Evaluating a linear k-mer model for protein-DNA interactions using high-throughput SELEX data
- PMID: 24267147
- PMCID: PMC3750486
- DOI: 10.1186/1471-2105-14-S10-S2
Evaluating a linear k-mer model for protein-DNA interactions using high-throughput SELEX data
Abstract
Transcription factor (TF) binding to DNA can be modeled in a number of different ways. It is highly debated which modeling methods are the best, how the models should be built and what can they be applied to. In this study a linear k-mer model proposed for predicting TF specificity in protein binding microarrays (PBM) is applied to a high-throughput SELEX data and the question of how to choose the most informative k-mers to the binding model is studied. We implemented the standard cross-validation scheme to reduce the number of k-mers in the model and observed that the number of k-mers can often be reduced significantly without a great negative effect on prediction accuracy. We also found that the later SELEX enrichment cycles provide a much better discrimination between bound and unbound sequences as model prediction accuracies increased for all proteins together with the cycle number. We compared prediction performance of k-mer and position specific weight matrix (PWM) models derived from the same SELEX data. Consistent with previous results on PBM data, performance of the k-mer model was on average 9%-units better. For the 15 proteins in the SELEX data set with medium enrichment cycles, classification accuracies were on average 71% and 62% for k-mer and PWMs, respectively. Finally, the k-mer model trained with SELEX data was evaluated on ChIP-seq data demonstrating substantial improvements for some proteins. For protein GATA1 the model can distinquish between true ChIP-seq peaks and negative peaks. For proteins RFX3 and NFATC1 the performance of the model was no better than chance.
Figures
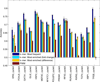
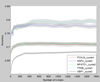
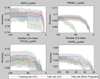
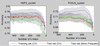
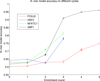
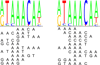
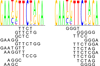
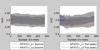
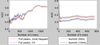
Similar articles
-
Transcription factor-binding k-mer analysis clarifies the cell type dependency of binding specificities and cis-regulatory SNPs in humans.BMC Genomics. 2023 Oct 7;24(1):597. doi: 10.1186/s12864-023-09692-9. BMC Genomics. 2023. PMID: 37805453 Free PMC article.
-
A comparative analysis of transcription factor binding models learned from PBM, HT-SELEX and ChIP data.Nucleic Acids Res. 2014 Apr;42(8):e63. doi: 10.1093/nar/gku117. Epub 2014 Feb 5. Nucleic Acids Res. 2014. PMID: 24500199 Free PMC article.
-
Quantitative modeling of transcription factor binding specificities using DNA shape.Proc Natl Acad Sci U S A. 2015 Apr 14;112(15):4654-9. doi: 10.1073/pnas.1422023112. Epub 2015 Mar 9. Proc Natl Acad Sci U S A. 2015. PMID: 25775564 Free PMC article.
-
In vitro DNA-binding profile of transcription factors: methods and new insights.J Endocrinol. 2011 Jul;210(1):15-27. doi: 10.1530/JOE-11-0010. Epub 2011 Mar 9. J Endocrinol. 2011. PMID: 21389103 Review.
-
[DNA-binding profiles of mammalian transcription factors].Yi Chuan. 2012 Aug;34(8):950-68. doi: 10.3724/sp.j.1005.2012.00950. Yi Chuan. 2012. PMID: 22917900 Review. Chinese.
Cited by
-
TFBSshape: an expanded motif database for DNA shape features of transcription factor binding sites.Nucleic Acids Res. 2020 Jan 8;48(D1):D246-D255. doi: 10.1093/nar/gkz970. Nucleic Acids Res. 2020. PMID: 31665425 Free PMC article.
-
Absence of a simple code: how transcription factors read the genome.Trends Biochem Sci. 2014 Sep;39(9):381-99. doi: 10.1016/j.tibs.2014.07.002. Epub 2014 Aug 14. Trends Biochem Sci. 2014. PMID: 25129887 Free PMC article. Review.
References
-
- Weirauch M, Cote A, Norel R, Annala M, Zhao Y, Riley T, Saez-Rodriguez J, Cokelaer T, Vedenko A, Talukder S. DREAM5 Consortium. Bussemaker H, Morris Q, Bulyk M, Stolovitzky G, Hughes T. Evaluation of methods for modeling transcription factor sequence specificity. Nature Biotechnology. 2013;2:126–34. - PMC - PubMed
-
- Bailey T, Elkan C. Fitting a mixture model by expectation maximization to discover motifs in biopolymers. Proceedings of the Second International Conference on Intelligent Systems for Molecular Biology. 1994;2:28–36. - PubMed
Publication types
MeSH terms
Substances
LinkOut - more resources
Full Text Sources
Other Literature Sources
Miscellaneous