Prioritizing candidate disease genes by network-based boosting of genome-wide association data
- PMID: 21536720
- PMCID: PMC3129253
- DOI: 10.1101/gr.118992.110
Prioritizing candidate disease genes by network-based boosting of genome-wide association data
Abstract
Network "guilt by association" (GBA) is a proven approach for identifying novel disease genes based on the observation that similar mutational phenotypes arise from functionally related genes. In principle, this approach could account even for nonadditive genetic interactions, which underlie the synergistic combinations of mutations often linked to complex diseases. Here, we analyze a large-scale, human gene functional interaction network (dubbed HumanNet). We show that candidate disease genes can be effectively identified by GBA in cross-validated tests using label propagation algorithms related to Google's PageRank. However, GBA has been shown to work poorly in genome-wide association studies (GWAS), where many genes are somewhat implicated, but few are known with very high certainty. Here, we resolve this by explicitly modeling the uncertainty of the associations and incorporating the uncertainty for the seed set into the GBA framework. We observe a significant boost in the power to detect validated candidate genes for Crohn's disease and type 2 diabetes by comparing our predictions to results from follow-up meta-analyses, with incorporation of the network serving to highlight the JAK-STAT pathway and associated adaptors GRB2/SHC1 in Crohn's disease and BACH2 in type 2 diabetes. Consideration of the network during GWAS thus conveys some of the benefits of enrolling more participants in the GWAS study. More generally, we demonstrate that a functional network of human genes provides a valuable statistical framework for prioritizing candidate disease genes, both for candidate gene-based and GWAS-based studies.
Figures
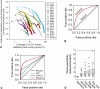
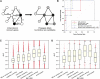
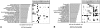
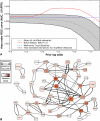
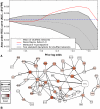
Similar articles
-
Genome-wide meta-analysis of genetic susceptible genes for Type 2 Diabetes.BMC Syst Biol. 2012;6 Suppl 3(Suppl 3):S16. doi: 10.1186/1752-0509-6-S3-S16. Epub 2012 Dec 17. BMC Syst Biol. 2012. PMID: 23281828 Free PMC article.
-
Gene, pathway and network frameworks to identify epistatic interactions of single nucleotide polymorphisms derived from GWAS data.BMC Syst Biol. 2012;6 Suppl 3(Suppl 3):S15. doi: 10.1186/1752-0509-6-S3-S15. Epub 2012 Dec 17. BMC Syst Biol. 2012. PMID: 23281810 Free PMC article.
-
Integrated enrichment analysis of variants and pathways in genome-wide association studies indicates central role for IL-2 signaling genes in type 1 diabetes, and cytokine signaling genes in Crohn's disease.PLoS Genet. 2013;9(10):e1003770. doi: 10.1371/journal.pgen.1003770. Epub 2013 Oct 3. PLoS Genet. 2013. PMID: 24098138 Free PMC article.
-
Network propagation for GWAS analysis: a practical guide to leveraging molecular networks for disease gene discovery.Brief Bioinform. 2024 Jan 22;25(2):bbae014. doi: 10.1093/bib/bbae014. Brief Bioinform. 2024. PMID: 38340090 Free PMC article. Review.
-
Prioritizing GWAS results: A review of statistical methods and recommendations for their application.Am J Hum Genet. 2010 Jan;86(1):6-22. doi: 10.1016/j.ajhg.2009.11.017. Am J Hum Genet. 2010. PMID: 20074509 Free PMC article. Review.
Cited by
-
Selecting causal genes from genome-wide association studies via functionally coherent subnetworks.Nat Methods. 2015 Feb;12(2):154-9. doi: 10.1038/nmeth.3215. Epub 2014 Dec 22. Nat Methods. 2015. PMID: 25532137 Free PMC article.
-
Chapter 2: Data-driven view of disease biology.PLoS Comput Biol. 2012;8(12):e1002816. doi: 10.1371/journal.pcbi.1002816. Epub 2012 Dec 27. PLoS Comput Biol. 2012. PMID: 23300408 Free PMC article.
-
Whole Transcriptome Profiling Identifies CD93 and Other Plasma Cell Survival Factor Genes Associated with Measles-Specific Antibody Response after Vaccination.PLoS One. 2016 Aug 16;11(8):e0160970. doi: 10.1371/journal.pone.0160970. eCollection 2016. PLoS One. 2016. PMID: 27529750 Free PMC article.
-
A framework for pathway knowledge driven prioritization in genome-wide association studies.Genet Epidemiol. 2020 Nov;44(8):841-853. doi: 10.1002/gepi.22345. Epub 2020 Aug 10. Genet Epidemiol. 2020. PMID: 32779262 Free PMC article.
-
Exploiting protein-protein interaction networks for genome-wide disease-gene prioritization.PLoS One. 2012;7(9):e43557. doi: 10.1371/journal.pone.0043557. Epub 2012 Sep 21. PLoS One. 2012. PMID: 23028459 Free PMC article.
References
-
- Akerblad P, Mansson R, Lagergren A, Westerlund S, Basta B, Lind U, Thelin A, Gisler R, Liberg D, Nelander S, et al. 2005. Gene expression analysis suggests that EBF-1 and PPARγ2 induce adipogenesis of NIH-3T3 cells with similar efficiency and kinetics. Physiol Genomics 23: 206–216 - PubMed
Publication types
MeSH terms
Substances
Grants and funding
LinkOut - more resources
Full Text Sources
Other Literature Sources
Medical
Research Materials
Miscellaneous