A computational method for predicting regulation of human microRNAs on the influenza virus genome
- PMID: 24565017
- PMCID: PMC3851852
- DOI: 10.1186/1752-0509-7-S2-S3
A computational method for predicting regulation of human microRNAs on the influenza virus genome
Abstract
Background: While it has been suggested that host microRNAs (miRNAs) may downregulate viral gene expression as an antiviral defense mechanism, such a mechanism has not been explored in the influenza virus for human flu studies. As it is difficult to conduct related experiments on humans, computational studies can provide some insight. Although many computational tools have been designed for miRNA target prediction, there is a need for cross-species prediction, especially for predicting viral targets of human miRNAs. However, finding putative human miRNAs targeting influenza virus genome is still challenging.
Results: We developed machine-learning features and conducted comprehensive data training for predicting interactions between H1N1 genome segments and host miRNA. We defined our seed region as the first ten nucleotides from the 5' end of the miRNA to the 3' end of the miRNA and integrated various features including the number of consecutive matching bases in the seed region of 10 bases, a triplet feature in seed regions, thermodynamic energy, penalty of bulges and wobbles at binding sites, and the secondary structure of viral RNA for the prediction.
Conclusions: Compared to general predictive models, our model fully takes into account the conservation patterns and features of viral RNA secondary structures, and greatly improves the prediction accuracy. Our model identified some key miRNAs including hsa-miR-489, hsa-miR-325, hsa-miR-876-3p and hsa-miR-2117, which target HA, PB2, MP and NS of H1N1, respectively. Our study provided an interesting hypothesis concerning the miRNA-based antiviral defense mechanism against influenza virus in human, i.e., the binding between human miRNA and viral RNAs may not result in gene silencing but rather may block the viral RNA replication.
Figures
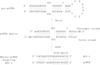
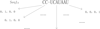
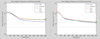
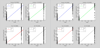
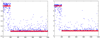
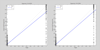
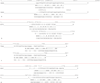
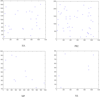
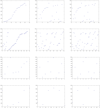
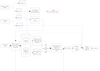
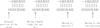
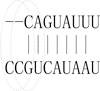
Similar articles
-
The highly pathogenic H5N1 influenza A virus down-regulated several cellular MicroRNAs which target viral genome.J Cell Mol Med. 2017 Nov;21(11):3076-3086. doi: 10.1111/jcmm.13219. Epub 2017 Jun 13. J Cell Mol Med. 2017. PMID: 28609011 Free PMC article.
-
Cellular microRNAs inhibit replication of the H1N1 influenza A virus in infected cells.J Virol. 2010 Sep;84(17):8849-60. doi: 10.1128/JVI.00456-10. Epub 2010 Jun 16. J Virol. 2010. PMID: 20554777 Free PMC article.
-
Sus scrofa miR-204 and miR-4331 Negatively Regulate Swine H1N1/2009 Influenza A Virus Replication by Targeting Viral HA and NS, Respectively.Int J Mol Sci. 2017 Apr 3;18(4):749. doi: 10.3390/ijms18040749. Int J Mol Sci. 2017. PMID: 28368362 Free PMC article.
-
Computational approaches for microRNA studies: a review.Mamm Genome. 2010 Feb;21(1-2):1-12. doi: 10.1007/s00335-009-9241-2. Epub 2009 Dec 15. Mamm Genome. 2010. PMID: 20012966 Review.
-
Computational Resources for Prediction and Analysis of Functional miRNA and Their Targetome.Methods Mol Biol. 2019;1912:215-250. doi: 10.1007/978-1-4939-8982-9_9. Methods Mol Biol. 2019. PMID: 30635896 Review.
Cited by
-
Human miR-3145 inhibits influenza A viruses replication by targeting and silencing viral PB1 gene.Exp Biol Med (Maywood). 2015 Dec;240(12):1630-9. doi: 10.1177/1535370215589051. Epub 2015 Jun 15. Exp Biol Med (Maywood). 2015. PMID: 26080461 Free PMC article.
-
Compounds with anti-influenza activity: present and future of strategies for the optimal treatment and management of influenza. Part II: Future compounds against influenza virus.J Prev Med Hyg. 2014 Dec;55(4):109-29. J Prev Med Hyg. 2014. PMID: 26137785 Free PMC article. Review.
-
A Relationship Prediction Method for Magnaporthe oryzae-Rice Multi-Omics Data Based on WGCNA and Graph Autoencoder.J Fungi (Basel). 2023 Oct 12;9(10):1007. doi: 10.3390/jof9101007. J Fungi (Basel). 2023. PMID: 37888263 Free PMC article.
-
MiR-876-5p suppresses epithelial-mesenchymal transition of lung cancer by directly down-regulating bone morphogenetic protein 4.J Biosci. 2017 Dec;42(4):671-681. doi: 10.1007/s12038-017-9722-5. J Biosci. 2017. PMID: 29229885
-
MicroRNA Regulation of RNA Virus Replication and Pathogenesis.Trends Mol Med. 2017 Jan;23(1):80-93. doi: 10.1016/j.molmed.2016.11.003. Epub 2016 Dec 16. Trends Mol Med. 2017. PMID: 27989642 Free PMC article. Review.
References
-
- Nakajima K. [Influenza virus genome structure and encoded proteins] Nihon rinsho Japanese journal of clinical medicine. 1997;55(10):2542–2546. - PubMed
-
- Novel Swine-Origin Influenza AVIT. Dawood FS, Jain S, Finelli L, Shaw MW, Lindstrom S, Garten RJ, Gubareva LV, Xu X, Bridges CB. et al.Emergence of a novel swine-origin influenza A (H1N1) virus in humans. The New England journal of medicine. 2009;360(25):2605–2615. - PubMed
Publication types
MeSH terms
Substances
LinkOut - more resources
Full Text Sources
Other Literature Sources
Research Materials
Miscellaneous