Epidemic malaria and warmer temperatures in recent decades in an East African highland
- PMID: 21068045
- PMCID: PMC3081772
- DOI: 10.1098/rspb.2010.2020
Epidemic malaria and warmer temperatures in recent decades in an East African highland
Abstract
Climate change impacts on malaria are typically assessed with scenarios for the long-term future. Here we focus instead on the recent past (1970-2003) to address whether warmer temperatures have already increased the incidence of malaria in a highland region of East Africa. Our analyses rely on a new coupled mosquito-human model of malaria, which we use to compare projected disease levels with and without the observed temperature trend. Predicted malaria cases exhibit a highly nonlinear response to warming, with a significant increase from the 1970s to the 1990s, although typical epidemic sizes are below those observed. These findings suggest that climate change has already played an important role in the exacerbation of malaria in this region. As the observed changes in malaria are even larger than those predicted by our model, other factors previously suggested to explain all of the increase in malaria may be enhancing the impact of climate change.
Figures
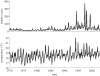
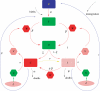
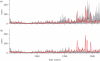
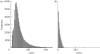
Similar articles
-
Malaria trends in Ethiopian highlands track the 2000 'slowdown' in global warming.Nat Commun. 2021 Mar 10;12(1):1555. doi: 10.1038/s41467-021-21815-y. Nat Commun. 2021. PMID: 33692343 Free PMC article.
-
Malaria smear positivity among Kenyan children peaks at intermediate temperatures as predicted by ecological models.Parasit Vectors. 2019 Jun 6;12(1):288. doi: 10.1186/s13071-019-3547-z. Parasit Vectors. 2019. PMID: 31171037 Free PMC article.
-
Association between climate variability and malaria epidemics in the East African highlands.Proc Natl Acad Sci U S A. 2004 Feb 24;101(8):2375-80. doi: 10.1073/pnas.0308714100. Proc Natl Acad Sci U S A. 2004. PMID: 14983017 Free PMC article.
-
Climate, environment and transmission of malaria.Infez Med. 2016 Jun 1;24(2):93-104. Infez Med. 2016. PMID: 27367318 Review.
-
The ecology of Anopheles mosquitoes under climate change: case studies from the effects of deforestation in East African highlands.Ann N Y Acad Sci. 2012 Feb;1249:204-10. doi: 10.1111/j.1749-6632.2011.06432.x. Epub 2012 Feb 9. Ann N Y Acad Sci. 2012. PMID: 22320421 Free PMC article. Review.
Cited by
-
Effects of Hurricane Irma on mosquito abundance and species composition in a metropolitan Gulf coastal city, 2016-2018.Sci Rep. 2024 Sep 19;14(1):21886. doi: 10.1038/s41598-024-72734-z. Sci Rep. 2024. PMID: 39300158 Free PMC article.
-
Climate change, malaria and neglected tropical diseases: a scoping review.Trans R Soc Trop Med Hyg. 2024 Sep 2;118(9):561-579. doi: 10.1093/trstmh/trae026. Trans R Soc Trop Med Hyg. 2024. PMID: 38724044 Free PMC article. Review.
-
Predicting malaria risk considering vector control interventions under climate change scenarios.Sci Rep. 2024 Jan 29;14(1):2430. doi: 10.1038/s41598-024-52724-x. Sci Rep. 2024. PMID: 38286803 Free PMC article.
-
Global warming may significantly increase childhood anemia burden in sub-Saharan Africa.One Earth. 2023 Oct 20;6(10):1388-1399. doi: 10.1016/j.oneear.2023.09.003. One Earth. 2023. PMID: 37904727 Free PMC article.
-
Time lag effect on malaria transmission dynamics in an Amazonian Colombian municipality and importance for early warning systems.Sci Rep. 2023 Oct 30;13(1):18636. doi: 10.1038/s41598-023-44821-0. Sci Rep. 2023. PMID: 37903862 Free PMC article.
References
-
- MacDonald G. 1957. The epidemiology and control of malaria. Oxford, UK: Oxford University Press
-
- Metselaar D., van Theil P. M. 1959. Classification of malaria. Trop. Geogr. Med. 11, 157–161
-
- Detinova T. 1962. Age-grouping methods in Diptera of medical importance, vol. 47 World Health Organization Monograph Series Geneva, Switzerland: World Health Organization - PubMed
-
- Lindsay S. W., Birley M. H. 1996. Climate change and malaria transmission. Ann. Trop. Med. Parasitol. 90, 573–588 - PubMed
-
- Rogers D. J., Randolph S. E. 2000. The global spread of malaria in a future, warmer world. Science 289, 1763–176610.1126/science.289.5478.391b (doi:10.1126/science.289.5478.391b) - DOI - DOI - PubMed
Publication types
MeSH terms
Grants and funding
LinkOut - more resources
Full Text Sources
Medical
Research Materials
Miscellaneous