Beyond modules and hubs: the potential of gene coexpression networks for investigating molecular mechanisms of complex brain disorders
- PMID: 24320616
- PMCID: PMC3896950
- DOI: 10.1111/gbb.12106
Beyond modules and hubs: the potential of gene coexpression networks for investigating molecular mechanisms of complex brain disorders
Abstract
In a research environment dominated by reductionist approaches to brain disease mechanisms, gene network analysis provides a complementary framework in which to tackle the complex dysregulations that occur in neuropsychiatric and other neurological disorders. Gene-gene expression correlations are a common source of molecular networks because they can be extracted from high-dimensional disease data and encapsulate the activity of multiple regulatory systems. However, the analysis of gene coexpression patterns is often treated as a mechanistic black box, in which looming 'hub genes' direct cellular networks, and where other features are obscured. By examining the biophysical bases of coexpression and gene regulatory changes that occur in disease, recent studies suggest it is possible to use coexpression networks as a multi-omic screening procedure to generate novel hypotheses for disease mechanisms. Because technical processing steps can affect the outcome and interpretation of coexpression networks, we examine the assumptions and alternatives to common patterns of coexpression analysis and discuss additional topics such as acceptable datasets for coexpression analysis, the robust identification of modules, disease-related prioritization of genes and molecular systems and network meta-analysis. To accelerate coexpression research beyond modules and hubs, we highlight some emerging directions for coexpression network research that are especially relevant to complex brain disease, including the centrality-lethality relationship, integration with machine learning approaches and network pharmacology.
Keywords: Coexpression; complex diseases; coregulation; depression; gene; hub; module; network; post-mortem; regulatory network; small-world; transcriptome.
© 2013 John Wiley & Sons Ltd and International Behavioural and Neural Genetics Society.
Figures
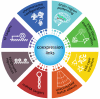
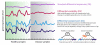
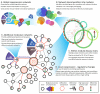
Similar articles
-
Differentially expressed genes in major depression reside on the periphery of resilient gene coexpression networks.Front Neurosci. 2011 Aug 30;5:95. doi: 10.3389/fnins.2011.00095. eCollection 2011. Front Neurosci. 2011. PMID: 21922000 Free PMC article.
-
Identification of common coexpression modules based on quantitative network comparison.BMC Bioinformatics. 2018 Jun 13;19(Suppl 8):213. doi: 10.1186/s12859-018-2193-3. BMC Bioinformatics. 2018. PMID: 29897320 Free PMC article.
-
Identification of co‑expression modules and hub genes of retinoblastoma via co‑expression analysis and protein‑protein interaction networks.Mol Med Rep. 2020 Aug;22(2):1155-1168. doi: 10.3892/mmr.2020.11189. Epub 2020 May 27. Mol Med Rep. 2020. PMID: 32468072 Free PMC article.
-
Recent advances in gene function prediction using context-specific coexpression networks in plants.F1000Res. 2019 Feb 5;8:F1000 Faculty Rev-153. doi: 10.12688/f1000research.17207.1. eCollection 2019. F1000Res. 2019. PMID: 30800290 Free PMC article. Review.
-
Coexpression and cosplicing network approaches for the study of mammalian brain transcriptomes.Int Rev Neurobiol. 2014;116:73-93. doi: 10.1016/B978-0-12-801105-8.00004-7. Int Rev Neurobiol. 2014. PMID: 25172472 Review.
Cited by
-
GWENA: gene co-expression networks analysis and extended modules characterization in a single Bioconductor package.BMC Bioinformatics. 2021 May 25;22(1):267. doi: 10.1186/s12859-021-04179-4. BMC Bioinformatics. 2021. PMID: 34034647 Free PMC article.
-
Altered expression of neuroplasticity-related genes in the brain of depressed suicides.Neuroscience. 2015 Jul 23;299:1-17. doi: 10.1016/j.neuroscience.2015.04.057. Epub 2015 Apr 28. Neuroscience. 2015. PMID: 25934039 Free PMC article.
-
Leptin receptor co-expression gene network moderates the effect of early life adversity on eating behavior in children.Commun Biol. 2022 Oct 14;5(1):1092. doi: 10.1038/s42003-022-03992-8. Commun Biol. 2022. PMID: 36241774 Free PMC article.
-
Graphlet Based Metrics for the Comparison of Gene Regulatory Networks.PLoS One. 2016 Oct 3;11(10):e0163497. doi: 10.1371/journal.pone.0163497. eCollection 2016. PLoS One. 2016. PMID: 27695050 Free PMC article.
-
Exploring Potential Biomarkers Underlying Pathogenesis of Alzheimer's Disease by Differential Co-expression Analysis.Avicenna J Med Biotechnol. 2018 Oct-Dec;10(4):233-241. Avicenna J Med Biotechnol. 2018. PMID: 30555656 Free PMC article.
References
-
- Albert R, Jeong H, Barabasi A-L. Error and attack tolerance of complex networks. Nature. 2000;406:378–382. - PubMed
Publication types
MeSH terms
Grants and funding
LinkOut - more resources
Full Text Sources
Other Literature Sources
Medical