Evaluation of methods for modeling transcription factor sequence specificity
- PMID: 23354101
- PMCID: PMC3687085
- DOI: 10.1038/nbt.2486
Evaluation of methods for modeling transcription factor sequence specificity
Abstract
Genomic analyses often involve scanning for potential transcription factor (TF) binding sites using models of the sequence specificity of DNA binding proteins. Many approaches have been developed to model and learn a protein's DNA-binding specificity, but these methods have not been systematically compared. Here we applied 26 such approaches to in vitro protein binding microarray data for 66 mouse TFs belonging to various families. For nine TFs, we also scored the resulting motif models on in vivo data, and found that the best in vitro-derived motifs performed similarly to motifs derived from the in vivo data. Our results indicate that simple models based on mononucleotide position weight matrices trained by the best methods perform similarly to more complex models for most TFs examined, but fall short in specific cases (<10% of the TFs examined here). In addition, the best-performing motifs typically have relatively low information content, consistent with widespread degeneracy in eukaryotic TF sequence preferences.
Figures
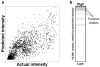
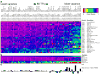
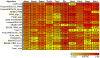
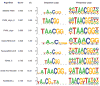
Similar articles
-
Optimally choosing PWM motif databases and sequence scanning approaches based on ChIP-seq data.BMC Bioinformatics. 2015 May 1;16:140. doi: 10.1186/s12859-015-0573-5. BMC Bioinformatics. 2015. PMID: 25927199 Free PMC article.
-
High resolution models of transcription factor-DNA affinities improve in vitro and in vivo binding predictions.PLoS Comput Biol. 2010 Sep 9;6(9):e1000916. doi: 10.1371/journal.pcbi.1000916. PLoS Comput Biol. 2010. PMID: 20838582 Free PMC article.
-
Nonconsensus Protein Binding to Repetitive DNA Sequence Elements Significantly Affects Eukaryotic Genomes.PLoS Comput Biol. 2015 Aug 18;11(8):e1004429. doi: 10.1371/journal.pcbi.1004429. eCollection 2015 Aug. PLoS Comput Biol. 2015. PMID: 26285121 Free PMC article.
-
Transcription factor-DNA binding: beyond binding site motifs.Curr Opin Genet Dev. 2017 Apr;43:110-119. doi: 10.1016/j.gde.2017.02.007. Epub 2017 Mar 27. Curr Opin Genet Dev. 2017. PMID: 28359978 Free PMC article. Review.
-
DNA Motif Databases and Their Uses.Curr Protoc Bioinformatics. 2015 Sep 3;51:2.15.1-2.15.6. doi: 10.1002/0471250953.bi0215s51. Curr Protoc Bioinformatics. 2015. PMID: 26334922 Review.
Cited by
-
Machine learning and genome annotation: a match meant to be?Genome Biol. 2013 May 29;14(5):205. doi: 10.1186/gb-2013-14-5-205. Genome Biol. 2013. PMID: 23731483 Free PMC article. Review.
-
SeqGL Identifies Context-Dependent Binding Signals in Genome-Wide Regulatory Element Maps.PLoS Comput Biol. 2015 May 27;11(5):e1004271. doi: 10.1371/journal.pcbi.1004271. eCollection 2015 May. PLoS Comput Biol. 2015. PMID: 26016777 Free PMC article.
-
Spatial distribution of predicted transcription factor binding sites in Drosophila ChIP peaks.Mech Dev. 2016 Aug;141:51-61. doi: 10.1016/j.mod.2016.06.001. Epub 2016 Jun 2. Mech Dev. 2016. PMID: 27264535 Free PMC article.
-
Bayesian Markov models consistently outperform PWMs at predicting motifs in nucleotide sequences.Nucleic Acids Res. 2016 Jul 27;44(13):6055-69. doi: 10.1093/nar/gkw521. Epub 2016 Jun 9. Nucleic Acids Res. 2016. PMID: 27288444 Free PMC article.
-
Position Weight Matrix or Acyclic Probabilistic Finite Automaton: Which model to use? A decision rule inferred for the prediction of transcription factor binding sites.Genet Mol Biol. 2024 Jan 19;46(4):e20230048. doi: 10.1590/1678-4685-GMB-2023-0048. eCollection 2024. Genet Mol Biol. 2024. PMID: 38285430 Free PMC article.
References
-
- Berg OG, von Hippel PH. Selection of DNA binding sites by regulatory proteins. Statistical-mechanical theory and application to operators and promoters. J Mol Biol. 1987;193:723–750. - PubMed
-
- Stormo GD. Consensus patterns in DNA. Methods Enzymol. 1990;183:211–221. - PubMed
-
- Zhao X, Huang H, Speed TP. Finding short DNA motifs using permuted Markov models. J Comput Biol. 2005;12:894–906. - PubMed
Publication types
MeSH terms
Substances
Associated data
- Actions
Grants and funding
LinkOut - more resources
Full Text Sources
Other Literature Sources
Molecular Biology Databases
Miscellaneous