Single-cell and subcellular pharmacokinetic imaging allows insight into drug action in vivo
- PMID: 23422672
- PMCID: PMC3579506
- DOI: 10.1038/ncomms2506
Single-cell and subcellular pharmacokinetic imaging allows insight into drug action in vivo
Abstract
Pharmacokinetic analysis at the organ level provides insight into how drugs distribute throughout the body, but cannot explain how drugs work at the cellular level. Here we demonstrate in vivo single-cell pharmacokinetic imaging of PARP-1 inhibitors and model drug behaviour under varying conditions. We visualize intracellular kinetics of the PARP-1 inhibitor distribution in real time, showing that PARP-1 inhibitors reach their cellular target compartment, the nucleus, within minutes in vivo both in cancer and normal cells in various cancer models. We also use these data to validate predictive finite element modelling. Our theoretical and experimental data indicate that tumour cells are exposed to sufficiently high PARP-1 inhibitor concentrations in vivo and suggest that drug inefficiency is likely related to proteomic heterogeneity or insensitivity of cancer cells to DNA-repair inhibition. This suggests that single-cell pharmacokinetic imaging and derived modelling improve our understanding of drug action at single-cell resolution in vivo.
Figures
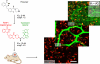
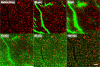
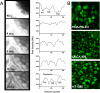
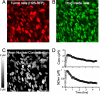
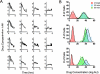
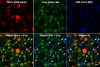
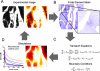
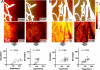
Similar articles
-
Effect of small-molecule modification on single-cell pharmacokinetics of PARP inhibitors.Mol Cancer Ther. 2014 Apr;13(4):986-95. doi: 10.1158/1535-7163.MCT-13-0801. Epub 2014 Feb 19. Mol Cancer Ther. 2014. PMID: 24552776 Free PMC article.
-
Multi-targeted organometallic ruthenium(II)-arene anticancer complexes bearing inhibitors of poly(ADP-ribose) polymerase-1: A strategy to improve cytotoxicity.J Inorg Biochem. 2014 Feb;131:47-55. doi: 10.1016/j.jinorgbio.2013.10.017. Epub 2013 Oct 31. J Inorg Biochem. 2014. PMID: 24239912
-
Poly (ADP-Ribose) Polymerases (PARPs) and PARP Inhibitor-Targeted Therapeutics.Anticancer Agents Med Chem. 2019;19(2):206-212. doi: 10.2174/1871520618666181109164645. Anticancer Agents Med Chem. 2019. PMID: 30417796 Review.
-
A novel and potent poly(ADP-ribose) polymerase-1 inhibitor, FR247304 (5-chloro-2-[3-(4-phenyl-3,6-dihydro-1(2H)-pyridinyl)propyl]-4(3H)-quinazolinone), attenuates neuronal damage in in vitro and in vivo models of cerebral ischemia.J Pharmacol Exp Ther. 2004 Aug;310(2):425-36. doi: 10.1124/jpet.104.066944. Epub 2004 Apr 9. J Pharmacol Exp Ther. 2004. PMID: 15075382
-
Poly(ADP-ribose) polymerase activity and inhibition in cancer.Semin Cell Dev Biol. 2017 Mar;63:144-153. doi: 10.1016/j.semcdb.2017.01.007. Epub 2017 Jan 10. Semin Cell Dev Biol. 2017. PMID: 28087320 Review.
Cited by
-
Real-time high dynamic range laser scanning microscopy.Nat Commun. 2016 Apr 1;7:11077. doi: 10.1038/ncomms11077. Nat Commun. 2016. PMID: 27032979 Free PMC article.
-
Mathematical Based Calculation of Drug Penetration Depth in Solid Tumors.Biomed Res Int. 2016;2016:8437247. doi: 10.1155/2016/8437247. Epub 2016 Jun 8. Biomed Res Int. 2016. PMID: 27376087 Free PMC article.
-
Spatial analysis of drug absorption, distribution, metabolism, and toxicology using mass spectrometry imaging.Biochem Pharmacol. 2022 Jul;201:115080. doi: 10.1016/j.bcp.2022.115080. Epub 2022 May 10. Biochem Pharmacol. 2022. PMID: 35561842 Free PMC article. Review.
-
Micropharmacology: An In Silico Approach for Assessing Drug Efficacy Within a Tumor Tissue.Bull Math Biol. 2019 Sep;81(9):3623-3641. doi: 10.1007/s11538-018-0402-x. Epub 2018 Feb 8. Bull Math Biol. 2019. PMID: 29423880 Free PMC article.
-
Multichannel imaging to quantify four classes of pharmacokinetic distribution in tumors.J Pharm Sci. 2014 Oct;103(10):3276-86. doi: 10.1002/jps.24086. Epub 2014 Jul 21. J Pharm Sci. 2014. PMID: 25048378 Free PMC article.
References
-
- Sun H, et al. Population pharmacokinetics. A regulatory perspective. Clin Pharmacokinet. 1999;37:41–58. - PubMed
Publication types
MeSH terms
Substances
Associated data
Grants and funding
LinkOut - more resources
Full Text Sources
Other Literature Sources
Miscellaneous