Quantitative dissection of the simple repression input-output function
- PMID: 21730194
- PMCID: PMC3141941
- DOI: 10.1073/pnas.1015616108
Quantitative dissection of the simple repression input-output function
Abstract
We present a quantitative case study of transcriptional regulation in which we carry out a systematic dialogue between theory and measurement for an important and ubiquitous regulatory motif in bacteria, namely, that of simple repression. This architecture is realized by a single repressor binding site overlapping the promoter. From the theory point of view, this motif is described by a single gene regulation function based upon only a few parameters that are convenient theoretically and accessible experimentally. The usual approach is turned on its side by using the mathematical description of these regulatory motifs as a predictive tool to determine the number of repressors in a collection of strains with a large variation in repressor copy number. The predictions and corresponding measurements are carried out over a large dynamic range in both expression fold change (spanning nearly four orders of magnitude) and repressor copy number (spanning about two orders of magnitude). The predictions are tested by measuring the resulting level of gene expression and are then validated by using quantitative immunoblots. The key outcomes of this study include a systematic quantitative analysis of the limits and validity of the input-output relation for simple repression, a precise determination of the in vivo binding energies for DNA-repressor interactions for several distinct repressor binding sites, and a repressor census for Lac repressor in Escherichia coli.
Conflict of interest statement
The authors declare no conflict of interest.
Figures
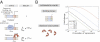
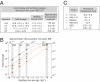
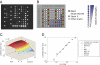
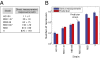
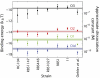
Similar articles
-
Mechanism of promoter repression by Lac repressor-DNA loops.Nucleic Acids Res. 2013 Jan 7;41(1):156-66. doi: 10.1093/nar/gks1011. Epub 2012 Nov 9. Nucleic Acids Res. 2013. PMID: 23143103 Free PMC article.
-
Tuning Transcriptional Regulation through Signaling: A Predictive Theory of Allosteric Induction.Cell Syst. 2018 Apr 25;6(4):456-469.e10. doi: 10.1016/j.cels.2018.02.004. Epub 2018 Mar 21. Cell Syst. 2018. PMID: 29574055 Free PMC article.
-
In vivo tests of thermodynamic models of transcription repressor function.Biophys Chem. 2011 Nov;159(1):142-51. doi: 10.1016/j.bpc.2011.06.005. Epub 2011 Jun 15. Biophys Chem. 2011. PMID: 21715082 Free PMC article.
-
Direct and indirect effects in the regulation of overlapping promoters.Nucleic Acids Res. 2011 Sep 1;39(16):6879-85. doi: 10.1093/nar/gkr390. Epub 2011 May 23. Nucleic Acids Res. 2011. PMID: 21609952 Free PMC article.
-
The lac repressor.C R Biol. 2005 Jun;328(6):521-48. doi: 10.1016/j.crvi.2005.04.004. C R Biol. 2005. PMID: 15950160 Review.
Cited by
-
Deciphering regulatory architectures from synthetic single-cell expression patterns.bioRxiv [Preprint]. 2024 Jun 5:2024.01.28.577658. doi: 10.1101/2024.01.28.577658. bioRxiv. 2024. Update in: PLoS Comput Biol. 2024 Dec 26;20(12):e1012697. doi: 10.1371/journal.pcbi.1012697. PMID: 38352569 Free PMC article. Updated. Preprint.
-
Tuning promoter strength through RNA polymerase binding site design in Escherichia coli.PLoS Comput Biol. 2012;8(12):e1002811. doi: 10.1371/journal.pcbi.1002811. Epub 2012 Dec 13. PLoS Comput Biol. 2012. PMID: 23271961 Free PMC article.
-
Cis-Regulatory Logic Produces Gene-Expression Noise Describing Phenotypic Heterogeneity in Bacteria.Front Genet. 2021 Sep 28;12:698910. doi: 10.3389/fgene.2021.698910. eCollection 2021. Front Genet. 2021. PMID: 34650591 Free PMC article. Review.
-
Theory in Biology: Figure 1 or Figure 7?Trends Cell Biol. 2015 Dec;25(12):723-729. doi: 10.1016/j.tcb.2015.10.007. Epub 2015 Nov 13. Trends Cell Biol. 2015. PMID: 26584768 Free PMC article. Review.
-
Quantitative imaging of RNA polymerase II activity in plants reveals the single-cell basis of tissue-wide transcriptional dynamics.Nat Plants. 2021 Aug;7(8):1037-1049. doi: 10.1038/s41477-021-00976-0. Epub 2021 Aug 9. Nat Plants. 2021. PMID: 34373604 Free PMC article.
References
-
- Endy D. Foundations for engineering biology. Nature. 2005;438:449–453. - PubMed
-
- Voigt CA. Genetic parts to program bacteria. Curr Opin Biotechnol. 2006;17:548–557. - PubMed
-
- Ben-Tabou de-Leon S, Davidson EH. Gene regulation: Gene control network in development. Annu Rev Biophys Biomol Struct. 2007;36:191. - PubMed
Publication types
MeSH terms
Substances
Grants and funding
LinkOut - more resources
Full Text Sources