A novel approach to investigate tissue-specific trinucleotide repeat instability
- PMID: 20302627
- PMCID: PMC2856555
- DOI: 10.1186/1752-0509-4-29
A novel approach to investigate tissue-specific trinucleotide repeat instability
Abstract
Background: In Huntington's disease (HD), an expanded CAG repeat produces characteristic striatal neurodegeneration. Interestingly, the HD CAG repeat, whose length determines age at onset, undergoes tissue-specific somatic instability, predominant in the striatum, suggesting that tissue-specific CAG length changes could modify the disease process. Therefore, understanding the mechanisms underlying the tissue specificity of somatic instability may provide novel routes to therapies. However progress in this area has been hampered by the lack of sensitive high-throughput instability quantification methods and global approaches to identify the underlying factors.
Results: Here we describe a novel approach to gain insight into the factors responsible for the tissue specificity of somatic instability. Using accurate genetic knock-in mouse models of HD, we developed a reliable, high-throughput method to quantify tissue HD CAG repeat instability and integrated this with genome-wide bioinformatic approaches. Using tissue instability quantified in 16 tissues as a phenotype and tissue microarray gene expression as a predictor, we built a mathematical model and identified a gene expression signature that accurately predicted tissue instability. Using the predictive ability of this signature we found that somatic instability was not a consequence of pathogenesis. In support of this, genetic crosses with models of accelerated neuropathology failed to induce somatic instability. In addition, we searched for genes and pathways that correlated with tissue instability. We found that expression levels of DNA repair genes did not explain the tissue specificity of somatic instability. Instead, our data implicate other pathways, particularly cell cycle, metabolism and neurotransmitter pathways, acting in combination to generate tissue-specific patterns of instability.
Conclusion: Our study clearly demonstrates that multiple tissue factors reflect the level of somatic instability in different tissues. In addition, our quantitative, genome-wide approach is readily applicable to high-throughput assays and opens the door to widespread applications with the potential to accelerate the discovery of drugs that alter tissue instability.
Figures
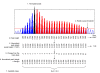
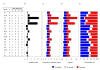
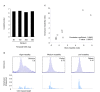
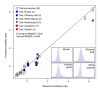
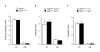
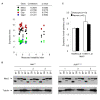
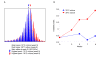
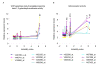
Similar articles
-
Quantification of age-dependent somatic CAG repeat instability in Hdh CAG knock-in mice reveals different expansion dynamics in striatum and liver.PLoS One. 2011;6(8):e23647. doi: 10.1371/journal.pone.0023647. Epub 2011 Aug 29. PLoS One. 2011. PMID: 21897851 Free PMC article.
-
Stoichiometry of base excision repair proteins correlates with increased somatic CAG instability in striatum over cerebellum in Huntington's disease transgenic mice.PLoS Genet. 2009 Dec;5(12):e1000749. doi: 10.1371/journal.pgen.1000749. Epub 2009 Dec 4. PLoS Genet. 2009. PMID: 19997493 Free PMC article.
-
MSH3 polymorphisms and protein levels affect CAG repeat instability in Huntington's disease mice.PLoS Genet. 2013;9(2):e1003280. doi: 10.1371/journal.pgen.1003280. Epub 2013 Feb 28. PLoS Genet. 2013. PMID: 23468640 Free PMC article.
-
Modifiers of Somatic Repeat Instability in Mouse Models of Friedreich Ataxia and the Fragile X-Related Disorders: Implications for the Mechanism of Somatic Expansion in Huntington's Disease.J Huntingtons Dis. 2021;10(1):149-163. doi: 10.3233/JHD-200423. J Huntingtons Dis. 2021. PMID: 33579860 Free PMC article. Review.
-
Methods for Assessing DNA Repair and Repeat Expansion in Huntington's Disease.Methods Mol Biol. 2018;1780:483-495. doi: 10.1007/978-1-4939-7825-0_22. Methods Mol Biol. 2018. PMID: 29856032 Review.
Cited by
-
A CAG repeat threshold for therapeutics targeting somatic instability in Huntington's disease.Brain. 2024 May 3;147(5):1784-1798. doi: 10.1093/brain/awae063. Brain. 2024. PMID: 38387080 Free PMC article.
-
Large expansion of CTG•CAG repeats is exacerbated by MutSβ in human cells.Sci Rep. 2015 Jun 5;5:11020. doi: 10.1038/srep11020. Sci Rep. 2015. PMID: 26047474 Free PMC article.
-
Promotion of somatic CAG repeat expansion by Fan1 knock-out in Huntington's disease knock-in mice is blocked by Mlh1 knock-out.Hum Mol Genet. 2020 Nov 4;29(18):3044-3053. doi: 10.1093/hmg/ddaa196. Hum Mol Genet. 2020. PMID: 32876667 Free PMC article.
-
A MutSβ-Dependent Contribution of MutSα to Repeat Expansions in Fragile X Premutation Mice?PLoS Genet. 2016 Jul 18;12(7):e1006190. doi: 10.1371/journal.pgen.1006190. eCollection 2016 Jul. PLoS Genet. 2016. PMID: 27427765 Free PMC article.
-
CAG Repeat Instability in the Peripheral and Central Nervous System of Transgenic Huntington's Disease Monkeys.Biomedicines. 2022 Aug 2;10(8):1863. doi: 10.3390/biomedicines10081863. Biomedicines. 2022. PMID: 36009409 Free PMC article.
References
-
- Ashizawa T, Dubel JR, Harati Y. Somatic instability of CTG repeat in myotonic dystrophy. Neurology. 1993;43:2674–2678. - PubMed
Publication types
MeSH terms
Substances
Grants and funding
LinkOut - more resources
Full Text Sources
Other Literature Sources
Medical
Molecular Biology Databases