Virtual screening using molecular simulations
- PMID: 21491494
- PMCID: PMC3092865
- DOI: 10.1002/prot.23018
Virtual screening using molecular simulations
Abstract
Effective virtual screening relies on our ability to make accurate prediction of protein-ligand binding, which remains a great challenge. In this work, utilizing the molecular-mechanics Poisson-Boltzmann (or Generalized Born) surface area approach, we have evaluated the binding affinity of a set of 156 ligands to seven families of proteins, trypsin β, thrombin α, cyclin-dependent kinase (CDK), cAMP-dependent kinase (PKA), urokinase-type plasminogen activator, β-glucosidase A, and coagulation factor Xa. The effect of protein dielectric constant in the implicit-solvent model on the binding free energy calculation is shown to be important. The statistical correlations between the binding energy calculated from the implicit-solvent approach and experimental free energy are in the range of 0.56-0.79 across all the families. This performance is better than that of typical docking programs especially given that the latter is directly trained using known binding data whereas the molecular mechanics is based on general physical parameters. Estimation of entropic contribution remains the barrier to accurate free energy calculation. We show that the traditional rigid rotor harmonic oscillator approximation is unable to improve the binding free energy prediction. Inclusion of conformational restriction seems to be promising but requires further investigation. On the other hand, our preliminary study suggests that implicit-solvent based alchemical perturbation, which offers explicit sampling of configuration entropy, can be a viable approach to significantly improve the prediction of binding free energy. Overall, the molecular mechanics approach has the potential for medium to high-throughput computational drug discovery.
Copyright © 2011 Wiley-Liss, Inc.
Figures
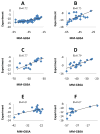
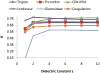
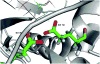
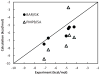
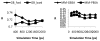
Similar articles
-
Develop and test a solvent accessible surface area-based model in conformational entropy calculations.J Chem Inf Model. 2012 May 25;52(5):1199-212. doi: 10.1021/ci300064d. Epub 2012 Apr 24. J Chem Inf Model. 2012. PMID: 22497310 Free PMC article.
-
Influence of the solvent representation on vibrational entropy calculations: generalized born versus distance-dependent dielectric model.J Comput Chem. 2012 Apr 5;33(9):1004-13. doi: 10.1002/jcc.22933. Epub 2012 Feb 1. J Comput Chem. 2012. PMID: 22298332
-
How Well Does the Extended Linear Interaction Energy Method Perform in Accurate Binding Free Energy Calculations?J Chem Inf Model. 2020 Dec 28;60(12):6624-6633. doi: 10.1021/acs.jcim.0c00934. Epub 2020 Nov 19. J Chem Inf Model. 2020. PMID: 33213150
-
The MM/PBSA and MM/GBSA methods to estimate ligand-binding affinities.Expert Opin Drug Discov. 2015 May;10(5):449-61. doi: 10.1517/17460441.2015.1032936. Epub 2015 Apr 2. Expert Opin Drug Discov. 2015. PMID: 25835573 Free PMC article. Review.
-
Advances in Docking.Curr Med Chem. 2019;26(42):7555-7580. doi: 10.2174/0929867325666180904115000. Curr Med Chem. 2019. PMID: 30182836 Review.
Cited by
-
Biomolecular electrostatics and solvation: a computational perspective.Q Rev Biophys. 2012 Nov;45(4):427-91. doi: 10.1017/S003358351200011X. Q Rev Biophys. 2012. PMID: 23217364 Free PMC article. Review.
-
Promising terpenes as SARS-CoV-2 spike receptor-binding domain (RBD) attachment inhibitors to the human ACE2 receptor: Integrated computational approach.J Mol Liq. 2020 Dec 15;320:114493. doi: 10.1016/j.molliq.2020.114493. Epub 2020 Oct 7. J Mol Liq. 2020. PMID: 33041407 Free PMC article.
-
An Immunoinformatics Approach to Design a Potent Multi-Epitope Vaccine against Asia-1 Genotype of Crimean-Congo Haemorrhagic Fever Virus Using the Structural Glycoproteins as a Target.Vaccines (Basel). 2022 Dec 27;11(1):61. doi: 10.3390/vaccines11010061. Vaccines (Basel). 2022. PMID: 36679906 Free PMC article.
-
Mollusc-Derived Brominated Indoles for the Selective Inhibition of Cyclooxygenase: A Computational Expedition.Molecules. 2021 Oct 29;26(21):6538. doi: 10.3390/molecules26216538. Molecules. 2021. PMID: 34770946 Free PMC article.
-
Machine learning accelerates MD-based binding pose prediction between ligands and proteins.Bioinformatics. 2018 Mar 1;34(5):770-778. doi: 10.1093/bioinformatics/btx638. Bioinformatics. 2018. PMID: 29040432 Free PMC article.
References
-
- Paul SM, Mytelka DS, Dunwiddie CT, Persinger CC, Munos BH, Lindborg SR, Schacht AL. How to improve R&D productivity: the pharmaceutical industry’s grand challenge. Nat Rev Drug Discov. 2010;9:203–214. - PubMed
-
- Dean NM. Functional genomics and target validation approaches using antisense oligonucleotide technology. Curr Opin Biotechnol. 2001;12:622–625. - PubMed
-
- Otto S, Furlan RL, Sanders JK. Dynamic combinatorial chemistry. Drug Discov Today. 2002;7:117–125. - PubMed
-
- Li AP. Screening for human ADME/Tox drug properties in drug discovery. Drug Discov Today. 2001;6:357–366. - PubMed
-
- Greer J, Erickson JW, Baldwin JJ, Varney MD. Application of the three-dimensional structures of protein target molecules in structure-based drug design. J Med Chem. 1994;37:1035–1054. - PubMed
Publication types
MeSH terms
Substances
Grants and funding
LinkOut - more resources
Full Text Sources
Other Literature Sources