MCL-CAw: a refinement of MCL for detecting yeast complexes from weighted PPI networks by incorporating core-attachment structure
- PMID: 20939868
- PMCID: PMC2965181
- DOI: 10.1186/1471-2105-11-504
MCL-CAw: a refinement of MCL for detecting yeast complexes from weighted PPI networks by incorporating core-attachment structure
Abstract
Background: The reconstruction of protein complexes from the physical interactome of organisms serves as a building block towards understanding the higher level organization of the cell. Over the past few years, several independent high-throughput experiments have helped to catalogue enormous amount of physical protein interaction data from organisms such as yeast. However, these individual datasets show lack of correlation with each other and also contain substantial number of false positives (noise). Over these years, several affinity scoring schemes have also been devised to improve the qualities of these datasets. Therefore, the challenge now is to detect meaningful as well as novel complexes from protein interaction (PPI) networks derived by combining datasets from multiple sources and by making use of these affinity scoring schemes. In the attempt towards tackling this challenge, the Markov Clustering algorithm (MCL) has proved to be a popular and reasonably successful method, mainly due to its scalability, robustness, and ability to work on scored (weighted) networks. However, MCL produces many noisy clusters, which either do not match known complexes or have additional proteins that reduce the accuracies of correctly predicted complexes.
Results: Inspired by recent experimental observations by Gavin and colleagues on the modularity structure in yeast complexes and the distinctive properties of "core" and "attachment" proteins, we develop a core-attachment based refinement method coupled to MCL for reconstruction of yeast complexes from scored (weighted) PPI networks. We combine physical interactions from two recent "pull-down" experiments to generate an unscored PPI network. We then score this network using available affinity scoring schemes to generate multiple scored PPI networks. The evaluation of our method (called MCL-CAw) on these networks shows that: (i) MCL-CAw derives larger number of yeast complexes and with better accuracies than MCL, particularly in the presence of natural noise; (ii) Affinity scoring can effectively reduce the impact of noise on MCL-CAw and thereby improve the quality (precision and recall) of its predicted complexes; (iii) MCL-CAw responds well to most available scoring schemes. We discuss several instances where MCL-CAw was successful in deriving meaningful complexes, and where it missed a few proteins or whole complexes due to affinity scoring of the networks. We compare MCL-CAw with several recent complex detection algorithms on unscored and scored networks, and assess the relative performance of the algorithms on these networks. Further, we study the impact of augmenting physical datasets with computationally inferred interactions for complex detection. Finally, we analyse the essentiality of proteins within predicted complexes to understand a possible correlation between protein essentiality and their ability to form complexes.
Conclusions: We demonstrate that core-attachment based refinement in MCL-CAw improves the predictions of MCL on yeast PPI networks. We show that affinity scoring improves the performance of MCL-CAw.
Figures
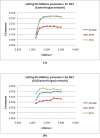
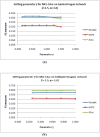
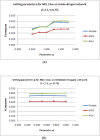
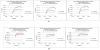
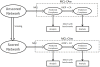
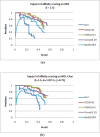
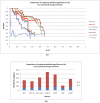
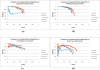
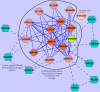
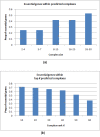
Similar articles
-
Refining Markov Clustering for protein complex prediction by incorporating core-attachment structure.Genome Inform. 2009 Oct;23(1):159-68. Genome Inform. 2009. PMID: 20180271
-
Markov clustering versus affinity propagation for the partitioning of protein interaction graphs.BMC Bioinformatics. 2009 Mar 30;10:99. doi: 10.1186/1471-2105-10-99. BMC Bioinformatics. 2009. PMID: 19331680 Free PMC article.
-
Employing functional interactions for characterisation and detection of sparse complexes from yeast PPI networks.Int J Bioinform Res Appl. 2012;8(3-4):286-304. doi: 10.1504/IJBRA.2012.048962. Int J Bioinform Res Appl. 2012. PMID: 22961456
-
Yeast-based screening platforms to understand and improve human health.Trends Biotechnol. 2024 Oct;42(10):1258-1272. doi: 10.1016/j.tibtech.2024.04.003. Epub 2024 Apr 26. Trends Biotechnol. 2024. PMID: 38677901 Review.
-
Protein-protein interactions: switch from classical methods to proteomics and bioinformatics-based approaches.Cell Mol Life Sci. 2014 Jan;71(2):205-28. doi: 10.1007/s00018-013-1333-1. Epub 2013 Apr 12. Cell Mol Life Sci. 2014. PMID: 23579629 Free PMC article. Review.
Cited by
-
A Central Edge Selection Based Overlapping Community Detection Algorithm for the Detection of Overlapping Structures in Protein⁻Protein Interaction Networks.Molecules. 2018 Oct 13;23(10):2633. doi: 10.3390/molecules23102633. Molecules. 2018. PMID: 30322177 Free PMC article.
-
Fundamentals of protein interaction network mapping.Mol Syst Biol. 2015 Dec 17;11(12):848. doi: 10.15252/msb.20156351. Mol Syst Biol. 2015. PMID: 26681426 Free PMC article. Review.
-
A method for predicting protein complex in dynamic PPI networks.BMC Bioinformatics. 2016 Jul 25;17 Suppl 7(Suppl 7):229. doi: 10.1186/s12859-016-1101-y. BMC Bioinformatics. 2016. PMID: 27454775 Free PMC article.
-
Supervised maximum-likelihood weighting of composite protein networks for complex prediction.BMC Syst Biol. 2012;6 Suppl 2(Suppl 2):S13. doi: 10.1186/1752-0509-6-S2-S13. Epub 2012 Dec 12. BMC Syst Biol. 2012. PMID: 23281936 Free PMC article.
-
Identifying Protein Complexes from Dynamic Temporal Interval Protein-Protein Interaction Networks.Biomed Res Int. 2019 Aug 21;2019:3726721. doi: 10.1155/2019/3726721. eCollection 2019. Biomed Res Int. 2019. PMID: 31531351 Free PMC article.
References
-
- Uetz P, Giot L, Cagney G, Traci A, Judson R, Knight J, Lockshon D, Narayan V, Srinivasan M, Pochart P, Emil QA, Li Y, Godwin B, Conover D, Kalbfleisch T, Vijayadamodar G, Yang M, Johnston M, Fields S, Rothberg M. A comprehensive analysis of protein-protein interactions in Saccharomyces cerevisiae. Nature. 2000;403:623–627. doi: 10.1038/35001009. - DOI - PubMed
-
- Gavin AC, Bosche M, Krause R, Grandi P, Marzioch M, Bauer A, Schultz J, Rick JM, Michon AM, Cruciat CM, Remor M, Hofert C, Schelder M, Brajenovic M, Ruffner H, Merino A, Klien K, Hudak M, Dickson D, Rudi T, Gnau V, Bauch A, Bastuck S, Huhse B, Leutwin C, Heurtier MA, Copley RR, Edelmann A, Rybin V, Drewes G, Raida M, Bouwmeester T, Bork P, Sepharin B, Kuster B, Neubauer G, Furga GS. Functional organization of the yeast proteome by systematic analysis of protein complexes. Nature. 2002;415:141–147. doi: 10.1038/415141a. - DOI - PubMed
-
- Ho Y, Gruhler A, Heilbut A, Bader G, Moore L, Adams SL, Millar A, Taylor P, Bennet K, Boutlier K, Yang L, Wolting C, Donaldson I, Schandorff S, Shewnarane J, Vo M, Taggart M, Gouderault M, Muskat B, Alfarano C, Dewar D, Lin Z, Michalickova K, Willems AR, Sassi H, Nielson P, Rasmussen K, Anderson J, Johansen L, Hansen L, Jesperson H, Podtelejnikov A, Nielson E, Crawford J, Poulsen V, Sorensen B, Matthiesen J, Hendrickson RC, Gleeson F, Pawson T, Moran MF, Durocher D, Mann M, Hogue CWV, Figeys D, Tyers M. Systematic identification of protein complexes in Saccharomyces cerevisiae by mass spectrometry. Nature. 2002;415:180–183. doi: 10.1038/415180a. - DOI - PubMed
Publication types
MeSH terms
Substances
LinkOut - more resources
Full Text Sources
Molecular Biology Databases
Miscellaneous