Sensitive detection of somatic point mutations in impure and heterogeneous cancer samples
- PMID: 23396013
- PMCID: PMC3833702
- DOI: 10.1038/nbt.2514
Sensitive detection of somatic point mutations in impure and heterogeneous cancer samples
Abstract
Detection of somatic point substitutions is a key step in characterizing the cancer genome. However, existing methods typically miss low-allelic-fraction mutations that occur in only a subset of the sequenced cells owing to either tumor heterogeneity or contamination by normal cells. Here we present MuTect, a method that applies a Bayesian classifier to detect somatic mutations with very low allele fractions, requiring only a few supporting reads, followed by carefully tuned filters that ensure high specificity. We also describe benchmarking approaches that use real, rather than simulated, sequencing data to evaluate the sensitivity and specificity as a function of sequencing depth, base quality and allelic fraction. Compared with other methods, MuTect has higher sensitivity with similar specificity, especially for mutations with allelic fractions as low as 0.1 and below, making MuTect particularly useful for studying cancer subclones and their evolution in standard exome and genome sequencing data.
Figures
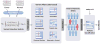
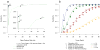
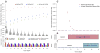
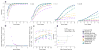
Comment in
-
Bioinformatics: Tumors have their differences.Nat Methods. 2013 Apr;10(4):282-3. doi: 10.1038/nmeth.2426. Nat Methods. 2013. PMID: 23653923 No abstract available.
Similar articles
-
Optimized pipeline of MuTect and GATK tools to improve the detection of somatic single nucleotide polymorphisms in whole-exome sequencing data.BMC Bioinformatics. 2016 Nov 8;17(Suppl 12):341. doi: 10.1186/s12859-016-1190-7. BMC Bioinformatics. 2016. PMID: 28185561 Free PMC article.
-
SiNVICT: ultra-sensitive detection of single nucleotide variants and indels in circulating tumour DNA.Bioinformatics. 2017 Jan 1;33(1):26-34. doi: 10.1093/bioinformatics/btw536. Epub 2016 Aug 16. Bioinformatics. 2017. PMID: 27531099
-
Integrated approach to generate artificial samples with low tumor fraction for somatic variant calling benchmarking.BMC Bioinformatics. 2024 May 8;25(1):180. doi: 10.1186/s12859-024-05793-8. BMC Bioinformatics. 2024. PMID: 38720249 Free PMC article.
-
Targeted deep resequencing of the human cancer genome using next-generation technologies.Biotechnol Genet Eng Rev. 2010;27:135-58. doi: 10.1080/02648725.2010.10648148. Biotechnol Genet Eng Rev. 2010. PMID: 21415896 Free PMC article. Review.
-
Computational approaches for discovery of mutational signatures in cancer.Brief Bioinform. 2019 Jan 18;20(1):77-88. doi: 10.1093/bib/bbx082. Brief Bioinform. 2019. PMID: 28968631 Free PMC article. Review.
Cited by
-
Proteogenomic analysis dissects early-onset breast cancer patients with prognostic relevance.Exp Mol Med. 2024 Nov;56(11):2382-2394. doi: 10.1038/s12276-024-01332-w. Epub 2024 Nov 1. Exp Mol Med. 2024. PMID: 39482530 Free PMC article.
-
CCND1 Amplification Contributes to Immunosuppression and Is Associated With a Poor Prognosis to Immune Checkpoint Inhibitors in Solid Tumors.Front Immunol. 2020 Aug 10;11:1620. doi: 10.3389/fimmu.2020.01620. eCollection 2020. Front Immunol. 2020. PMID: 32903763 Free PMC article.
-
Ganciclovir-induced mutations are present in a diverse spectrum of post-transplant malignancies.Genome Med. 2022 Oct 31;14(1):124. doi: 10.1186/s13073-022-01131-w. Genome Med. 2022. PMID: 36316687 Free PMC article.
-
Whole-exome sequencing of Nigerian benign prostatic hyperplasia reveals increased alterations in apoptotic pathways.Prostate. 2024 Apr;84(5):460-472. doi: 10.1002/pros.24662. Epub 2024 Jan 8. Prostate. 2024. PMID: 38192023
-
Clonal relationships between lobular carcinoma in situ and other breast malignancies.Breast Cancer Res. 2016 Jun 23;18(1):66. doi: 10.1186/s13058-016-0727-z. Breast Cancer Res. 2016. PMID: 27334989 Free PMC article.
References
Publication types
MeSH terms
Substances
Grants and funding
LinkOut - more resources
Full Text Sources
Other Literature Sources