Essential gene profiles in breast, pancreatic, and ovarian cancer cells
- PMID: 22585861
- PMCID: PMC5057396
- DOI: 10.1158/2159-8290.CD-11-0224
Essential gene profiles in breast, pancreatic, and ovarian cancer cells
Abstract
Genomic analyses are yielding a host of new information on the multiple genetic abnormalities associated with specific types of cancer. A comprehensive description of cancer-associated genetic abnormalities can improve our ability to classify tumors into clinically relevant subgroups and, on occasion, identify mutant genes that drive the cancer phenotype ("drivers"). More often, though, the functional significance of cancer-associated mutations is difficult to discern. Genome-wide pooled short hairpin RNA (shRNA) screens enable global identification of the genes essential for cancer cell survival and proliferation, providing a "functional genomic" map of human cancer to complement genomic studies. Using a lentiviral shRNA library targeting ~16,000 genes and a newly developed, dynamic scoring approach, we identified essential gene profiles in 72 breast, pancreatic, and ovarian cancer cell lines. Integrating our results with current and future genomic data should facilitate the systematic identification of drivers, unanticipated synthetic lethal relationships, and functional vulnerabilities of these tumor types.
Significance: This study presents a resource of genome-scale, pooled shRNA screens for 72 breast, pancreatic, and ovarian cancer cell lines that will serve as a functional complement to genomics data, facilitate construction of essential gene profiles, help uncover synthetic lethal relationships, and identify uncharacterized genetic vulnerabilities in these tumor types.
Significance: This study presents a resource of genome-scale, pooled shRNA screens for 72 breast, pancreatic, and ovarian cancer cell lines that will serve as a functional complement to genomics data, facilitate construction of essential gene profiles, help uncover synthetic lethal relationships, and identify uncharacterized genetic vulnerabilities in these tumor types.
©2011 AACR.
Figures
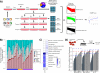
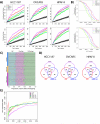
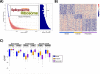
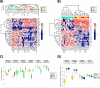
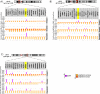
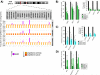
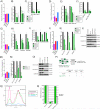
Similar articles
-
A negative genetic interaction map in isogenic cancer cell lines reveals cancer cell vulnerabilities.Mol Syst Biol. 2013 Oct 8;9:696. doi: 10.1038/msb.2013.54. Mol Syst Biol. 2013. PMID: 24104479 Free PMC article.
-
Integrative genomic and functional profiling of the pancreatic cancer genome.BMC Genomics. 2013 Sep 16;14:624. doi: 10.1186/1471-2164-14-624. BMC Genomics. 2013. PMID: 24041470 Free PMC article.
-
COLT-Cancer: functional genetic screening resource for essential genes in human cancer cell lines.Nucleic Acids Res. 2012 Jan;40(Database issue):D957-63. doi: 10.1093/nar/gkr959. Epub 2011 Nov 18. Nucleic Acids Res. 2012. PMID: 22102578 Free PMC article.
-
More than fishing for a cure: The promises and pitfalls of high throughput cancer cell line screens.Pharmacol Ther. 2018 Nov;191:178-189. doi: 10.1016/j.pharmthera.2018.06.014. Epub 2018 Jun 25. Pharmacol Ther. 2018. PMID: 29953899 Free PMC article. Review.
-
A primer on using pooled shRNA libraries for functional genomic screens.Acta Biochim Biophys Sin (Shanghai). 2012 Feb;44(2):103-12. doi: 10.1093/abbs/gmr116. Acta Biochim Biophys Sin (Shanghai). 2012. PMID: 22271906 Free PMC article. Review.
Cited by
-
Cancer vulnerabilities unveiled by genomic loss.Cell. 2012 Aug 17;150(4):842-54. doi: 10.1016/j.cell.2012.07.023. Cell. 2012. PMID: 22901813 Free PMC article.
-
CX-5461 Enhances the Efficacy of APR-246 via Induction of DNA Damage and Replication Stress in Triple-Negative Breast Cancer.Int J Mol Sci. 2021 May 28;22(11):5782. doi: 10.3390/ijms22115782. Int J Mol Sci. 2021. PMID: 34071360 Free PMC article.
-
Transcriptional Factor Repertoire of Breast Cancer in 3D Cell Culture Models.Cancers (Basel). 2022 Feb 17;14(4):1023. doi: 10.3390/cancers14041023. Cancers (Basel). 2022. PMID: 35205770 Free PMC article. Review.
-
Discoidin Domain Receptor-1 (DDR1) is Involved in Angiolymphatic Invasion in Oral Cancer.Cancers (Basel). 2020 Mar 31;12(4):841. doi: 10.3390/cancers12040841. Cancers (Basel). 2020. PMID: 32244515 Free PMC article.
-
Gene Essentiality Profiling Reveals Gene Networks and Synthetic Lethal Interactions with Oncogenic Ras.Cell. 2017 Feb 23;168(5):890-903.e15. doi: 10.1016/j.cell.2017.01.013. Epub 2017 Feb 2. Cell. 2017. PMID: 28162770 Free PMC article.
References
-
- Chin K, DeVries S, Fridlyand J, Spellman PT, Roydasgupta R, Kuo WL, et al. Genomic and transcriptional aberrations linked to breast cancer pathophysiologies. Cancer Cell. 2006;10:529–41. - PubMed
Publication types
MeSH terms
Substances
Grants and funding
LinkOut - more resources
Full Text Sources
Other Literature Sources
Medical
Research Materials