New tools for classification and monitoring of autoimmune diseases
- PMID: 22647780
- PMCID: PMC3409841
- DOI: 10.1038/nrrheum.2012.66
New tools for classification and monitoring of autoimmune diseases
Erratum in
- Nat Rev Rheumatol. 2012 Oct;8(10):562
Abstract
Rheumatologists see patients with a range of autoimmune diseases. Phenotyping these diseases for diagnosis, prognosis and selection of therapies is an ever increasing problem. Advances in multiplexed assay technology at the gene, protein, and cellular level have enabled the identification of 'actionable biomarkers'; that is, biological metrics that can inform clinical practice. Not only will such biomarkers yield insight into the development, remission, and exacerbation of a disease, they will undoubtedly improve diagnostic sensitivity and accuracy of classification, and ultimately guide treatment. This Review provides an introduction to these powerful technologies that could promote the identification of actionable biomarkers, including mass cytometry, protein arrays, and immunoglobulin and T-cell receptor high-throughput sequencing. In our opinion, these technologies should become part of routine clinical practice for the management of autoimmune diseases. The use of analytical tools to deconvolve the data obtained from use of these technologies is also presented here. These analyses are revealing a more comprehensive and interconnected view of the immune system than ever before and should have an important role in directing future treatment approaches for autoimmune diseases.
Figures
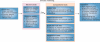
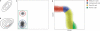
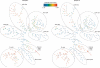
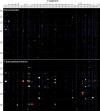
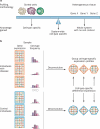
Similar articles
-
New technologies for autoimmune disease monitoring.Curr Opin Endocrinol Diabetes Obes. 2010 Aug;17(4):322-8. doi: 10.1097/MED.0b013e32833ada91. Curr Opin Endocrinol Diabetes Obes. 2010. PMID: 20531181 Free PMC article. Review.
-
Immunophenotyping As a New Tool for Classification and Monitoring of Systemic Autoimmune Diseases.Clin Rev Allergy Immunol. 2017 Oct;53(2):177-180. doi: 10.1007/s12016-017-8604-9. Clin Rev Allergy Immunol. 2017. PMID: 28477077
-
Advances and applications of multiplexed diagnostic technologies in autoimmune diseases.Lupus. 2006;15(7):422-7. doi: 10.1191/0961203306lu2327oa. Lupus. 2006. PMID: 16898176 Review.
-
Technology insight: can autoantibody profiling improve clinical practice?Nat Clin Pract Rheumatol. 2007 Feb;3(2):96-103. doi: 10.1038/ncprheum0404. Nat Clin Pract Rheumatol. 2007. PMID: 17299447 Review.
-
Multiplexed protein array platforms for analysis of autoimmune diseases.Annu Rev Immunol. 2006;24:391-418. doi: 10.1146/annurev.immunol.24.021605.090709. Annu Rev Immunol. 2006. PMID: 16551254 Review.
Cited by
-
Annexin A2 as a target endothelial cell membrane autoantigen in Behçet's disease.Sci Rep. 2015 Feb 2;5:8162. doi: 10.1038/srep08162. Sci Rep. 2015. PMID: 25641213 Free PMC article.
-
Autologous Hematopoietic Stem Cell Transplantation for Autoimmune Diseases: From Mechanistic Insights to Biomarkers.Front Immunol. 2018 Nov 16;9:2602. doi: 10.3389/fimmu.2018.02602. eCollection 2018. Front Immunol. 2018. PMID: 30505303 Free PMC article. Review.
-
The promise and challenge of high-throughput sequencing of the antibody repertoire.Nat Biotechnol. 2014 Feb;32(2):158-68. doi: 10.1038/nbt.2782. Epub 2014 Jan 19. Nat Biotechnol. 2014. PMID: 24441474 Free PMC article. Review.
-
Unmet needs in autoimmunity and potential new tools.Clin Rev Allergy Immunol. 2014 Oct;47(2):111-8. doi: 10.1007/s12016-014-8414-2. Clin Rev Allergy Immunol. 2014. PMID: 24595881 Review.
-
Profiling of the TCRβ repertoire in non-model species using high-throughput sequencing.Sci Rep. 2018 Aug 2;8(1):11613. doi: 10.1038/s41598-018-30037-0. Sci Rep. 2018. PMID: 30072736 Free PMC article.
References
-
- Capdeville R, Buchdunger E, Zimmermann J, Matter A. Glivec (STI571, imatinib), a rationally developed, targeted anticancer drug. Nat. Rev. Drug Discov. 2002;1:493–502. - PubMed
-
- Nahta R, Esteva FJ. HER-2-targeted therapy: lessons learned and future directions. Clin. Cancer Res. 2003;9:5078–5084. - PubMed
-
- LaGasse JM, et al. Successful prospective prediction of type 1 diabetes in schoolchildren through multiple defined autoantibodies: an 8-year follow-up of the Washington State Diabetes Prediction Study. Diabetes Care. 2002;25:505–511. - PubMed
-
- van der Woude D, et al. The ACPA isotype profile reflects long-term radiographic progression in rheumatoid arthritis. Ann. Rheum. Dis. 2010;69:1110–1116. - PubMed
-
- Zethelius B, et al. Use of multiple biomarkers to improve the prediction of death from cardiovascular causes. N. Engl. J. Med. 2008;358:2107–2116. - PubMed
Publication types
MeSH terms
Substances
Grants and funding
LinkOut - more resources
Full Text Sources
Other Literature Sources
Medical