MS-GF+ makes progress towards a universal database search tool for proteomics
- PMID: 25358478
- PMCID: PMC5036525
- DOI: 10.1038/ncomms6277
MS-GF+ makes progress towards a universal database search tool for proteomics
Abstract
Mass spectrometry (MS) instruments and experimental protocols are rapidly advancing, but the software tools to analyse tandem mass spectra are lagging behind. We present a database search tool MS-GF+ that is sensitive (it identifies more peptides than most other database search tools) and universal (it works well for diverse types of spectra, different configurations of MS instruments and different experimental protocols). We benchmark MS-GF+ using diverse spectral data sets: (i) spectra of varying fragmentation methods; (ii) spectra of multiple enzyme digests; (iii) spectra of phosphorylated peptides; and (iv) spectra of peptides with unusual fragmentation propensities produced by a novel alpha-lytic protease. For all these data sets, MS-GF+ significantly increases the number of identified peptides compared with commonly used methods for peptide identifications. We emphasize that although MS-GF+ is not specifically designed for any particular experimental set-up, it improves on the performance of tools specifically designed for these applications (for example, specialized tools for phosphoproteomics).
Conflict of interest statement
Contributions S.K. and P.P. designed the algorithms and the experiments and wrote the manuscript. S.K. implemented the algorithms and performed the data analysis. The authors declare no conflict of interest.
Figures
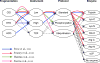
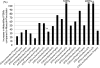
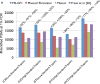
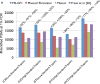
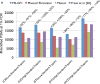
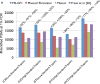
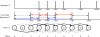
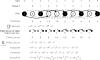
Similar articles
-
In-depth analysis of protein inference algorithms using multiple search engines and well-defined metrics.J Proteomics. 2017 Jan 6;150:170-182. doi: 10.1016/j.jprot.2016.08.002. Epub 2016 Aug 4. J Proteomics. 2017. PMID: 27498275
-
PepArML: A Meta-Search Peptide Identification Platform for Tandem Mass Spectra.Curr Protoc Bioinformatics. 2013 Dec;44(1323):13.23.1-23. doi: 10.1002/0471250953.bi1323s44. Curr Protoc Bioinformatics. 2013. PMID: 25663956 Free PMC article.
-
Data-Dependent Scoring Parameter Optimization in MS-GF+ Using Spectrum Quality Filter.J Proteome Res. 2018 Oct 5;17(10):3593-3598. doi: 10.1021/acs.jproteome.8b00415. Epub 2018 Jul 26. J Proteome Res. 2018. PMID: 30033731
-
The spectral networks paradigm in high throughput mass spectrometry.Mol Biosyst. 2012 Oct;8(10):2535-44. doi: 10.1039/c2mb25085c. Mol Biosyst. 2012. PMID: 22610447 Free PMC article. Review.
-
Peptide identification by tandem mass spectrometry with alternate fragmentation modes.Mol Cell Proteomics. 2012 Sep;11(9):550-7. doi: 10.1074/mcp.R112.018556. Epub 2012 May 17. Mol Cell Proteomics. 2012. PMID: 22595789 Free PMC article. Review.
Cited by
-
Short-term acidification promotes diverse iron acquisition and conservation mechanisms in upwelling-associated phytoplankton.Nat Commun. 2023 Nov 8;14(1):7215. doi: 10.1038/s41467-023-42949-1. Nat Commun. 2023. PMID: 37940668 Free PMC article.
-
Comprehensive Overview of Bottom-Up Proteomics using Mass Spectrometry.ArXiv [Preprint]. 2023 Nov 13:arXiv:2311.07791v1. ArXiv. 2023. Update in: ACS Meas Sci Au. 2024 Jun 04;4(4):338-417. doi: 10.1021/acsmeasuresciau.3c00068 PMID: 38013887 Free PMC article. Updated. Preprint.
-
A pan-cancer transcriptome analysis of exitron splicing identifies novel cancer driver genes and neoepitopes.Mol Cell. 2021 May 20;81(10):2246-2260.e12. doi: 10.1016/j.molcel.2021.03.028. Epub 2021 Apr 15. Mol Cell. 2021. PMID: 33861991 Free PMC article.
-
Remodeling of the human skeletal muscle proteome found after long-term endurance training but not after strength training.iScience. 2023 Dec 5;27(1):108638. doi: 10.1016/j.isci.2023.108638. eCollection 2024 Jan 19. iScience. 2023. PMID: 38213622 Free PMC article.
-
Identification of modified peptides using localization-aware open search.Nat Commun. 2020 Aug 13;11(1):4065. doi: 10.1038/s41467-020-17921-y. Nat Commun. 2020. PMID: 32792501 Free PMC article.
References
-
- Eng J, McCormack A, Yates J. An approach to correlate tandem mass spectral data of peptides with amino acid sequences in a protein database. J Am Soc Mass Spectrom. 1994;5:976–89. - PubMed
-
- Perkins D, Pappin D, Creasy D, Cottrell J. Probability-based protein identification by searching sequence databases using mass spectrometry data. Electrophoresis. 1999;20:3551–67. - PubMed
-
- Cox J, et al. Andromeda: A peptide search engine integrated into the maxquant environment. J Proteome Res. 2011;10:1794–805. - PubMed
Publication types
MeSH terms
Substances
Grants and funding
LinkOut - more resources
Full Text Sources
Other Literature Sources
Molecular Biology Databases