Structure-based prediction of protein-protein interactions on a genome-wide scale
- PMID: 23023127
- PMCID: PMC3482288
- DOI: 10.1038/nature11503
Structure-based prediction of protein-protein interactions on a genome-wide scale
Erratum in
- Nature. 2013 Mar 7;495(7439):127
Abstract
The genome-wide identification of pairs of interacting proteins is an important step in the elucidation of cell regulatory mechanisms. Much of our present knowledge derives from high-throughput techniques such as the yeast two-hybrid assay and affinity purification, as well as from manual curation of experiments on individual systems. A variety of computational approaches based, for example, on sequence homology, gene co-expression and phylogenetic profiles, have also been developed for the genome-wide inference of protein-protein interactions (PPIs). Yet comparative studies suggest that the development of accurate and complete repertoires of PPIs is still in its early stages. Here we show that three-dimensional structural information can be used to predict PPIs with an accuracy and coverage that are superior to predictions based on non-structural evidence. Moreover, an algorithm, termed PrePPI, which combines structural information with other functional clues, is comparable in accuracy to high-throughput experiments, yielding over 30,000 high-confidence interactions for yeast and over 300,000 for human. Experimental tests of a number of predictions demonstrate the ability of the PrePPI algorithm to identify unexpected PPIs of considerable biological interest. The surprising effectiveness of three-dimensional structural information can be attributed to the use of homology models combined with the exploitation of both close and remote geometric relationships between proteins.
Conflict of interest statement
Reprints and permissions information is available at
Figures
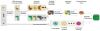
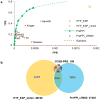
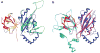
Comment in
-
Predicting PPIs.Nat Methods. 2012 Dec;9(12):1139. doi: 10.1038/nmeth.2272. Nat Methods. 2012. PMID: 23355983 No abstract available.
Similar articles
-
Toward a "structural BLAST": using structural relationships to infer function.Protein Sci. 2013 Apr;22(4):359-66. doi: 10.1002/pro.2225. Epub 2013 Feb 21. Protein Sci. 2013. PMID: 23349097 Free PMC article. Review.
-
PIPE: a protein-protein interaction prediction engine based on the re-occurring short polypeptide sequences between known interacting protein pairs.BMC Bioinformatics. 2006 Jul 27;7:365. doi: 10.1186/1471-2105-7-365. BMC Bioinformatics. 2006. PMID: 16872538 Free PMC article.
-
Heterogeneous data integration by tree-augmented naïve Bayes for protein-protein interactions prediction.Proteomics. 2013 Jan;13(2):261-8. doi: 10.1002/pmic.201200326. Epub 2012 Dec 3. Proteomics. 2013. PMID: 23112070
-
A Bayesian approach for estimating protein-protein interactions by integrating structural and non-structural biological data.Mol Biosyst. 2017 Nov 21;13(12):2592-2602. doi: 10.1039/c7mb00484b. Mol Biosyst. 2017. PMID: 29028065
-
Protein-fragment complementation assays for large-scale analysis of protein-protein interactions.Biochem Soc Trans. 2021 Jun 30;49(3):1337-1348. doi: 10.1042/BST20201058. Biochem Soc Trans. 2021. PMID: 34156434 Free PMC article. Review.
Cited by
-
Molecular determinants within the C-termini of L-HDAg that regulate hepatitis D virus replication and assembly.JHEP Rep. 2023 Nov 15;6(1):100961. doi: 10.1016/j.jhepr.2023.100961. eCollection 2024 Jan. JHEP Rep. 2023. PMID: 38192534 Free PMC article.
-
An expanded universe of cancer targets.Cell. 2021 Mar 4;184(5):1142-1155. doi: 10.1016/j.cell.2021.02.020. Cell. 2021. PMID: 33667368 Free PMC article. Review.
-
Using prior knowledge from cellular pathways and molecular networks for diagnostic specimen classification.Brief Bioinform. 2016 May;17(3):440-52. doi: 10.1093/bib/bbv044. Epub 2015 Jul 2. Brief Bioinform. 2016. PMID: 26141830 Free PMC article.
-
Template-based prediction of protein function.Curr Opin Struct Biol. 2015 Jun;32:33-8. doi: 10.1016/j.sbi.2015.01.007. Epub 2015 Feb 10. Curr Opin Struct Biol. 2015. PMID: 25678152 Free PMC article. Review.
-
Advances in the Application of Protein Language Modeling for Nucleic Acid Protein Binding Site Prediction.Genes (Basel). 2024 Aug 18;15(8):1090. doi: 10.3390/genes15081090. Genes (Basel). 2024. PMID: 39202449 Free PMC article.
References
-
- Bonetta L. Protein-protein interactions: Interactome under construction. Nature. 2010;468:851–854. - PubMed
Publication types
MeSH terms
Substances
Grants and funding
- R01NS043915/NS/NINDS NIH HHS/United States
- DK057539/DK/NIDDK NIH HHS/United States
- CA082683/CA/NCI NIH HHS/United States
- CA121852/CA/NCI NIH HHS/United States
- GM094597/GM/NIGMS NIH HHS/United States
- U54 CA121852/CA/NCI NIH HHS/United States
- R01 DK057539/DK/NIDDK NIH HHS/United States
- R37 GM030518/GM/NIGMS NIH HHS/United States
- R01 CA082683/CA/NCI NIH HHS/United States
- R01 NS043915/NS/NINDS NIH HHS/United States
- HHMI/Howard Hughes Medical Institute/United States
- R01 GM030518/GM/NIGMS NIH HHS/United States
- U54 GM094597/GM/NIGMS NIH HHS/United States
- GM030518/GM/NIGMS NIH HHS/United States
LinkOut - more resources
Full Text Sources
Other Literature Sources