Partitioning heritability of regulatory and cell-type-specific variants across 11 common diseases
- PMID: 25439723
- PMCID: PMC4225595
- DOI: 10.1016/j.ajhg.2014.10.004
Partitioning heritability of regulatory and cell-type-specific variants across 11 common diseases
Abstract
Regulatory and coding variants are known to be enriched with associations identified by genome-wide association studies (GWASs) of complex disease, but their contributions to trait heritability are currently unknown. We applied variance-component methods to imputed genotype data for 11 common diseases to partition the heritability explained by genotyped SNPs (hg(2)) across functional categories (while accounting for shared variance due to linkage disequilibrium). Extensive simulations showed that in contrast to current estimates from GWAS summary statistics, the variance-component approach partitions heritability accurately under a wide range of complex-disease architectures. Across the 11 diseases DNaseI hypersensitivity sites (DHSs) from 217 cell types spanned 16% of imputed SNPs (and 24% of genotyped SNPs) but explained an average of 79% (SE = 8%) of hg(2) from imputed SNPs (5.1× enrichment; p = 3.7 × 10(-17)) and 38% (SE = 4%) of hg(2) from genotyped SNPs (1.6× enrichment, p = 1.0 × 10(-4)). Further enrichment was observed at enhancer DHSs and cell-type-specific DHSs. In contrast, coding variants, which span 1% of the genome, explained <10% of hg(2) despite having the highest enrichment. We replicated these findings but found no significant contribution from rare coding variants in independent schizophrenia cohorts genotyped on GWAS and exome chips. Our results highlight the value of analyzing components of heritability to unravel the functional architecture of common disease.
Copyright © 2014 The American Society of Human Genetics. Published by Elsevier Inc. All rights reserved.
Figures
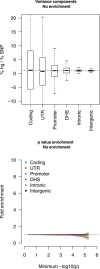
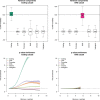
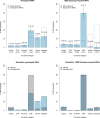
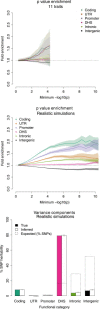
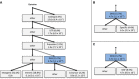
Similar articles
-
Missing heritability of common diseases and treatments outside the protein-coding exome.Hum Genet. 2014 Oct;133(10):1199-215. doi: 10.1007/s00439-014-1476-7. Epub 2014 Aug 9. Hum Genet. 2014. PMID: 25107510 Free PMC article. Review.
-
Evaluation of heritability partitioning approaches in livestock populations.BMC Genomics. 2024 Jul 13;25(1):690. doi: 10.1186/s12864-024-10600-y. BMC Genomics. 2024. PMID: 39003468 Free PMC article.
-
Disease Heritability Enrichment of Regulatory Elements Is Concentrated in Elements with Ancient Sequence Age and Conserved Function across Species.Am J Hum Genet. 2019 Apr 4;104(4):611-624. doi: 10.1016/j.ajhg.2019.02.008. Epub 2019 Mar 21. Am J Hum Genet. 2019. PMID: 30905396 Free PMC article.
-
Quantifying missing heritability at known GWAS loci.PLoS Genet. 2013;9(12):e1003993. doi: 10.1371/journal.pgen.1003993. Epub 2013 Dec 26. PLoS Genet. 2013. PMID: 24385918 Free PMC article.
-
Finding the missing heritability of complex diseases.Nature. 2009 Oct 8;461(7265):747-53. doi: 10.1038/nature08494. Nature. 2009. PMID: 19812666 Free PMC article. Review.
Cited by
-
Genomic Prediction for Quantitative Traits Is Improved by Mapping Variants to Gene Ontology Categories in Drosophila melanogaster.Genetics. 2016 Aug;203(4):1871-83. doi: 10.1534/genetics.116.187161. Epub 2016 May 27. Genetics. 2016. PMID: 27235308 Free PMC article.
-
Identifying genes associated with brain volumetric differences through tissue specific transcriptomic inference from GWAS summary data.BMC Bioinformatics. 2022 Sep 28;23(Suppl 3):398. doi: 10.1186/s12859-022-04947-w. BMC Bioinformatics. 2022. PMID: 36171548 Free PMC article.
-
Powerful mapping of cis-genetic effects on gene expression across diverse populations reveals novel disease-critical genes.medRxiv [Preprint]. 2024 Sep 26:2024.09.25.24314410. doi: 10.1101/2024.09.25.24314410. medRxiv. 2024. PMID: 39399015 Free PMC article. Preprint.
-
Type 2 diabetes: genetic data sharing to advance complex disease research.Nat Rev Genet. 2016 Sep;17(9):535-49. doi: 10.1038/nrg.2016.56. Epub 2016 Jul 11. Nat Rev Genet. 2016. PMID: 27402621 Review.
-
The impact of host genetic variation on infection with HIV-1.Nat Immunol. 2015 Jun;16(6):577-83. doi: 10.1038/ni.3147. Nat Immunol. 2015. PMID: 25988890 Free PMC article. Review.
References
Publication types
MeSH terms
Grants and funding
- F32 GM106584/GM/NIGMS NIH HHS/United States
- G0601635/MRC_/Medical Research Council/United Kingdom
- SCD/12/CSO_/Chief Scientist Office/United Kingdom
- 1U01HG0070033/HG/NHGRI NIH HHS/United States
- 076113/WT_/Wellcome Trust/United Kingdom
- G0800509/MRC_/Medical Research Council/United Kingdom
- R03 HG006731/HG/NHGRI NIH HHS/United States
- R01 MH085548/MH/NIMH NIH HHS/United States
- R01 AR063759/AR/NIAMS NIH HHS/United States
- R01 GM105857/GM/NIGMS NIH HHS/United States
- WT_/Wellcome Trust/United Kingdom
- R01 MH101244/MH/NIMH NIH HHS/United States
- U01 MH094432/MH/NIMH NIH HHS/United States
- U01 MH096296/MH/NIMH NIH HHS/United States
- MR/L010305/1/MRC_/Medical Research Council/United Kingdom
- T32 MH020030/MH/NIMH NIH HHS/United States
LinkOut - more resources
Full Text Sources
Other Literature Sources
Medical