When is hub gene selection better than standard meta-analysis?
- PMID: 23613865
- PMCID: PMC3629234
- DOI: 10.1371/journal.pone.0061505
When is hub gene selection better than standard meta-analysis?
Abstract
Since hub nodes have been found to play important roles in many networks, highly connected hub genes are expected to play an important role in biology as well. However, the empirical evidence remains ambiguous. An open question is whether (or when) hub gene selection leads to more meaningful gene lists than a standard statistical analysis based on significance testing when analyzing genomic data sets (e.g., gene expression or DNA methylation data). Here we address this question for the special case when multiple genomic data sets are available. This is of great practical importance since for many research questions multiple data sets are publicly available. In this case, the data analyst can decide between a standard statistical approach (e.g., based on meta-analysis) and a co-expression network analysis approach that selects intramodular hubs in consensus modules. We assess the performance of these two types of approaches according to two criteria. The first criterion evaluates the biological insights gained and is relevant in basic research. The second criterion evaluates the validation success (reproducibility) in independent data sets and often applies in clinical diagnostic or prognostic applications. We compare meta-analysis with consensus network analysis based on weighted correlation network analysis (WGCNA) in three comprehensive and unbiased empirical studies: (1) Finding genes predictive of lung cancer survival, (2) finding methylation markers related to age, and (3) finding mouse genes related to total cholesterol. The results demonstrate that intramodular hub gene status with respect to consensus modules is more useful than a meta-analysis p-value when identifying biologically meaningful gene lists (reflecting criterion 1). However, standard meta-analysis methods perform as good as (if not better than) a consensus network approach in terms of validation success (criterion 2). The article also reports a comparison of meta-analysis techniques applied to gene expression data and presents novel R functions for carrying out consensus network analysis, network based screening, and meta analysis.
Conflict of interest statement
Figures
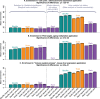


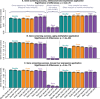
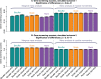
Similar articles
-
WGCNA: an R package for weighted correlation network analysis.BMC Bioinformatics. 2008 Dec 29;9:559. doi: 10.1186/1471-2105-9-559. BMC Bioinformatics. 2008. PMID: 19114008 Free PMC article.
-
VAN: an R package for identifying biologically perturbed networks via differential variability analysis.BMC Res Notes. 2013 Oct 25;6:430. doi: 10.1186/1756-0500-6-430. BMC Res Notes. 2013. PMID: 24156242 Free PMC article.
-
Weighted Gene Correlation Network Analysis (WGCNA) Detected Loss of MAGI2 Promotes Chronic Kidney Disease (CKD) by Podocyte Damage.Cell Physiol Biochem. 2018;51(1):244-261. doi: 10.1159/000495205. Epub 2018 Nov 16. Cell Physiol Biochem. 2018. PMID: 30448842
-
Exploration and validation of hub genes and pathways in the progression of hypoplastic left heart syndrome via weighted gene co-expression network analysis.BMC Cardiovasc Disord. 2021 Jun 15;21(1):300. doi: 10.1186/s12872-021-02108-0. BMC Cardiovasc Disord. 2021. PMID: 34130651 Free PMC article.
-
Identification of potential transcriptomic markers in developing pediatric sepsis: a weighted gene co-expression network analysis and a case-control validation study.J Transl Med. 2017 Dec 13;15(1):254. doi: 10.1186/s12967-017-1364-8. J Transl Med. 2017. PMID: 29237456 Free PMC article.
Cited by
-
An Integrative Transcriptomic Analysis for Identifying Novel Target Genes Corresponding to Severity Spectrum in Spinal Muscular Atrophy.PLoS One. 2016 Jun 22;11(6):e0157426. doi: 10.1371/journal.pone.0157426. eCollection 2016. PLoS One. 2016. PMID: 27331400 Free PMC article.
-
Searching for convergent pathways in autism spectrum disorders: insights from human brain transcriptome studies.Cell Mol Life Sci. 2016 Dec;73(23):4517-4530. doi: 10.1007/s00018-016-2304-0. Epub 2016 Jul 12. Cell Mol Life Sci. 2016. PMID: 27405608 Free PMC article. Review.
-
Construction and analysis of gene co-expression network in the pathogenic fungus Ustilago maydis.Front Microbiol. 2022 Dec 7;13:1048694. doi: 10.3389/fmicb.2022.1048694. eCollection 2022. Front Microbiol. 2022. PMID: 36569046 Free PMC article.
-
Gene coexpression network analysis combined with metabonomics reveals the resistance responses to powdery mildew in Tibetan hulless barley.Sci Rep. 2018 Oct 8;8(1):14928. doi: 10.1038/s41598-018-33113-7. Sci Rep. 2018. PMID: 30297768 Free PMC article.
-
Integrating gene regulatory pathways into differential network analysis of gene expression data.Sci Rep. 2019 Apr 2;9(1):5479. doi: 10.1038/s41598-019-41918-3. Sci Rep. 2019. PMID: 30940863 Free PMC article.
References
-
- Stuart JM, Segal E, Koller D, Kim SK (2003) A Gene-Coexpression Network for Global Discovery of Conserved Genetic Modules. Science 302: 249–255. - PubMed
-
- Zhang B, Horvath S (2005) General framework for weighted gene coexpression analysis. Statistical Applications in Genetics and Molecular Biology 4. - PubMed
-
- Huang Y, Li H, Hu H, Yan X, Waterman M, et al. (2007) Systematic discovery of functional modules and context-specific functional annotation of human genome. Bioinformatics 23: i222–229. - PubMed
Publication types
MeSH terms
Grants and funding
LinkOut - more resources
Full Text Sources
Other Literature Sources