MicroRNA transfection and AGO-bound CLIP-seq data sets reveal distinct determinants of miRNA action
- PMID: 21389147
- PMCID: PMC3078732
- DOI: 10.1261/rna.2387911
MicroRNA transfection and AGO-bound CLIP-seq data sets reveal distinct determinants of miRNA action
Abstract
Microarray expression analyses following miRNA transfection/inhibition and, more recently, Argonaute cross-linked immunoprecipitation (CLIP)-seq assays have been used to detect miRNA target sites. CLIP and expression approaches measure differing stages of miRNA functioning-initial binding of the miRNP complex and subsequent message repression. We use nonparametric predictive models to characterize a large number of known target and flanking features, utilizing miRNA transfection, HITS-CLIP, and PAR-CLIP data. In particular, we utilize the precise spatial information provided by CLIP-seq to analyze the predictive effect of target flanking features. We observe distinct target determinants between expression-based and CLIP-based data. Target flanking features such as flanking region conservation are an important AGO-binding determinant-we hypothesize that CLIP experiments have a preference for strongly bound miRNP-target interactions involving adjacent RNA-binding proteins that increase the strength of cross-linking. In contrast, seed-related features are major determinants in expression-based studies, but less so for CLIP-seq studies, and increased miRNA concentrations typical of transfection studies contribute to this difference. While there is a good overlap between miRNA targets detected by miRNA transfection and CLIP-seq, the detection of CLIP-seq targets is largely independent of the level of subsequent mRNA degradation. Also, models built using CLIP-seq data show strong predictive power between independent CLIP-seq data sets, but are not strongly predictive for expression change. Similarly, models built from expression data are not strongly predictive for CLIP-seq data sets, supporting the finding that the determinants of miRNA binding and mRNA degradation differ. Predictive models and results are available at http://servers.binf.ku.dk/antar/.
Figures
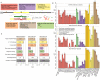
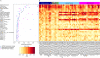
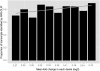
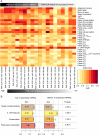
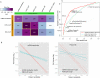
Similar articles
-
A computational approach for identifying microRNA-target interactions using high-throughput CLIP and PAR-CLIP sequencing.BMC Genomics. 2013;14 Suppl 1(Suppl 1):S2. doi: 10.1186/1471-2164-14-S1-S2. Epub 2013 Jan 21. BMC Genomics. 2013. PMID: 23368412 Free PMC article.
-
CLIP-based prediction of mammalian microRNA binding sites.Nucleic Acids Res. 2013 Aug;41(14):e138. doi: 10.1093/nar/gkt435. Epub 2013 May 22. Nucleic Acids Res. 2013. PMID: 23703212 Free PMC article.
-
starBase: a database for exploring microRNA-mRNA interaction maps from Argonaute CLIP-Seq and Degradome-Seq data.Nucleic Acids Res. 2011 Jan;39(Database issue):D202-9. doi: 10.1093/nar/gkq1056. Epub 2010 Oct 30. Nucleic Acids Res. 2011. PMID: 21037263 Free PMC article.
-
Recent computational developments on CLIP-seq data analysis and microRNA targeting implications.Brief Bioinform. 2018 Nov 27;19(6):1290-1301. doi: 10.1093/bib/bbx063. Brief Bioinform. 2018. PMID: 28605404 Free PMC article. Review.
-
HITS-CLIP and PAR-CLIP advance viral miRNA targetome analysis.Crit Rev Eukaryot Gene Expr. 2014;24(2):101-16. doi: 10.1615/critreveukaryotgeneexpr.2014006367. Crit Rev Eukaryot Gene Expr. 2014. PMID: 24940765 Free PMC article. Review.
Cited by
-
Decoding the cardiac message: the 2011 Thomas W. Smith Memorial Lecture.Circ Res. 2012 Mar 2;110(5):755-63. doi: 10.1161/CIRCRESAHA.111.256768. Circ Res. 2012. PMID: 22383710 Free PMC article. Review.
-
A Rapid Evolving microRNA Cluster Rewires Its Target Regulatory Networks in Drosophila.Front Genet. 2021 Oct 28;12:760530. doi: 10.3389/fgene.2021.760530. eCollection 2021. Front Genet. 2021. PMID: 34777478 Free PMC article.
-
Allogeneic T cell responses are regulated by a specific miRNA-mRNA network.J Clin Invest. 2013 Nov;123(11):4739-54. doi: 10.1172/JCI70013. J Clin Invest. 2013. PMID: 24216511 Free PMC article.
-
Identification of novel targets for miR-29a using miRNA proteomics.PLoS One. 2012;7(8):e43243. doi: 10.1371/journal.pone.0043243. Epub 2012 Aug 27. PLoS One. 2012. PMID: 22952654 Free PMC article.
-
Non-MHC risk alleles in rheumatoid arthritis and in the syntenic chromosome regions of corresponding animal models.Clin Dev Immunol. 2012;2012:284751. doi: 10.1155/2012/284751. Epub 2012 Dec 6. Clin Dev Immunol. 2012. PMID: 23251214 Free PMC article. Review.
References
-
- Bartel DP, Chen CZ 2004. Micromanagers of gene expression: the potentially widespread influence of metazoan microRNAs. Nat Rev Genet 5: 396–400 - PubMed
-
- Breiman L 2001. Random forests. Mach Learn 45: 5–32
-
- Brodersen P, Voinnet O 2009. Revisiting the principles of microRNA target recognition and mode of action. Nat Rev Mol Cell Biol 10: 141–148 - PubMed
Publication types
MeSH terms
Substances
LinkOut - more resources
Full Text Sources