Aberrant gene expression in humans
- PMID: 25617623
- PMCID: PMC4305293
- DOI: 10.1371/journal.pgen.1004942
Aberrant gene expression in humans
Abstract
Gene expression as an intermediate molecular phenotype has been a focus of research interest. In particular, studies of expression quantitative trait loci (eQTL) have offered promise for understanding gene regulation through the discovery of genetic variants that explain variation in gene expression levels. Existing eQTL methods are designed for assessing the effects of common variants, but not rare variants. Here, we address the problem by establishing a novel analytical framework for evaluating the effects of rare or private variants on gene expression. Our method starts from the identification of outlier individuals that show markedly different gene expression from the majority of a population, and then reveals the contributions of private SNPs to the aberrant gene expression in these outliers. Using population-scale mRNA sequencing data, we identify outlier individuals using a multivariate approach. We find that outlier individuals are more readily detected with respect to gene sets that include genes involved in cellular regulation and signal transduction, and less likely to be detected with respect to the gene sets with genes involved in metabolic pathways and other fundamental molecular functions. Analysis of polymorphic data suggests that private SNPs of outlier individuals are enriched in the enhancer and promoter regions of corresponding aberrantly-expressed genes, suggesting a specific regulatory role of private SNPs, while the commonly-occurring regulatory genetic variants (i.e., eQTL SNPs) show little evidence of involvement. Additional data suggest that non-genetic factors may also underlie aberrant gene expression. Taken together, our findings advance a novel viewpoint relevant to situations wherein common eQTLs fail to predict gene expression when heritable, rare inter-individual variation exists. The analytical framework we describe, taking into consideration the reality of differential phenotypic robustness, may be valuable for investigating complex traits and conditions.
Conflict of interest statement
The authors have declared that no competing interests exist.
Figures
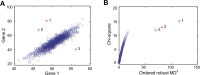
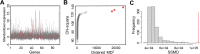
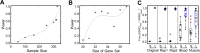
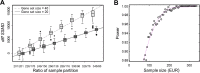
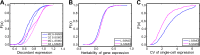
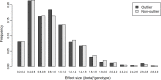
Similar articles
-
Trans-eQTLs reveal that independent genetic variants associated with a complex phenotype converge on intermediate genes, with a major role for the HLA.PLoS Genet. 2011 Aug;7(8):e1002197. doi: 10.1371/journal.pgen.1002197. Epub 2011 Aug 4. PLoS Genet. 2011. PMID: 21829388 Free PMC article.
-
Cis-regulatory variations: a study of SNPs around genes showing cis-linkage in segregating mouse populations.BMC Genomics. 2006 Sep 15;7:235. doi: 10.1186/1471-2164-7-235. BMC Genomics. 2006. PMID: 16978413 Free PMC article.
-
Population- and individual-specific regulatory variation in Sardinia.Nat Genet. 2017 May;49(5):700-707. doi: 10.1038/ng.3840. Epub 2017 Apr 10. Nat Genet. 2017. PMID: 28394350 Free PMC article.
-
Expression Quantitative Trait Loci Information Improves Predictive Modeling of Disease Relevance of Non-Coding Genetic Variation.PLoS One. 2015 Oct 16;10(10):e0140758. doi: 10.1371/journal.pone.0140758. eCollection 2015. PLoS One. 2015. PMID: 26474488 Free PMC article. Review.
-
Expression quantitative trait loci: present and future.Philos Trans R Soc Lond B Biol Sci. 2013 May 6;368(1620):20120362. doi: 10.1098/rstb.2012.0362. Print 2013. Philos Trans R Soc Lond B Biol Sci. 2013. PMID: 23650636 Free PMC article. Review.
Cited by
-
Improving data interpretability with new differential sample variance gene set tests.Res Sq [Preprint]. 2024 Sep 9:rs.3.rs-4888767. doi: 10.21203/rs.3.rs-4888767/v1. Res Sq. 2024. PMID: 39315246 Free PMC article. Preprint.
-
ORE identifies extreme expression effects enriched for rare variants.Bioinformatics. 2019 Oct 15;35(20):3906-3912. doi: 10.1093/bioinformatics/btz202. Bioinformatics. 2019. PMID: 30903145 Free PMC article.
-
RegVar: Tissue-specific Prioritization of Non-coding Regulatory Variants.Genomics Proteomics Bioinformatics. 2023 Apr;21(2):385-395. doi: 10.1016/j.gpb.2021.08.011. Epub 2021 Dec 29. Genomics Proteomics Bioinformatics. 2023. PMID: 34973416 Free PMC article.
-
Exploring the RNA Gap for Improving Diagnostic Yield in Primary Immunodeficiencies.Front Genet. 2019 Dec 11;10:1204. doi: 10.3389/fgene.2019.01204. eCollection 2019. Front Genet. 2019. PMID: 31921280 Free PMC article. Review.
-
A Burden of Rare Variants Associated with Extremes of Gene Expression in Human Peripheral Blood.Am J Hum Genet. 2016 Feb 4;98(2):299-309. doi: 10.1016/j.ajhg.2015.12.023. Am J Hum Genet. 2016. PMID: 26849112 Free PMC article.
References
Publication types
MeSH terms
Grants and funding
LinkOut - more resources
Full Text Sources
Other Literature Sources