Evolution, energy landscapes and the paradoxes of protein folding
- PMID: 25530262
- PMCID: PMC4472606
- DOI: 10.1016/j.biochi.2014.12.007
Evolution, energy landscapes and the paradoxes of protein folding
Abstract
Protein folding has been viewed as a difficult problem of molecular self-organization. The search problem involved in folding however has been simplified through the evolution of folding energy landscapes that are funneled. The funnel hypothesis can be quantified using energy landscape theory based on the minimal frustration principle. Strong quantitative predictions that follow from energy landscape theory have been widely confirmed both through laboratory folding experiments and from detailed simulations. Energy landscape ideas also have allowed successful protein structure prediction algorithms to be developed. The selection constraint of having funneled folding landscapes has left its imprint on the sequences of existing protein structural families. Quantitative analysis of co-evolution patterns allows us to infer the statistical characteristics of the folding landscape. These turn out to be consistent with what has been obtained from laboratory physicochemical folding experiments signaling a beautiful confluence of genomics and chemical physics.
Keywords: Folding landscape; Natural selection; Structure prediction.
Copyright © 2014 Elsevier B.V. and Société Française de Biochimie et Biologie Moléculaire (SFBBM). All rights reserved.
Figures
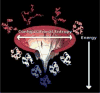
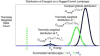
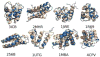
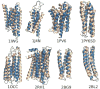
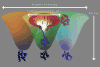
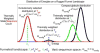
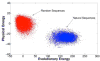
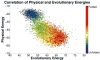
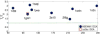
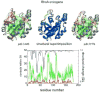
Similar articles
-
Coevolutionary information, protein folding landscapes, and the thermodynamics of natural selection.Proc Natl Acad Sci U S A. 2014 Aug 26;111(34):12408-13. doi: 10.1073/pnas.1413575111. Epub 2014 Aug 11. Proc Natl Acad Sci U S A. 2014. PMID: 25114242 Free PMC article.
-
Recent successes of the energy landscape theory of protein folding and function.Q Rev Biophys. 2005 Nov;38(4):405-10. doi: 10.1017/S0033583505004075. Q Rev Biophys. 2005. PMID: 16934172 Review.
-
Buffed energy landscapes: another solution to the kinetic paradoxes of protein folding.Proc Natl Acad Sci U S A. 2003 Apr 15;100(8):4417-22. doi: 10.1073/pnas.0330720100. Epub 2003 Apr 3. Proc Natl Acad Sci U S A. 2003. PMID: 12677002 Free PMC article.
-
The folding energy landscape and free energy excitations of cytochrome c.Acc Chem Res. 2010 May 18;43(5):652-60. doi: 10.1021/ar9002703. Acc Chem Res. 2010. PMID: 20143816 Free PMC article. Review.
-
Folding funnels: the key to robust protein structure prediction.J Comput Chem. 2002 Jan 15;23(1):138-46. doi: 10.1002/jcc.1162. J Comput Chem. 2002. PMID: 11913379
Cited by
-
The alterations of repetitive transcranial magnetic stimulation on the energy landscape of resting-state networks differ across the human cortex.Hum Brain Mapp. 2024 Oct 15;45(15):e70029. doi: 10.1002/hbm.70029. Hum Brain Mapp. 2024. PMID: 39465912 Free PMC article.
-
Exploring the aggregation free energy landscape of the amyloid-β protein (1-40).Proc Natl Acad Sci U S A. 2016 Oct 18;113(42):11835-11840. doi: 10.1073/pnas.1612362113. Epub 2016 Oct 3. Proc Natl Acad Sci U S A. 2016. PMID: 27698130 Free PMC article.
-
Effects of nonequilibrium fluctuations on ultrafast short-range electron transfer dynamics.Nat Commun. 2020 Jun 4;11(1):2822. doi: 10.1038/s41467-020-15535-y. Nat Commun. 2020. PMID: 32499536 Free PMC article.
-
De novo prediction of human chromosome structures: Epigenetic marking patterns encode genome architecture.Proc Natl Acad Sci U S A. 2017 Nov 14;114(46):12126-12131. doi: 10.1073/pnas.1714980114. Epub 2017 Oct 31. Proc Natl Acad Sci U S A. 2017. PMID: 29087948 Free PMC article.
-
Energy-dependent protein folding: modeling how a protein folding machine may work.F1000Res. 2021 Jan 5;10:3. doi: 10.12688/f1000research.28175.1. eCollection 2021. F1000Res. 2021. PMID: 33633838 Free PMC article.
References
-
- Wolynes PG. Three Paradoxes of Protein Folding. In: Bohr H, Brunak S, editors. Proceedings on Symposium on Protein Folds: A Distance-Based Approach, Symposium Distance-Based Approaches to Protein Structure Determination II; Copenhagen. November 1994; Boca Raton, FL: CRC Press; 1995. pp. 3–17.
-
- Anfinsen CB. Nobel Lecture. Bethesda, MD: National Institutes Of Health; Dec 11, 1972. Studies On The Principles That Govern The Folding Of Protein Chains. http://www.nobelprize.org/nobel_prizes/chemistry/laureates/1972/anfinsen....
-
- Stent G. That was the molecular biology that was. Science. 1968;160:390–395. - PubMed
-
- Service RF. Problem -Solved* (*Sort of) Science. 2008;321:784–786. - PubMed
Publication types
MeSH terms
Grants and funding
LinkOut - more resources
Full Text Sources
Other Literature Sources