Improving performance of mammalian microRNA target prediction
- PMID: 20860840
- PMCID: PMC2955701
- DOI: 10.1186/1471-2105-11-476
Improving performance of mammalian microRNA target prediction
Abstract
Background: MicroRNAs (miRNAs) are single-stranded non-coding RNAs known to regulate a wide range of cellular processes by silencing the gene expression at the protein and/or mRNA levels. Computational prediction of miRNA targets is essential for elucidating the detailed functions of miRNA. However, the prediction specificity and sensitivity of the existing algorithms are still poor to generate meaningful, workable hypotheses for subsequent experimental testing. Constructing a richer and more reliable training data set and developing an algorithm that properly exploits this data set would be the key to improve the performance current prediction algorithms.
Results: A comprehensive training data set is constructed for mammalian miRNAs with its positive targets obtained from the most up-to-date miRNA target depository called miRecords and its negative targets derived from 20 microarray data. A new algorithm SVMicrO is developed, which assumes a 2-stage structure including a site support vector machine (SVM) followed by a UTR-SVM. SVMicrO makes prediction based on 21 optimal site features and 18 optimal UTR features, selected by training from a comprehensive collection of 113 site and 30 UTR features. Comprehensive evaluation of SVMicrO performance has been carried out on the training data, proteomics data, and immunoprecipitation (IP) pull-down data. Comparisons with some popular algorithms demonstrate consistent improvements in prediction specificity, sensitivity and precision in all tested cases. All the related materials including source code and genome-wide prediction of human targets are available at http://compgenomics.utsa.edu/svmicro.html.
Conclusions: A 2-stage SVM based new miRNA target prediction algorithm called SVMicrO is developed. SVMicrO is shown to be able to achieve robust performance. It holds the promise to achieve continuing improvement whenever better training data that contain additional verified or high confidence positive targets and properly selected negative targets are available.
Figures
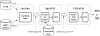
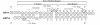
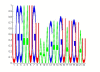
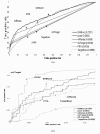
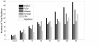
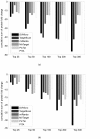
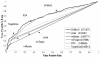
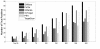
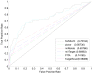
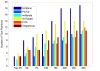
Similar articles
-
A Bayesian approach for identifying miRNA targets by combining sequence prediction and gene expression profiling.BMC Genomics. 2010 Dec 1;11 Suppl 3(Suppl 3):S12. doi: 10.1186/1471-2164-11-S3-S12. BMC Genomics. 2010. PMID: 21143779 Free PMC article.
-
Min3: Predict microRNA target gene using an improved binding-site representation method and support vector machine.J Bioinform Comput Biol. 2019 Oct;17(5):1950032. doi: 10.1142/S021972001950032X. J Bioinform Comput Biol. 2019. PMID: 31856668
-
MiRTif: a support vector machine-based microRNA target interaction filter.BMC Bioinformatics. 2008 Dec 12;9 Suppl 12(Suppl 12):S4. doi: 10.1186/1471-2105-9-S12-S4. BMC Bioinformatics. 2008. PMID: 19091027 Free PMC article.
-
Prediction of human microRNA targets.Methods Mol Biol. 2006;342:101-13. doi: 10.1385/1-59745-123-1:101. Methods Mol Biol. 2006. PMID: 16957370 Review.
-
Complications in mammalian microRNA target prediction.Methods Mol Biol. 2006;342:115-27. doi: 10.1385/1-59745-123-1:115. Methods Mol Biol. 2006. PMID: 16957371 Review.
Cited by
-
PSI-MOUSE: Predicting Mouse Pseudouridine Sites From Sequence and Genome-Derived Features.Evol Bioinform Online. 2020 Jun 9;16:1176934320925752. doi: 10.1177/1176934320925752. eCollection 2020. Evol Bioinform Online. 2020. PMID: 32565674 Free PMC article.
-
Molecular Mechanisms of PD-1 and PD-L1 Activity on a Pan-Cancer Basis: A Bioinformatic Exploratory Study.Int J Mol Sci. 2021 May 22;22(11):5478. doi: 10.3390/ijms22115478. Int J Mol Sci. 2021. PMID: 34067485 Free PMC article.
-
mirDIP 4.1-integrative database of human microRNA target predictions.Nucleic Acids Res. 2018 Jan 4;46(D1):D360-D370. doi: 10.1093/nar/gkx1144. Nucleic Acids Res. 2018. PMID: 29194489 Free PMC article.
-
Single base mismatches in the mRNA target site allow specific seed region-mediated off-target binding of siRNA targeting human coagulation factor 7.RNA Biol. 2012 Jan;9(1):87-97. doi: 10.4161/rna.9.1.18121. Epub 2012 Jan 1. RNA Biol. 2012. PMID: 22258146 Free PMC article.
-
Identification of miR-145 targets through an integrated omics analysis.Mol Biosyst. 2015 Jan;11(1):197-207. doi: 10.1039/c4mb00585f. Epub 2014 Oct 30. Mol Biosyst. 2015. PMID: 25354783 Free PMC article.
References
Publication types
MeSH terms
Substances
Grants and funding
LinkOut - more resources
Full Text Sources