compared. Error bars represent SEM or 1/n.
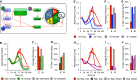
A After parameterizing the multi-scale model using results from wild-type B cells stimulated with 250 nM CpG (red), we predicted the effects of decreasing IKK duration (green), lack of NF-κB cRel (blue), and decreased protein synthesis (purple) in silico and compared the results to those from analogous time-lapse microscopy experiments where we stimulated with only 10 nM CpG, used cRel deficient cells, or pretreated with 1 ng/ml rapamycin.
B–J Side-by-side comparison of modeling and experimental results: total cell counts (B, E, H), average number of divisions (C, F, I), and fraction of growing progenitors that died (D, G, J).
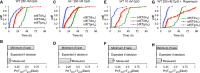
A–H Time-lapse microscopy images of wild-type B cells stimulated with 250 nM CpG (A, B), NF-κB cRel-deficient B cells stimulated with 250 nM CpG (C, D), wild-type cells stimulated with 10 nM CpG (E, F), and wild-type B cells stimulated with 250 nM CpG and pretreated with 1 ng/ml rapamycin for 1 h (G, H) were tracked. The observed cumulative distributions (A, C, E, G) for time to start growing (Tgro), time to divide (Tdiv), and time to die (Tdie) were used to estimate the minimum probability of observing grown cells that die in generation 0 assuming that division and death were occurring simultaneously (molecular race), and compared to the actual sampled probabilities for each condition (B, D, F, H).
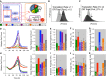
A–K The cell-to-cell distribution of total IKK (green), NF-κB cRel/NF-κB p50 (blue), both NF-κB C,50 and total IKK (purple), non-NF-κB proteins (orange), or all proteins (gray) was varied (A). Specifically, the protein production (B, D–G) was increased (Total IKK mean ×1.5, cRel/p50 induction ×1.5, or protein production ×1.5), or the coefficient of variation (C, H–K) was doubled, and the population dynamics and maximum relative cell count (D, H), mean number of times a progenitor is expected to divide given the observed fraction of dividers in each generation (E, I), average generation 1,2,… division times (F, J), and the number of in silico cell surviving at the end of the simulation (G, K) were compared. Error bars = SD. No error bars are shown for D, E, G, H, I, and K as they represent one global feature for each simulation.
Similar articles
-
IκBε is a key regulator of B cell expansion by providing negative feedback on cRel and RelA in a stimulus-specific manner.J Immunol. 2014 Apr 1;192(7):3121-32. doi: 10.4049/jimmunol.1302351. Epub 2014 Mar 3. J Immunol. 2014. PMID: 24591377 Free PMC article.
-
Combined deficiency of p50 and cRel in CD4+ T cells reveals an essential requirement for nuclear factor kappaB in regulating mature T cell survival and in vivo function.J Exp Med. 2003 Apr 7;197(7):861-74. doi: 10.1084/jem.20021610. Epub 2003 Mar 31. J Exp Med. 2003. PMID: 12668645 Free PMC article.
-
Critical role of cRel subunit of NF-κB in sepsis survival.Infect Immun. 2011 May;79(5):1848-54. doi: 10.1128/IAI.00021-11. Epub 2011 Feb 22. Infect Immun. 2011. PMID: 21343350 Free PMC article.
-
NF-kappaB signaling in lymphocytes: a new cast of characters.J Cell Sci. 2004 Jan 1;117(Pt 1):31-9. doi: 10.1242/jcs.00904. J Cell Sci. 2004. PMID: 14657271 Review.
-
[The Rel/NF-kappa B transcription factors and their biological functions].Cas Lek Cesk. 2001 Mar 29;140(6):163-7. Cas Lek Cesk. 2001. PMID: 11347205 Review. Czech.
Cited by
-
The NFκB signaling system in the generation of B-cell subsets: from germinal center B cells to memory B cells and plasma cells.Front Immunol. 2023 Dec 11;14:1185597. doi: 10.3389/fimmu.2023.1185597. eCollection 2023. Front Immunol. 2023. PMID: 38169968 Free PMC article. Review.
-
Cyton2: A Model of Immune Cell Population Dynamics That Includes Familial Instructional Inheritance.Front Bioinform. 2021 Oct 26;1:723337. doi: 10.3389/fbinf.2021.723337. eCollection 2021. Front Bioinform. 2021. PMID: 36303793 Free PMC article.
-
Image data in need of a home.Mol Syst Biol. 2015 Dec 23;11(12):853. doi: 10.15252/msb.20156719. Mol Syst Biol. 2015. PMID: 26700853 Free PMC article.
-
Protein abundance of AKT and ERK pathway components governs cell type-specific regulation of proliferation.Mol Syst Biol. 2017 Jan 24;13(1):904. doi: 10.15252/msb.20167258. Mol Syst Biol. 2017. PMID: 28123004 Free PMC article.
-
Patient-specific computational models predict prognosis in B cell lymphoma by quantifying pro-proliferative and anti-apoptotic signatures from genetic sequencing data.Blood Cancer J. 2024 Jul 4;14(1):105. doi: 10.1038/s41408-024-01090-y. Blood Cancer J. 2024. PMID: 38965209 Free PMC article.
References
Publication types
MeSH terms
Substances
Associated data
- Actions
Grants and funding
LinkOut - more resources
Full Text Sources
Other Literature Sources
Molecular Biology Databases