Integrated genome and transcriptome sequencing of the same cell
- PMID: 25599178
- PMCID: PMC4374170
- DOI: 10.1038/nbt.3129
Integrated genome and transcriptome sequencing of the same cell
Abstract
Single-cell genomics and single-cell transcriptomics have emerged as powerful tools to study the biology of single cells at a genome-wide scale. However, a major challenge is to sequence both genomic DNA and mRNA from the same cell, which would allow direct comparison of genomic variation and transcriptome heterogeneity. We describe a quasilinear amplification strategy to quantify genomic DNA and mRNA from the same cell without physically separating the nucleic acids before amplification. We show that the efficiency of our integrated approach is similar to existing methods for single-cell sequencing of either genomic DNA or mRNA. Further, we find that genes with high cell-to-cell variability in transcript numbers generally have lower genomic copy numbers, and vice versa, suggesting that copy number variations may drive variability in gene expression among individual cells. Applications of our integrated sequencing approach could range from gaining insights into cancer evolution and heterogeneity to understanding the transcriptional consequences of copy number variations in healthy and diseased tissues.
Figures
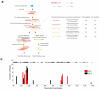
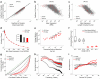
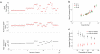
Similar articles
-
Combined Genome and Transcriptome (G&T) Sequencing of Single Cells.Methods Mol Biol. 2019;1979:319-362. doi: 10.1007/978-1-4939-9240-9_20. Methods Mol Biol. 2019. PMID: 31028647
-
Chromosomal Instability Estimation Based on Next Generation Sequencing and Single Cell Genome Wide Copy Number Variation Analysis.PLoS One. 2016 Nov 16;11(11):e0165089. doi: 10.1371/journal.pone.0165089. eCollection 2016. PLoS One. 2016. PMID: 27851748 Free PMC article.
-
Fungal Transcriptomics.Methods Mol Biol. 2018;1775:83-92. doi: 10.1007/978-1-4939-7804-5_8. Methods Mol Biol. 2018. PMID: 29876811
-
Current and Future Methods for mRNA Analysis: A Drive Toward Single Molecule Sequencing.Methods Mol Biol. 2018;1783:209-241. doi: 10.1007/978-1-4939-7834-2_11. Methods Mol Biol. 2018. PMID: 29767365 Review.
-
Single-cell sequencing technologies: current and future.J Genet Genomics. 2014 Oct 20;41(10):513-28. doi: 10.1016/j.jgg.2014.09.005. Epub 2014 Oct 18. J Genet Genomics. 2014. PMID: 25438696 Review.
Cited by
-
Chromatin tracing and multiplexed imaging of nucleome architectures (MINA) and RNAs in single mammalian cells and tissue.Nat Protoc. 2021 May;16(5):2667-2697. doi: 10.1038/s41596-021-00518-0. Epub 2021 Apr 26. Nat Protoc. 2021. PMID: 33903756 Free PMC article.
-
Failure of Glial Cell-Line Derived Neurotrophic Factor (GDNF) in Clinical Trials Orchestrated By Reduced NR4A2 (NURR1) Transcription Factor in Parkinson's Disease. A Systematic Review.Front Aging Neurosci. 2021 Feb 24;13:645583. doi: 10.3389/fnagi.2021.645583. eCollection 2021. Front Aging Neurosci. 2021. PMID: 33716718 Free PMC article.
-
Deciphering Cell Fate Decision by Integrated Single-Cell Sequencing Analysis.Annu Rev Biomed Data Sci. 2020 Jul;3:1-22. doi: 10.1146/annurev-biodatasci-111419-091750. Epub 2020 Mar 2. Annu Rev Biomed Data Sci. 2020. PMID: 32780577 Free PMC article.
-
Exploring long non-coding RNA networks from single cell omics data.Comput Struct Biotechnol J. 2022 Aug 4;20:4381-4389. doi: 10.1016/j.csbj.2022.08.003. eCollection 2022. Comput Struct Biotechnol J. 2022. PMID: 36051880 Free PMC article. Review.
-
Identifying cancer cells from calling single-nucleotide variants in scRNA-seq data.Bioinformatics. 2024 Sep 2;40(9):btae512. doi: 10.1093/bioinformatics/btae512. Bioinformatics. 2024. PMID: 39163479 Free PMC article.
References
Publication types
MeSH terms
Substances
Associated data
- Actions
Grants and funding
LinkOut - more resources
Full Text Sources
Other Literature Sources
Molecular Biology Databases