Computational identification of diverse mechanisms underlying transcription factor-DNA occupancy
- PMID: 23935523
- PMCID: PMC3731213
- DOI: 10.1371/journal.pgen.1003571
Computational identification of diverse mechanisms underlying transcription factor-DNA occupancy
Abstract
ChIP-based genome-wide assays of transcription factor (TF) occupancy have emerged as a powerful, high-throughput method to understand transcriptional regulation, especially on a global scale. This has led to great interest in the underlying biochemical mechanisms that direct TF-DNA binding, with the ultimate goal of computationally predicting a TF's occupancy profile in any cellular condition. In this study, we examined the influence of various potential determinants of TF-DNA binding on a much larger scale than previously undertaken. We used a thermodynamics-based model of TF-DNA binding, called "STAP," to analyze 45 TF-ChIP data sets from Drosophila embryonic development. We built a cross-validation framework that compares a baseline model, based on the ChIP'ed ("primary") TF's motif, to more complex models where binding by secondary TFs is hypothesized to influence the primary TF's occupancy. Candidates interacting TFs were chosen based on RNA-SEQ expression data from the time point of the ChIP experiment. We found widespread evidence of both cooperative and antagonistic effects by secondary TFs, and explicitly quantified these effects. We were able to identify multiple classes of interactions, including (1) long-range interactions between primary and secondary motifs (separated by ≤150 bp), suggestive of indirect effects such as chromatin remodeling, (2) short-range interactions with specific inter-site spacing biases, suggestive of direct physical interactions, and (3) overlapping binding sites suggesting competitive binding. Furthermore, by factoring out the previously reported strong correlation between TF occupancy and DNA accessibility, we were able to categorize the effects into those that are likely to be mediated by the secondary TF's effect on local accessibility and those that utilize accessibility-independent mechanisms. Finally, we conducted in vitro pull-down assays to test model-based predictions of short-range cooperative interactions, and found that seven of the eight TF pairs tested physically interact and that some of these interactions mediate cooperative binding to DNA.
Conflict of interest statement
The authors have declared that no competing interests exist.
Figures
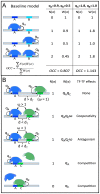
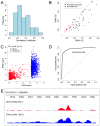

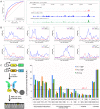
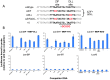
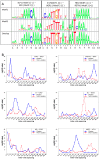
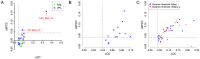
Similar articles
-
A biophysical model for analysis of transcription factor interaction and binding site arrangement from genome-wide binding data.PLoS One. 2009 Dec 1;4(12):e8155. doi: 10.1371/journal.pone.0008155. PLoS One. 2009. PMID: 19956545 Free PMC article.
-
Widespread evidence of cooperative DNA binding by transcription factors in Drosophila development.Nucleic Acids Res. 2013 Sep;41(17):8237-52. doi: 10.1093/nar/gkt598. Epub 2013 Jul 11. Nucleic Acids Res. 2013. PMID: 23847101 Free PMC article.
-
Heterogeneity of transcription factor binding specificity models within and across cell lines.Genome Res. 2016 Aug;26(8):1110-23. doi: 10.1101/gr.199166.115. Epub 2016 Jun 16. Genome Res. 2016. PMID: 27311443 Free PMC article.
-
Sequence and chromatin determinants of transcription factor binding and the establishment of cell type-specific binding patterns.Biochim Biophys Acta Gene Regul Mech. 2020 Jun;1863(6):194443. doi: 10.1016/j.bbagrm.2019.194443. Epub 2019 Oct 19. Biochim Biophys Acta Gene Regul Mech. 2020. PMID: 31639474 Free PMC article. Review.
-
Transcription factor-DNA binding: beyond binding site motifs.Curr Opin Genet Dev. 2017 Apr;43:110-119. doi: 10.1016/j.gde.2017.02.007. Epub 2017 Mar 27. Curr Opin Genet Dev. 2017. PMID: 28359978 Free PMC article. Review.
Cited by
-
ChIPulate: A comprehensive ChIP-seq simulation pipeline.PLoS Comput Biol. 2019 Mar 21;15(3):e1006921. doi: 10.1371/journal.pcbi.1006921. eCollection 2019 Mar. PLoS Comput Biol. 2019. PMID: 30897079 Free PMC article.
-
Estimating binding properties of transcription factors from genome-wide binding profiles.Nucleic Acids Res. 2015 Jan;43(1):84-94. doi: 10.1093/nar/gku1269. Epub 2014 Nov 28. Nucleic Acids Res. 2015. PMID: 25432957 Free PMC article.
-
Sex combs reduced (Scr) regulatory region of Drosophila revisited.Mol Genet Genomics. 2017 Aug;292(4):773-787. doi: 10.1007/s00438-017-1309-1. Epub 2017 Mar 22. Mol Genet Genomics. 2017. PMID: 28331962
-
Enhancer grammar in development, evolution, and disease: dependencies and interplay.Dev Cell. 2021 Mar 8;56(5):575-587. doi: 10.1016/j.devcel.2021.02.016. Dev Cell. 2021. PMID: 33689769 Free PMC article. Review.
-
Simulations of enhancer evolution provide mechanistic insights into gene regulation.Mol Biol Evol. 2014 Jan;31(1):184-200. doi: 10.1093/molbev/mst170. Epub 2013 Oct 4. Mol Biol Evol. 2014. PMID: 24097306 Free PMC article.
References
Publication types
MeSH terms
Substances
Grants and funding
LinkOut - more resources
Full Text Sources
Other Literature Sources
Molecular Biology Databases
Miscellaneous