Comparison of techniques for handling missing covariate data within prognostic modelling studies: a simulation study
- PMID: 20085642
- PMCID: PMC2824146
- DOI: 10.1186/1471-2288-10-7
Comparison of techniques for handling missing covariate data within prognostic modelling studies: a simulation study
Abstract
Background: There is no consensus on the most appropriate approach to handle missing covariate data within prognostic modelling studies. Therefore a simulation study was performed to assess the effects of different missing data techniques on the performance of a prognostic model.
Methods: Datasets were generated to resemble the skewed distributions seen in a motivating breast cancer example. Multivariate missing data were imposed on four covariates using four different mechanisms; missing completely at random (MCAR), missing at random (MAR), missing not at random (MNAR) and a combination of all three mechanisms. Five amounts of incomplete cases from 5% to 75% were considered. Complete case analysis (CC), single imputation (SI) and five multiple imputation (MI) techniques available within the R statistical software were investigated: a) data augmentation (DA) approach assuming a multivariate normal distribution, b) DA assuming a general location model, c) regression switching imputation, d) regression switching with predictive mean matching (MICE-PMM) and e) flexible additive imputation models. A Cox proportional hazards model was fitted and appropriate estimates for the regression coefficients and model performance measures were obtained.
Results: Performing a CC analysis produced unbiased regression estimates, but inflated standard errors, which affected the significance of the covariates in the model with 25% or more missingness. Using SI, underestimated the variability; resulting in poor coverage even with 10% missingness. Of the MI approaches, applying MICE-PMM produced, in general, the least biased estimates and better coverage for the incomplete covariates and better model performance for all mechanisms. However, this MI approach still produced biased regression coefficient estimates for the incomplete skewed continuous covariates when 50% or more cases had missing data imposed with a MCAR, MAR or combined mechanism. When the missingness depended on the incomplete covariates, i.e. MNAR, estimates were biased with more than 10% incomplete cases for all MI approaches.
Conclusion: The results from this simulation study suggest that performing MICE-PMM may be the preferred MI approach provided that less than 50% of the cases have missing data and the missing data are not MNAR.
Figures
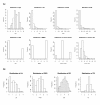
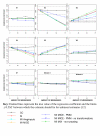
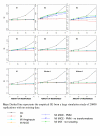
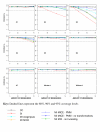
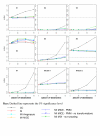
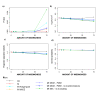
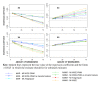
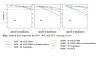
Similar articles
-
Comparison of imputation methods for handling missing covariate data when fitting a Cox proportional hazards model: a resampling study.BMC Med Res Methodol. 2010 Dec 31;10:112. doi: 10.1186/1471-2288-10-112. BMC Med Res Methodol. 2010. PMID: 21194416 Free PMC article.
-
Approaches for missing covariate data in logistic regression with MNAR sensitivity analyses.Biom J. 2020 Jul;62(4):1025-1037. doi: 10.1002/bimj.201900117. Epub 2020 Jan 20. Biom J. 2020. PMID: 31957905
-
Accounting for bias due to outcome data missing not at random: comparison and illustration of two approaches to probabilistic bias analysis: a simulation study.BMC Med Res Methodol. 2024 Nov 13;24(1):278. doi: 10.1186/s12874-024-02382-4. BMC Med Res Methodol. 2024. PMID: 39538117 Free PMC article.
-
Review: a gentle introduction to imputation of missing values.J Clin Epidemiol. 2006 Oct;59(10):1087-91. doi: 10.1016/j.jclinepi.2006.01.014. Epub 2006 Jul 11. J Clin Epidemiol. 2006. PMID: 16980149 Review.
-
Predictors of clinical outcome in pediatric oligodendroglioma: meta-analysis of individual patient data and multiple imputation.J Neurosurg Pediatr. 2018 Feb;21(2):153-163. doi: 10.3171/2017.7.PEDS17133. Epub 2017 Dec 1. J Neurosurg Pediatr. 2018. PMID: 29192869 Review.
Cited by
-
External Validation of a Referral Rule for Axial Spondyloarthritis in Primary Care Patients with Chronic Low Back Pain.PLoS One. 2015 Jul 22;10(7):e0131963. doi: 10.1371/journal.pone.0131963. eCollection 2015. PLoS One. 2015. PMID: 26200904 Free PMC article.
-
Seven-day mortality can be predicted in medical patients by blood pressure, age, respiratory rate, loss of independence, and peripheral oxygen saturation (the PARIS score): a prospective cohort study with external validation.PLoS One. 2015 Apr 13;10(4):e0122480. doi: 10.1371/journal.pone.0122480. eCollection 2015. PLoS One. 2015. PMID: 25867881 Free PMC article.
-
Survival analysis of gastric cancer patients with incomplete data.J Gastric Cancer. 2014 Dec;14(4):259-65. doi: 10.5230/jgc.2014.14.4.259. Epub 2014 Dec 26. J Gastric Cancer. 2014. PMID: 25580358 Free PMC article.
-
Utility of neuron-specific enolase in traumatic brain injury; relations to S100B levels, outcome, and extracranial injury severity.Crit Care. 2016 Sep 8;20:285. doi: 10.1186/s13054-016-1450-y. Crit Care. 2016. PMID: 27604350 Free PMC article.
-
Recovery of information from multiple imputation: a simulation study.Emerg Themes Epidemiol. 2012 Jun 13;9(1):3. doi: 10.1186/1742-7622-9-3. Emerg Themes Epidemiol. 2012. PMID: 22695083 Free PMC article.
References
-
- Vach W, Blettner M, Armitage P, Colton T. Encyclopedia of Biostatistics. New York: John Wiley & Sons; 1998. Missing data in epidemiologic studies; pp. 2641–2654.
Publication types
MeSH terms
Grants and funding
LinkOut - more resources
Full Text Sources
Other Literature Sources
Miscellaneous