Sequence and chromatin determinants of cell-type-specific transcription factor binding
- PMID: 22955984
- PMCID: PMC3431489
- DOI: 10.1101/gr.127712.111
Sequence and chromatin determinants of cell-type-specific transcription factor binding
Abstract
Gene regulatory programs in distinct cell types are maintained in large part through the cell-type-specific binding of transcription factors (TFs). The determinants of TF binding include direct DNA sequence preferences, DNA sequence preferences of cofactors, and the local cell-dependent chromatin context. To explore the contribution of DNA sequence signal, histone modifications, and DNase accessibility to cell-type-specific binding, we analyzed 286 ChIP-seq experiments performed by the ENCODE Consortium. This analysis included experiments for 67 transcriptional regulators, 15 of which were profiled in both the GM12878 (lymphoblastoid) and K562 (erythroleukemic) human hematopoietic cell lines. To model TF-bound regions, we trained support vector machines (SVMs) that use flexible k-mer patterns to capture DNA sequence signals more accurately than traditional motif approaches. In addition, we trained SVM spatial chromatin signatures to model local histone modifications and DNase accessibility, obtaining significantly more accurate TF occupancy predictions than simpler approaches. Consistent with previous studies, we find that DNase accessibility can explain cell-line-specific binding for many factors. However, we also find that of the 10 factors with prominent cell-type-specific binding patterns, four display distinct cell-type-specific DNA sequence preferences according to our models. Moreover, for two factors we identify cell-specific binding sites that are accessible in both cell types but bound only in one. For these sites, cell-type-specific sequence models, rather than DNase accessibility, are better able to explain differential binding. Our results suggest that using a single motif for each TF and filtering for chromatin accessible loci is not always sufficient to accurately account for cell-type-specific binding profiles.
Figures
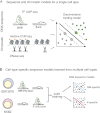
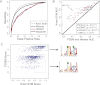
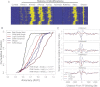
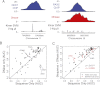
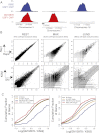
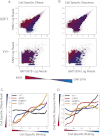
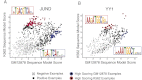
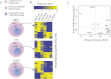
Similar articles
-
Sequence features and chromatin structure around the genomic regions bound by 119 human transcription factors.Genome Res. 2012 Sep;22(9):1798-812. doi: 10.1101/gr.139105.112. Genome Res. 2012. PMID: 22955990 Free PMC article.
-
SeqGL Identifies Context-Dependent Binding Signals in Genome-Wide Regulatory Element Maps.PLoS Comput Biol. 2015 May 27;11(5):e1004271. doi: 10.1371/journal.pcbi.1004271. eCollection 2015 May. PLoS Comput Biol. 2015. PMID: 26016777 Free PMC article.
-
BinDNase: a discriminatory approach for transcription factor binding prediction using DNase I hypersensitivity data.Bioinformatics. 2015 Sep 1;31(17):2852-9. doi: 10.1093/bioinformatics/btv294. Epub 2015 May 7. Bioinformatics. 2015. PMID: 25957350
-
Mechanisms by which transcription factors gain access to target sequence elements in chromatin.Curr Opin Genet Dev. 2013 Apr;23(2):116-23. doi: 10.1016/j.gde.2012.11.008. Epub 2012 Dec 19. Curr Opin Genet Dev. 2013. PMID: 23266217 Free PMC article. Review.
-
Role of ChIP-seq in the discovery of transcription factor binding sites, differential gene regulation mechanism, epigenetic marks and beyond.Cell Cycle. 2014;13(18):2847-52. doi: 10.4161/15384101.2014.949201. Cell Cycle. 2014. PMID: 25486472 Free PMC article. Review.
Cited by
-
Epigenetic Regulation of Ameloblast Differentiation by HMGN Proteins.J Dent Res. 2024 Jan;103(1):51-61. doi: 10.1177/00220345231202468. Epub 2023 Nov 10. J Dent Res. 2024. PMID: 37950483 Free PMC article.
-
Inflammation-induced repression of chromatin bound by the transcription factor Foxp3 in regulatory T cells.Nat Immunol. 2014 Jun;15(6):580-587. doi: 10.1038/ni.2868. Epub 2014 Apr 13. Nat Immunol. 2014. PMID: 24728351 Free PMC article.
-
Divergence in DNA Specificity among Paralogous Transcription Factors Contributes to Their Differential In Vivo Binding.Cell Syst. 2018 Apr 25;6(4):470-483.e8. doi: 10.1016/j.cels.2018.02.009. Epub 2018 Mar 28. Cell Syst. 2018. PMID: 29605182 Free PMC article.
-
Transcription factor binding predicts histone modifications in human cell lines.Proc Natl Acad Sci U S A. 2014 Sep 16;111(37):13367-72. doi: 10.1073/pnas.1412081111. Epub 2014 Sep 3. Proc Natl Acad Sci U S A. 2014. PMID: 25187560 Free PMC article.
-
Molecular features of cellular reprogramming and development.Nat Rev Mol Cell Biol. 2016 Mar;17(3):139-54. doi: 10.1038/nrm.2016.6. Epub 2016 Feb 17. Nat Rev Mol Cell Biol. 2016. PMID: 26883001 Review.
References
Publication types
MeSH terms
Substances
Grants and funding
LinkOut - more resources
Full Text Sources
Miscellaneous