Predictive performance of microarray gene signatures: impact of tumor heterogeneity and multiple mechanisms of drug resistance
- PMID: 24706696
- PMCID: PMC4040235
- DOI: 10.1158/0008-5472.CAN-13-3375
Predictive performance of microarray gene signatures: impact of tumor heterogeneity and multiple mechanisms of drug resistance
Abstract
Gene signatures have failed to predict responses to breast cancer therapy in patients to date. In this study, we used bioinformatic methods to explore the hypothesis that the existence of multiple drug resistance mechanisms in different patients may limit the power of gene signatures to predict responses to therapy. In addition, we explored whether substratification of resistant cases could improve performance. Gene expression profiles from 1,550 breast cancers analyzed with the same microarray platform were retrieved from publicly available sources. Gene expression changes were introduced in cases defined as sensitive or resistant to a hypothetical therapy. In the resistant group, up to five different mechanisms of drug resistance causing distinct or overlapping gene expression changes were generated bioinformatically, and their impact on sensitivity, specificity, and predictive values of the signatures was investigated. We found that increasing the number of resistance mechanisms corresponding to different gene expression changes weakened the performance of the predictive signatures generated, even if the resistance-induced changes in gene expression were sufficiently strong and informative. Performance was also affected by cohort composition and the proportion of sensitive versus resistant cases or resistant cases that were mechanistically distinct. It was possible to improve response prediction by substratifying chemotherapy-resistant cases from actual datasets (non-bioinformatically perturbed datasets) and by using outliers to model multiple resistance mechanisms. Our work supports the hypothesis that the presence of multiple resistance mechanisms in a given therapy in patients limits the ability of gene signatures to make clinically useful predictions.
©2014 American Association for Cancer Research.
Figures
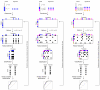
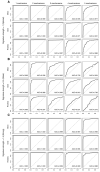
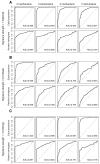
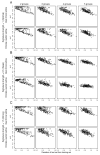
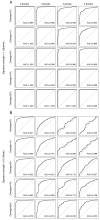
Similar articles
-
cDNA microarray analysis of isogenic paclitaxel- and doxorubicin-resistant breast tumor cell lines reveals distinct drug-specific genetic signatures of resistance.Breast Cancer Res Treat. 2006 Mar;96(1):17-39. doi: 10.1007/s10549-005-9026-6. Epub 2005 Dec 2. Breast Cancer Res Treat. 2006. PMID: 16322897
-
Evaluation of public cancer datasets and signatures identifies TP53 mutant signatures with robust prognostic and predictive value.BMC Cancer. 2015 Mar 26;15:179. doi: 10.1186/s12885-015-1102-7. BMC Cancer. 2015. PMID: 25886164 Free PMC article.
-
Changes in expression of oestrogen regulated and proliferation genes with neoadjuvant treatment highlight heterogeneity of clinical resistance to the aromatase inhibitor, letrozole.Breast Cancer Res. 2010;12(4):R52. doi: 10.1186/bcr2611. Epub 2010 Jul 20. Breast Cancer Res. 2010. PMID: 20646288 Free PMC article. Clinical Trial.
-
Clinical implications of chemotherapy-induced tumor gene expression in human breast cancers.Expert Opin Drug Metab Toxicol. 2010 Mar;6(3):283-306. doi: 10.1517/17425250903510611. Expert Opin Drug Metab Toxicol. 2010. PMID: 20163320 Review.
-
Overview of resistance to systemic therapy in patients with breast cancer.Adv Exp Med Biol. 2007;608:1-22. doi: 10.1007/978-0-387-74039-3_1. Adv Exp Med Biol. 2007. PMID: 17993229 Review.
Cited by
-
ISOpureR: an R implementation of a computational purification algorithm of mixed tumour profiles.BMC Bioinformatics. 2015 May 14;16:156. doi: 10.1186/s12859-015-0597-x. BMC Bioinformatics. 2015. PMID: 25972088 Free PMC article.
-
Benchmarking mutation effect prediction algorithms using functionally validated cancer-related missense mutations.Genome Biol. 2014 Oct 28;15(10):484. doi: 10.1186/s13059-014-0484-1. Genome Biol. 2014. PMID: 25348012 Free PMC article.
-
Statistical measures of transcriptional diversity capture genomic heterogeneity of cancer.BMC Genomics. 2014 Oct 8;15(1):876. doi: 10.1186/1471-2164-15-876. BMC Genomics. 2014. PMID: 25294321 Free PMC article.
-
Resistance mechanisms to drug therapy in breast cancer and other solid tumors: An opinion.F1000Res. 2017 Mar 17;6:288. doi: 10.12688/f1000research.10992.1. eCollection 2017. F1000Res. 2017. PMID: 28751966 Free PMC article.
-
New tools for old drugs: Functional genetic screens to optimize current chemotherapy.Drug Resist Updat. 2018 Jan;36:30-46. doi: 10.1016/j.drup.2018.01.001. Epub 2018 Jan 12. Drug Resist Updat. 2018. PMID: 29499836 Free PMC article. Review.
References
-
- Reis-Filho JS, Pusztai L. Gene expression profiling in breast cancer: classification, prognostication, and prediction. Lancet. 2011;378:1812–23. - PubMed
-
- van ’t Veer LJ, Dai H, van de Vijver MJ, He YD, Hart AA, Mao M, et al. Gene expression profiling predicts clinical outcome of breast cancer. Nature. 2002;415:530–6. - PubMed
-
- Weigelt B, Pusztai L, Ashworth A, Reis JS. Challenges translating breast cancer gene signatures into the clinic. Nat Rev Clin Oncol. 2012;9:58–64. - PubMed
-
- Perou CM, Sorlie T, Eisen MB, van de Rijn M, Jeffrey SS, Rees CA, et al. Molecular portraits of human breast tumours. Nature. 2000;406:747–52. - PubMed
Publication types
MeSH terms
Substances
Grants and funding
LinkOut - more resources
Full Text Sources
Other Literature Sources
Medical