Copy number variation detection in whole-genome sequencing data using the Bayesian information criterion
- PMID: 22065754
- PMCID: PMC3219132
- DOI: 10.1073/pnas.1110574108
Copy number variation detection in whole-genome sequencing data using the Bayesian information criterion
Abstract
DNA copy number variations (CNVs) play an important role in the pathogenesis and progression of cancer and confer susceptibility to a variety of human disorders. Array comparative genomic hybridization has been used widely to identify CNVs genome wide, but the next-generation sequencing technology provides an opportunity to characterize CNVs genome wide with unprecedented resolution. In this study, we developed an algorithm to detect CNVs from whole-genome sequencing data and applied it to a newly sequenced glioblastoma genome with a matched control. This read-depth algorithm, called BIC-seq, can accurately and efficiently identify CNVs via minimizing the Bayesian information criterion. Using BIC-seq, we identified hundreds of CNVs as small as 40 bp in the cancer genome sequenced at 10× coverage, whereas we could only detect large CNVs (> 15 kb) in the array comparative genomic hybridization profiles for the same genome. Eighty percent (14/16) of the small variants tested (110 bp to 14 kb) were experimentally validated by quantitative PCR, demonstrating high sensitivity and true positive rate of the algorithm. We also extended the algorithm to detect recurrent CNVs in multiple samples as well as deriving error bars for breakpoints using a Gibbs sampling approach. We propose this statistical approach as a principled yet practical and efficient method to estimate CNVs in whole-genome sequencing data.
Conflict of interest statement
The authors declare no conflict of interest.
Figures
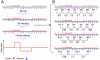
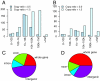
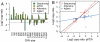
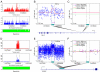
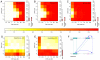
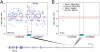
Similar articles
-
Detection of copy number variation from array intensity and sequencing read depth using a stepwise Bayesian model.BMC Bioinformatics. 2010 Oct 31;11:539. doi: 10.1186/1471-2105-11-539. BMC Bioinformatics. 2010. PMID: 21034510 Free PMC article.
-
Copy number analysis of whole-genome data using BIC-seq2 and its application to detection of cancer susceptibility variants.Nucleic Acids Res. 2016 Jul 27;44(13):6274-86. doi: 10.1093/nar/gkw491. Epub 2016 Jun 3. Nucleic Acids Res. 2016. PMID: 27260798 Free PMC article.
-
A single cell level based method for copy number variation analysis by low coverage massively parallel sequencing.PLoS One. 2013;8(1):e54236. doi: 10.1371/journal.pone.0054236. Epub 2013 Jan 23. PLoS One. 2013. PMID: 23372689 Free PMC article.
-
Copy number variation in the cattle genome.Funct Integr Genomics. 2012 Nov;12(4):609-24. doi: 10.1007/s10142-012-0289-9. Epub 2012 Jul 13. Funct Integr Genomics. 2012. PMID: 22790923 Review.
-
Pharmacogenetics: technologies to detect copy number variations.Curr Opin Mol Ther. 2009 Dec;11(6):670-80. Curr Opin Mol Ther. 2009. PMID: 20072944 Review.
Cited by
-
Distinct Classes of Complex Structural Variation Uncovered across Thousands of Cancer Genome Graphs.Cell. 2020 Oct 1;183(1):197-210.e32. doi: 10.1016/j.cell.2020.08.006. Cell. 2020. PMID: 33007263 Free PMC article.
-
A genetic model for neurodevelopmental disease.Curr Opin Neurobiol. 2012 Oct;22(5):829-36. doi: 10.1016/j.conb.2012.04.007. Epub 2012 May 2. Curr Opin Neurobiol. 2012. PMID: 22560351 Free PMC article. Review.
-
Preprocessing Sequence Coverage Data for More Precise Detection of Copy Number Variations.IEEE/ACM Trans Comput Biol Bioinform. 2020 May-Jun;17(3):868-876. doi: 10.1109/TCBB.2018.2869738. Epub 2018 Sep 12. IEEE/ACM Trans Comput Biol Bioinform. 2020. PMID: 30222580 Free PMC article.
-
Molecular dissection of colorectal cancer in pre-clinical models identifies biomarkers predicting sensitivity to EGFR inhibitors.Nat Commun. 2017 Feb 10;8:14262. doi: 10.1038/ncomms14262. Nat Commun. 2017. PMID: 28186126 Free PMC article.
-
NGSCheckMate: software for validating sample identity in next-generation sequencing studies within and across data types.Nucleic Acids Res. 2017 Jun 20;45(11):e103. doi: 10.1093/nar/gkx193. Nucleic Acids Res. 2017. PMID: 28369524 Free PMC article.
References
Publication types
MeSH terms
Grants and funding
LinkOut - more resources
Full Text Sources
Other Literature Sources