PON-P2: prediction method for fast and reliable identification of harmful variants
- PMID: 25647319
- PMCID: PMC4315405
- DOI: 10.1371/journal.pone.0117380
PON-P2: prediction method for fast and reliable identification of harmful variants
Abstract
More reliable and faster prediction methods are needed to interpret enormous amounts of data generated by sequencing and genome projects. We have developed a new computational tool, PON-P2, for classification of amino acid substitutions in human proteins. The method is a machine learning-based classifier and groups the variants into pathogenic, neutral and unknown classes, on the basis of random forest probability score. PON-P2 is trained using pathogenic and neutral variants obtained from VariBench, a database for benchmark variation datasets. PON-P2 utilizes information about evolutionary conservation of sequences, physical and biochemical properties of amino acids, GO annotations and if available, functional annotations of variation sites. Extensive feature selection was performed to identify 8 informative features among altogether 622 features. PON-P2 consistently showed superior performance in comparison to existing state-of-the-art tools. In 10-fold cross-validation test, its accuracy and MCC are 0.90 and 0.80, respectively, and in the independent test, they are 0.86 and 0.71, respectively. The coverage of PON-P2 is 61.7% in the 10-fold cross-validation and 62.1% in the test dataset. PON-P2 is a powerful tool for screening harmful variants and for ranking and prioritizing experimental characterization. It is very fast making it capable of analyzing large variant datasets. PON-P2 is freely available at http://structure.bmc.lu.se/PON-P2/.
Conflict of interest statement
Figures
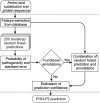
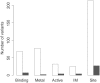
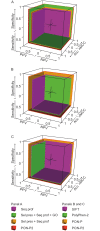
Similar articles
-
Classification of Amino Acid Substitutions in Mismatch Repair Proteins Using PON-MMR2.Hum Mutat. 2015 Dec;36(12):1128-34. doi: 10.1002/humu.22900. Epub 2015 Sep 22. Hum Mutat. 2015. PMID: 26333163
-
PON-Sol: prediction of effects of amino acid substitutions on protein solubility.Bioinformatics. 2016 Jul 1;32(13):2032-4. doi: 10.1093/bioinformatics/btw066. Epub 2016 Feb 19. Bioinformatics. 2016. PMID: 27153720
-
PON-All: Amino Acid Substitution Tolerance Predictor for All Organisms.Front Mol Biosci. 2022 Jun 16;9:867572. doi: 10.3389/fmolb.2022.867572. eCollection 2022. Front Mol Biosci. 2022. PMID: 35782867 Free PMC article.
-
Molecular dynamics simulations for genetic interpretation in protein coding regions: where we are, where to go and when.Brief Bioinform. 2021 Jan 18;22(1):3-19. doi: 10.1093/bib/bbz146. Brief Bioinform. 2021. PMID: 31813950 Review.
-
Evolution of a comprehensive, orthogonal approach to sequence variant analysis for biotherapeutics.MAbs. 2019 Jan;11(1):1-12. doi: 10.1080/19420862.2018.1531965. Epub 2018 Oct 25. MAbs. 2019. PMID: 30303443 Free PMC article. Review.
Cited by
-
RheoScale: A tool to aggregate and quantify experimentally determined substitution outcomes for multiple variants at individual protein positions.Hum Mutat. 2018 Dec;39(12):1814-1826. doi: 10.1002/humu.23616. Epub 2018 Aug 28. Hum Mutat. 2018. PMID: 30117637 Free PMC article.
-
Impact of Deleterious Mutations on Structure, Function and Stability of Serum/Glucocorticoid Regulated Kinase 1: A Gene to Diseases Correlation.Front Mol Biosci. 2021 Nov 3;8:780284. doi: 10.3389/fmolb.2021.780284. eCollection 2021. Front Mol Biosci. 2021. PMID: 34805284 Free PMC article.
-
PON-tstab: Protein Variant Stability Predictor. Importance of Training Data Quality.Int J Mol Sci. 2018 Mar 28;19(4):1009. doi: 10.3390/ijms19041009. Int J Mol Sci. 2018. PMID: 29597263 Free PMC article.
-
PMut: a web-based tool for the annotation of pathological variants on proteins, 2017 update.Nucleic Acids Res. 2017 Jul 3;45(W1):W222-W228. doi: 10.1093/nar/gkx313. Nucleic Acids Res. 2017. PMID: 28453649 Free PMC article.
-
PON-SC - program for identifying steric clashes caused by amino acid substitutions.BMC Bioinformatics. 2017 Nov 29;18(1):531. doi: 10.1186/s12859-017-1947-7. BMC Bioinformatics. 2017. PMID: 29187139 Free PMC article.
References
Publication types
MeSH terms
Substances
Grants and funding
LinkOut - more resources
Full Text Sources
Other Literature Sources
Miscellaneous