Real-time control of walking using recordings from dorsal root ganglia
- PMID: 23928579
- PMCID: PMC3791106
- DOI: 10.1088/1741-2560/10/5/056008
Real-time control of walking using recordings from dorsal root ganglia
Abstract
Objective: The goal of this study was to decode sensory information from the dorsal root ganglia (DRG) in real time, and to use this information to adapt the control of unilateral stepping with a state-based control algorithm consisting of both feed-forward and feedback components.
Approach: In five anesthetized cats, hind limb stepping on a walkway or treadmill was produced by patterned electrical stimulation of the spinal cord through implanted microwire arrays, while neuronal activity was recorded from the DRG. Different parameters, including distance and tilt of the vector between hip and limb endpoint, integrated gyroscope and ground reaction force were modelled from recorded neural firing rates. These models were then used for closed-loop feedback.
Main results: Overall, firing-rate-based predictions of kinematic sensors (limb endpoint, integrated gyroscope) were the most accurate with variance accounted for >60% on average. Force prediction had the lowest prediction accuracy (48 ± 13%) but produced the greatest percentage of successful rule activations (96.3%) for stepping under closed-loop feedback control. The prediction of all sensor modalities degraded over time, with the exception of tilt.
Significance: Sensory feedback from moving limbs would be a desirable component of any neuroprosthetic device designed to restore walking in people after a spinal cord injury. This study provides a proof-of-principle that real-time feedback from the DRG is possible and could form part of a fully implantable neuroprosthetic device with further development.
Figures
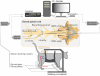
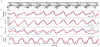
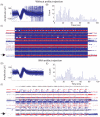
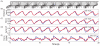
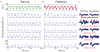
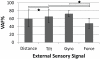
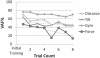
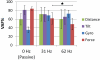
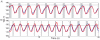
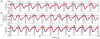
Similar articles
-
Real-time control of hind limb functional electrical stimulation using feedback from dorsal root ganglia recordings.J Neural Eng. 2013 Apr;10(2):026020. doi: 10.1088/1741-2560/10/2/026020. Epub 2013 Mar 15. J Neural Eng. 2013. PMID: 23503062 Free PMC article.
-
Online feedback control of functional electrical stimulation using dorsal root ganglia recordings.Annu Int Conf IEEE Eng Med Biol Soc. 2011;2011:7246-9. doi: 10.1109/IEMBS.2011.6091831. Annu Int Conf IEEE Eng Med Biol Soc. 2011. PMID: 22256011 Free PMC article.
-
Neuro-fuzzy decoding of sensory information from ensembles of simultaneously recorded dorsal root ganglion neurons for functional electrical stimulation applications.J Neural Eng. 2011 Aug;8(4):046019. doi: 10.1088/1741-2560/8/4/046019. Epub 2011 Jun 23. J Neural Eng. 2011. PMID: 21701057
-
Limb-state feedback from ensembles of simultaneously recorded dorsal root ganglion neurons.J Neural Eng. 2007 Sep;4(3):S168-80. doi: 10.1088/1741-2560/4/3/S04. Epub 2007 Aug 22. J Neural Eng. 2007. PMID: 17873416
-
Feed forward and feedback control for over-ground locomotion in anaesthetized cats.J Neural Eng. 2012 Apr;9(2):026003. doi: 10.1088/1741-2560/9/2/026003. Epub 2012 Feb 13. J Neural Eng. 2012. PMID: 22328615 Free PMC article.
Cited by
-
Flexible microelectrode array for interfacing with the surface of neural ganglia.J Neural Eng. 2018 Jun;15(3):036027. doi: 10.1088/1741-2552/aab55f. Epub 2018 Mar 9. J Neural Eng. 2018. PMID: 29521279 Free PMC article.
-
A Fuzzy Controller for Movement Stabilization Using Afferent Control: Controller Synthesis and Simulation.J Med Signals Sens. 2017 Oct-Dec;7(4):239-246. J Med Signals Sens. 2017. PMID: 29204381 Free PMC article.
-
A Review of Control Strategies in Closed-Loop Neuroprosthetic Systems.Front Neurosci. 2016 Jul 12;10:312. doi: 10.3389/fnins.2016.00312. eCollection 2016. Front Neurosci. 2016. PMID: 27462202 Free PMC article. Review.
-
Recording single- and multi-unit neuronal action potentials from the surface of the dorsal root ganglion.Sci Rep. 2019 Feb 26;9(1):2786. doi: 10.1038/s41598-019-38924-w. Sci Rep. 2019. PMID: 30808921 Free PMC article.
-
Spatial models of cell distribution in human lumbar dorsal root ganglia.J Comp Neurol. 2020 Jul;528(10):1644-1659. doi: 10.1002/cne.24848. Epub 2020 Jan 6. J Comp Neurol. 2020. PMID: 31872433 Free PMC article.
References
-
- Aoyagi Y, Stein RB, Branner A, Pearson KG, Normann RA. Capabilities of a penetrating microelectrode array for recording single units in dorsal root ganglia of the cat. J Neurosci Methods. 2003;128:9–20. - PubMed
-
- Branner A, R A Normann. A multielectrode array for intrafascicular recording and stimulation in sciatic nerve of cats. Brain Res Bull. 2000;51:293–306. - PubMed
-
- Brown-Triolo DL, Roach MJ, Nelson K, Triolo RJ. Consumer perspectives on mobility: implications for neuroprosthesis design. J Rehabil Res Dev. 2002;39:659–69. - PubMed
Publication types
MeSH terms
Grants and funding
LinkOut - more resources
Full Text Sources
Other Literature Sources
Miscellaneous