Protein deimmunization via structure-based design enables efficient epitope deletion at high mutational loads
- PMID: 25655032
- PMCID: PMC4452428
- DOI: 10.1002/bit.25554
Protein deimmunization via structure-based design enables efficient epitope deletion at high mutational loads
Abstract
Anti-drug immune responses are a unique risk factor for biotherapeutics, and undesired immunogenicity can alter pharmacokinetics, compromise drug efficacy, and in some cases even threaten patient safety. To fully capitalize on the promise of biotherapeutics, more efficient and generally applicable protein deimmunization tools are needed. Mutagenic deletion of a protein's T cell epitopes is one powerful strategy to engineer immunotolerance, but deimmunizing mutations must maintain protein structure and function. Here, EpiSweep, a structure-based protein design and deimmunization algorithm, has been used to produce a panel of seven beta-lactamase drug candidates having 27-47% reductions in predicted epitope content. Despite bearing eight mutations each, all seven engineered enzymes maintained good stability and activity. At the same time, the variants exhibited dramatically reduced interaction with human class II major histocompatibility complex proteins, key regulators of anti-drug immune responses. When compared to 8-mutation designs generated with a sequence-based deimmunization algorithm, the structure-based designs retained greater thermostability and possessed fewer high affinity epitopes, the dominant drivers of anti-biotherapeutic immune responses. These experimental results validate the first structure-based deimmunization algorithm capable of mapping optimal biotherapeutic design space. By designing optimal mutations that reduce immunogenic potential while imparting favorable intramolecular interactions, broadly distributed epitopes may be simultaneously targeted using high mutational loads.
Keywords: T cell epitope deletion; biotherapeutics; computational protein design; deimmunization; immunogenicity.
© 2015 Wiley Periodicals, Inc.
Conflict of interest statement
Karl E. Griswold and Chris Bailey-Kellogg are Dartmouth faculty and co-members of Stealth Biologics, LLC, a Delaware biotechnology company. They acknowledge that there is a potential conflict of interest related to their association with this company, and they hereby affirm that the data presented in this paper is free of any bias. This work has been reviewed and approved as specified in these faculty members’ Dartmouth conflict of interest management plans. The remaining authors declare no conflict of interest.
Figures
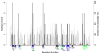
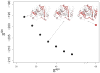
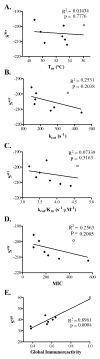
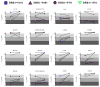
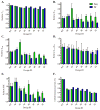
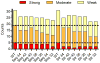
Similar articles
-
Computationally driven deletion of broadly distributed T cell epitopes in a biotherapeutic candidate.Cell Mol Life Sci. 2014 Dec;71(24):4869-80. doi: 10.1007/s00018-014-1652-x. Epub 2014 Jun 1. Cell Mol Life Sci. 2014. PMID: 24880662 Free PMC article.
-
EpiSweep: Computationally Driven Reengineering of Therapeutic Proteins to Reduce Immunogenicity While Maintaining Function.Methods Mol Biol. 2017;1529:375-398. doi: 10.1007/978-1-4939-6637-0_20. Methods Mol Biol. 2017. PMID: 27914063 Free PMC article.
-
Structure-guided deimmunization of therapeutic proteins.J Comput Biol. 2013 Feb;20(2):152-65. doi: 10.1089/cmb.2012.0251. J Comput Biol. 2013. PMID: 23384000 Free PMC article.
-
Deimmunization of protein therapeutics - Recent advances in experimental and computational epitope prediction and deletion.Comput Struct Biotechnol J. 2020 Dec 29;19:315-329. doi: 10.1016/j.csbj.2020.12.024. eCollection 2021. Comput Struct Biotechnol J. 2020. PMID: 33425259 Free PMC article. Review.
-
Applying MAPPs Assays to Assess Drug Immunogenicity.Front Immunol. 2020 Apr 21;11:698. doi: 10.3389/fimmu.2020.00698. eCollection 2020. Front Immunol. 2020. PMID: 32373128 Free PMC article. Review.
Cited by
-
Pareto Optimization of Combinatorial Mutagenesis Libraries.IEEE/ACM Trans Comput Biol Bioinform. 2019 Jul-Aug;16(4):1143-1153. doi: 10.1109/TCBB.2018.2858794. Epub 2018 Jul 23. IEEE/ACM Trans Comput Biol Bioinform. 2019. PMID: 30040654 Free PMC article.
-
Elimination of murine and human T-cell epitopes in recombinant immunotoxin eliminates neutralizing and anti-drug antibodies in vivo.Cell Mol Immunol. 2017 May;14(5):432-442. doi: 10.1038/cmi.2015.91. Epub 2015 Oct 19. Cell Mol Immunol. 2017. PMID: 26477977 Free PMC article.
-
OSPREY Predicts Resistance Mutations Using Positive and Negative Computational Protein Design.Methods Mol Biol. 2017;1529:291-306. doi: 10.1007/978-1-4939-6637-0_15. Methods Mol Biol. 2017. PMID: 27914058 Free PMC article.
-
Design and engineering of deimmunized biotherapeutics.Curr Opin Struct Biol. 2016 Aug;39:79-88. doi: 10.1016/j.sbi.2016.06.003. Epub 2016 Jun 17. Curr Opin Struct Biol. 2016. PMID: 27322891 Free PMC article. Review.
-
Computationally-driven identification of antibody epitopes.Elife. 2017 Dec 4;6:e29023. doi: 10.7554/eLife.29023. Elife. 2017. PMID: 29199956 Free PMC article.
References
-
- Aggarwal S. What’s fueling the biotech engine - 2012 to 2013. Nat Biotech. 2014;32(1):32–39. - PubMed
-
- Barbosa MD. Immunogenicity of biotherapeutics in the context of developing biosimilars and biobetters. Drug Discov Today. 2011;16(7–8):345–53. - PubMed
-
- Bryson CJ, Jones TD, Baker MP. Prediction of immunogenicity of therapeutic proteins: validity of computational tools. BioDrugs. 2010;24(1):1–8. - PubMed
Publication types
MeSH terms
Substances
Grants and funding
LinkOut - more resources
Full Text Sources
Other Literature Sources
Molecular Biology Databases