Probabilistic analysis of gene expression measurements from heterogeneous tissues
- PMID: 20631160
- PMCID: PMC2951082
- DOI: 10.1093/bioinformatics/btq406
Probabilistic analysis of gene expression measurements from heterogeneous tissues
Abstract
Motivation: Tissue heterogeneity, arising from multiple cell types, is a major confounding factor in experiments that focus on studying cell types, e.g. their expression profiles, in isolation. Although sample heterogeneity can be addressed by manual microdissection, prior to conducting experiments, computational treatment on heterogeneous measurements have become a reliable alternative to perform this microdissection in silico. Favoring computation over manual purification has its advantages, such as time consumption, measuring responses of multiple cell types simultaneously, keeping samples intact of external perturbations and unaltered yield of molecular content.
Results: We formalize a probabilistic model, DSection, and show with simulations as well as with real microarray data that DSection attains increased modeling accuracy in terms of (i) estimating cell-type proportions of heterogeneous tissue samples, (ii) estimating replication variance and (iii) identifying differential expression across cell types under various experimental conditions. As our reference we use the corresponding linear regression model, which mirrors the performance of the majority of current non-probabilistic modeling approaches. AVAILABILITY AND SOFTWARE: All codes are written in Matlab, and are freely available upon request as well as at the project web page http://www.cs.tut.fi/∼erkkila2/. Furthermore, a web-application for DSection exists at http://informatics.systemsbiology.net/DSection.
Contact: timo.p.erkkila@tut.fi; harri.lahdesmaki@tut.fi
Figures
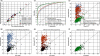

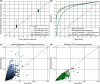
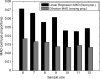
Similar articles
-
MMAD: microarray microdissection with analysis of differences is a computational tool for deconvoluting cell type-specific contributions from tissue samples.Bioinformatics. 2014 Mar 1;30(5):682-9. doi: 10.1093/bioinformatics/btt566. Epub 2013 Oct 1. Bioinformatics. 2014. PMID: 24085566 Free PMC article.
-
In silico microdissection of microarray data from heterogeneous cell populations.BMC Bioinformatics. 2005 Mar 14;6:54. doi: 10.1186/1471-2105-6-54. BMC Bioinformatics. 2005. PMID: 15766384 Free PMC article.
-
Evaluating the performance of microarray segmentation algorithms.Bioinformatics. 2006 Dec 1;22(23):2910-7. doi: 10.1093/bioinformatics/btl502. Epub 2006 Oct 10. Bioinformatics. 2006. PMID: 17032673
-
A sequential Monte Carlo approach to gene expression deconvolution.PLoS One. 2017 Oct 19;12(10):e0186167. doi: 10.1371/journal.pone.0186167. eCollection 2017. PLoS One. 2017. PMID: 29049343 Free PMC article.
-
Interactively optimizing signal-to-noise ratios in expression profiling: project-specific algorithm selection and detection p-value weighting in Affymetrix microarrays.Bioinformatics. 2004 Nov 1;20(16):2534-44. doi: 10.1093/bioinformatics/bth280. Epub 2004 Apr 29. Bioinformatics. 2004. PMID: 15117752
Cited by
-
Exploring Prognostic Immune Microenvironment-Related Genes in Head and Neck Squamous Cell Carcinoma from the TCGA Database.J Cancer. 2024 Jan 1;15(3):632-644. doi: 10.7150/jca.89581. eCollection 2024. J Cancer. 2024. PMID: 38213736 Free PMC article.
-
iProMix: A mixture model for studying the function of ACE2 based on bulk proteogenomic data.J Am Stat Assoc. 2023;118(541):43-55. doi: 10.1080/01621459.2022.2110876. Epub 2022 Oct 5. J Am Stat Assoc. 2023. PMID: 37409267 Free PMC article.
-
Tumor microenvironment: barrier or opportunity towards effective cancer therapy.J Biomed Sci. 2022 Oct 17;29(1):83. doi: 10.1186/s12929-022-00866-3. J Biomed Sci. 2022. PMID: 36253762 Free PMC article. Review.
-
Estimating cell type-specific differential expression using deconvolution.Brief Bioinform. 2022 Jan 17;23(1):bbab433. doi: 10.1093/bib/bbab433. Brief Bioinform. 2022. PMID: 34651640 Free PMC article. No abstract available.
-
CDSeqR: fast complete deconvolution for gene expression data from bulk tissues.BMC Bioinformatics. 2021 May 24;22(1):262. doi: 10.1186/s12859-021-04186-5. BMC Bioinformatics. 2021. PMID: 34030626 Free PMC article.
References
-
- Affymetrix (2009) Available at http://www.affymetrix.com/support/technical/sample_data/gene_1_0_array__.... (last accessed date June 22, 2009)
-
- Andrieu C, et al. An introduction to mcmc for machine learning. Mach. Learn. 2003;50:5–43.
-
- Cowles MK, Carlin BP. Markov chain monte carlo convergence diagnostics: a comparative review. J. Am. Stat. Assoc. 1996;91:883–904.
Publication types
MeSH terms
Substances
Grants and funding
LinkOut - more resources
Full Text Sources
Other Literature Sources
Research Materials