Identification of causal genetic drivers of human disease through systems-level analysis of regulatory networks
- PMID: 25303533
- PMCID: PMC4194029
- DOI: 10.1016/j.cell.2014.09.021
Identification of causal genetic drivers of human disease through systems-level analysis of regulatory networks
Erratum in
-
Identification of Causal Genetic Drivers of Human Disease through Systems-Level Analysis of Regulatory Networks.Cell. 2016 Aug 11;166(4):1055. doi: 10.1016/j.cell.2016.07.036. Cell. 2016. PMID: 27518566 No abstract available.
Abstract
Identification of driver mutations in human diseases is often limited by cohort size and availability of appropriate statistical models. We propose a framework for the systematic discovery of genetic alterations that are causal determinants of disease, by prioritizing genes upstream of functional disease drivers, within regulatory networks inferred de novo from experimental data. We tested this framework by identifying the genetic determinants of the mesenchymal subtype of glioblastoma. Our analysis uncovered KLHL9 deletions as upstream activators of two previously established master regulators of the subtype, C/EBPβ and C/EBPδ. Rescue of KLHL9 expression induced proteasomal degradation of C/EBP proteins, abrogated the mesenchymal signature, and reduced tumor viability in vitro and in vivo. Deletions of KLHL9 were confirmed in > 50% of mesenchymal cases in an independent cohort, thus representing the most frequent genetic determinant of the subtype. The method generalized to study other human diseases, including breast cancer and Alzheimer's disease.
Copyright © 2014 Elsevier Inc. All rights reserved.
Figures
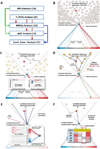
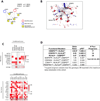
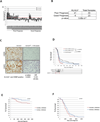
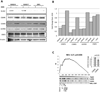
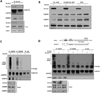
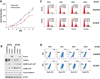
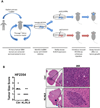
Similar articles
-
Proteasome-mediated CCAAT/enhancer-binding protein delta (C/EBPdelta) degradation is ubiquitin-independent.Biochem J. 2007 Jul 15;405(2):341-9. doi: 10.1042/BJ20070082. Biochem J. 2007. PMID: 17373909 Free PMC article.
-
The CCAAT/enhancer binding protein (C/EBP) δ is differently regulated by fibrillar and oligomeric forms of the Alzheimer amyloid-β peptide.J Neuroinflammation. 2011 Apr 14;8:34. doi: 10.1186/1742-2094-8-34. J Neuroinflammation. 2011. PMID: 21492414 Free PMC article.
-
DIGGIT: a Bioconductor package to infer genetic variants driving cellular phenotypes.Bioinformatics. 2015 Dec 15;31(24):4032-4. doi: 10.1093/bioinformatics/btv499. Epub 2015 Sep 2. Bioinformatics. 2015. PMID: 26338767 Free PMC article.
-
Human disorders of ubiquitination and proteasomal degradation.Curr Opin Pediatr. 2004 Aug;16(4):419-26. doi: 10.1097/01.mop.0000133634.79661.cd. Curr Opin Pediatr. 2004. PMID: 15273504 Review.
-
C/EBPβ and C/EBPδ transcription factors: Basic biology and roles in the CNS.Prog Neurobiol. 2015 Sep;132:1-33. doi: 10.1016/j.pneurobio.2015.06.003. Epub 2015 Jul 2. Prog Neurobiol. 2015. PMID: 26143335 Review.
Cited by
-
Transcriptional and metabolic effects of aspartate-glutamate carrier isoform 1 (AGC1) downregulation in mouse oligodendrocyte precursor cells (OPCs).Cell Mol Biol Lett. 2024 Mar 29;29(1):44. doi: 10.1186/s11658-024-00563-z. Cell Mol Biol Lett. 2024. PMID: 38553684 Free PMC article.
-
Elucidation and Pharmacological Targeting of Novel Molecular Drivers of Follicular Lymphoma Progression.Cancer Res. 2016 Feb 1;76(3):664-74. doi: 10.1158/0008-5472.CAN-15-0828. Epub 2015 Nov 20. Cancer Res. 2016. PMID: 26589882 Free PMC article.
-
Tuning Cancer Fate: Tumor Microenvironment's Role in Cancer Stem Cell Quiescence and Reawakening.Front Immunol. 2020 Oct 21;11:2166. doi: 10.3389/fimmu.2020.02166. eCollection 2020. Front Immunol. 2020. PMID: 33193295 Free PMC article. Review.
-
Identification of key regulators of pancreatic cancer progression through multidimensional systems-level analysis.Genome Med. 2016 May 3;8(1):38. doi: 10.1186/s13073-016-0282-3. Genome Med. 2016. PMID: 27137215 Free PMC article.
-
Inferring latent temporal progression and regulatory networks from cross-sectional transcriptomic data of cancer samples.PLoS Comput Biol. 2021 Mar 5;17(3):e1008379. doi: 10.1371/journal.pcbi.1008379. eCollection 2021 Mar. PLoS Comput Biol. 2021. PMID: 33667222 Free PMC article.
References
-
- Aytes A, Mitrofanova A, Lefebvre C, Alvarez MJ, Castillo-Martin M, Zheng T, Eastham JA, Gopalan A, Pienta K, Shen MM, et al. Cross-species analysis of genome-wide regulatory networks identifies a synergistic interaction between FOXM1 and CENPF that drives prostate cancer malignancy. Cancer Cell. 2014a;25:638–651. - PMC - PubMed
-
- Aytes A, Mitrofanova A, Lefebvre C, Alvarez MJ, Castillo-Martin M, Zheng T, Eastham JA, Gopalan A, Pienta KJ, Shen MM, et al. Cross-species regulatory network analysis identifies a synergistic interaction between FOXM1 and CENPF that drives prostate cancer malignancy. Cancer Cell. 2014b;25:638–651. - PMC - PubMed
-
- Basso K, Margolin AA, Stolovitzky G, Klein U, Dalla-Favera R, Califano A. Reverse engineering of regulatory networks in human B cells. Nat Genet. 2005;37:382–390. - PubMed
Publication types
MeSH terms
Substances
Grants and funding
- T32 GM082797/GM/NIGMS NIH HHS/United States
- RC2 CA148308/CA/NCI NIH HHS/United States
- U54CA121852/CA/NCI NIH HHS/United States
- U01 CA168426/CA/NCI NIH HHS/United States
- 1RC2CA148308-01/CA/NCI NIH HHS/United States
- T32 GM007088/GM/NIGMS NIH HHS/United States
- R01 NS061776-05/NS/NINDS NIH HHS/United States
- U01 CA168397/CA/NCI NIH HHS/United States
- U54 CA121852/CA/NCI NIH HHS/United States
- P01CA154293/CA/NCI NIH HHS/United States
- P50 CA127001/CA/NCI NIH HHS/United States
- R01 NS061776/NS/NINDS NIH HHS/United States
- T32GM082797/GM/NIGMS NIH HHS/United States
- 5U01CA16842603/CA/NCI NIH HHS/United States
- P01 CA154293/CA/NCI NIH HHS/United States
- P50CA127001/CA/NCI NIH HHS/United States
LinkOut - more resources
Full Text Sources
Other Literature Sources
Molecular Biology Databases