Proteogenomic characterization of human colon and rectal cancer
- PMID: 25043054
- PMCID: PMC4249766
- DOI: 10.1038/nature13438
Proteogenomic characterization of human colon and rectal cancer
Abstract
Extensive genomic characterization of human cancers presents the problem of inference from genomic abnormalities to cancer phenotypes. To address this problem, we analysed proteomes of colon and rectal tumours characterized previously by The Cancer Genome Atlas (TCGA) and perform integrated proteogenomic analyses. Somatic variants displayed reduced protein abundance compared to germline variants. Messenger RNA transcript abundance did not reliably predict protein abundance differences between tumours. Proteomics identified five proteomic subtypes in the TCGA cohort, two of which overlapped with the TCGA 'microsatellite instability/CpG island methylation phenotype' transcriptomic subtype, but had distinct mutation, methylation and protein expression patterns associated with different clinical outcomes. Although copy number alterations showed strong cis- and trans-effects on mRNA abundance, relatively few of these extend to the protein level. Thus, proteomics data enabled prioritization of candidate driver genes. The chromosome 20q amplicon was associated with the largest global changes at both mRNA and protein levels; proteomics data highlighted potential 20q candidates, including HNF4A (hepatocyte nuclear factor 4, alpha), TOMM34 (translocase of outer mitochondrial membrane 34) and SRC (SRC proto-oncogene, non-receptor tyrosine kinase). Integrated proteogenomic analysis provides functional context to interpret genomic abnormalities and affords a new paradigm for understanding cancer biology.
Conflict of interest statement
The authors declare no competing financial interests.
Figures
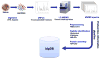
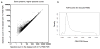
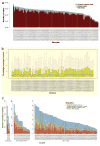
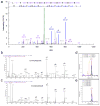
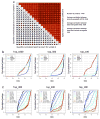
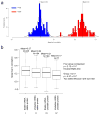
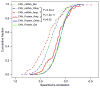
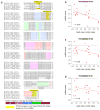
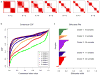
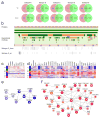
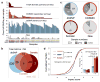
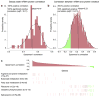
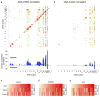
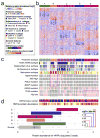
Comment in
-
Proteogenomics sheds light on tumors.Cancer Discov. 2014 Oct;4(10):1108. doi: 10.1158/2159-8290.CD-NB2014-123. Epub 2014 Aug 14. Cancer Discov. 2014. PMID: 25274663 No abstract available.
Similar articles
-
A comparison of colon and rectal somatic DNA alterations.Dis Colon Rectum. 2009 Jul;52(7):1304-11. doi: 10.1007/DCR.0b013e3181a0e5df. Dis Colon Rectum. 2009. PMID: 19571709 Free PMC article.
-
Integrated analysis of genome-wide copy number alterations and gene expression in microsatellite stable, CpG island methylator phenotype-negative colon cancer.Genes Chromosomes Cancer. 2013 May;52(5):450-66. doi: 10.1002/gcc.22043. Epub 2013 Jan 23. Genes Chromosomes Cancer. 2013. PMID: 23341073 Free PMC article.
-
Chr20q Amplification Defines a Distinct Molecular Subtype of Microsatellite Stable Colorectal Cancer.Cancer Res. 2021 Apr 15;81(8):1977-1987. doi: 10.1158/0008-5472.CAN-20-4009. Epub 2021 Feb 22. Cancer Res. 2021. PMID: 33619118 Free PMC article.
-
[Colorectal carcinogenesis. 2. Underlying epigenetic and genetic alterations and molecular classification of colorectal cancers].J Chir (Paris). 2007 Mar-Apr;144(2):97-104. doi: 10.1016/s0021-7697(07)89480-4. J Chir (Paris). 2007. PMID: 17607223 Review. French.
-
Gene methylation in gastric cancer.Clin Chim Acta. 2013 Sep 23;424:53-65. doi: 10.1016/j.cca.2013.05.002. Epub 2013 May 10. Clin Chim Acta. 2013. PMID: 23669186 Review.
Cited by
-
PDX models reflect the proteome landscape of pediatric acute lymphoblastic leukemia but divert in select pathways.J Exp Clin Cancer Res. 2021 Mar 15;40(1):96. doi: 10.1186/s13046-021-01835-8. J Exp Clin Cancer Res. 2021. PMID: 33722259 Free PMC article.
-
Leveraging premalignant biology for immune-based cancer prevention.Proc Natl Acad Sci U S A. 2016 Sep 27;113(39):10750-8. doi: 10.1073/pnas.1608077113. Epub 2016 Sep 16. Proc Natl Acad Sci U S A. 2016. PMID: 27638202 Free PMC article.
-
Population-scale proteome variation in human induced pluripotent stem cells.Elife. 2020 Aug 10;9:e57390. doi: 10.7554/eLife.57390. Elife. 2020. PMID: 32773033 Free PMC article.
-
Quantitative Analysis of the Proteome and the Succinylome in the Thyroid Tissue of High-Fat Diet-Induced Hypothyroxinemia in Rats.Int J Endocrinol. 2020 Jul 23;2020:3240198. doi: 10.1155/2020/3240198. eCollection 2020. Int J Endocrinol. 2020. PMID: 32774361 Free PMC article.
-
Stable gene expression for normalisation and single-sample scoring.Nucleic Acids Res. 2020 Nov 4;48(19):e113. doi: 10.1093/nar/gkaa802. Nucleic Acids Res. 2020. PMID: 32997146 Free PMC article.
References
Publication types
MeSH terms
Substances
Grants and funding
- P50CA095103/CA/NCI NIH HHS/United States
- R01 CA046413/CA/NCI NIH HHS/United States
- GM088822/GM/NIGMS NIH HHS/United States
- R01 GM088822/GM/NIGMS NIH HHS/United States
- U24 CA160034/CA/NCI NIH HHS/United States
- U24 CA159988/CA/NCI NIH HHS/United States
- P50 CA095103/CA/NCI NIH HHS/United States
- P30 DK058404/DK/NIDDK NIH HHS/United States
- U24CA159988/CA/NCI NIH HHS/United States
- P30 CA068485/CA/NCI NIH HHS/United States
- U24 CA160019/CA/NCI NIH HHS/United States
- U54 HG003079/HG/NHGRI NIH HHS/United States
- UL1 TR000448/TR/NCATS NIH HHS/United States
- U24 CA160035/CA/NCI NIH HHS/United States
- U24CA160034/CA/NCI NIH HHS/United States
- P30CA068485/CA/NCI NIH HHS/United States
- U24CA160035/CA/NCI NIH HHS/United States
LinkOut - more resources
Full Text Sources
Other Literature Sources
Molecular Biology Databases
Miscellaneous