Global genomic analysis reveals rapid control of a robust innate response in SIV-infected sooty mangabeys
- PMID: 19959874
- PMCID: PMC2786806
- DOI: 10.1172/JCI40115
Global genomic analysis reveals rapid control of a robust innate response in SIV-infected sooty mangabeys
Abstract
Natural SIV infection of sooty mangabeys (SMs) is nonprogressive despite chronic virus replication. Strikingly, it is characterized by low levels of immune activation, while pathogenic SIV infection of rhesus macaques (RMs) is associated with chronic immune activation. To elucidate the mechanisms underlying this intriguing phenotype, we used high-density oligonucleotide microarrays to longitudinally assess host gene expression in SIV-infected SMs and RMs. We found that acute SIV infection of SMs was consistently associated with a robust innate immune response, including widespread upregulation of IFN-stimulated genes (ISGs) in blood and lymph nodes. While SMs exhibited a rapid resolution of ISG expression and immune activation, both responses were observed chronically in RMs. Systems biology analysis indicated that expression of the lymphocyte inhibitory receptor LAG3, a marker of T cell exhaustion, correlated with immune activation in SIV-infected RMs but not SMs. Our findings suggest that active immune regulatory mechanisms, rather than intrinsically attenuated innate immune responses, underlie the low levels of immune activation characteristic of SMs chronically infected with SIV.
Figures
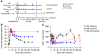
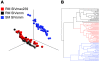
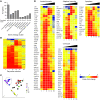
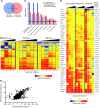
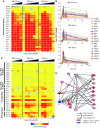
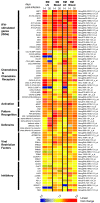
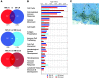
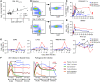
Comment in
-
Resolution of immune activation defines nonpathogenic SIV infection.J Clin Invest. 2009 Dec;119(12):3512-5. doi: 10.1172/JCI41509. J Clin Invest. 2009. PMID: 19959871 Free PMC article. Review.
Similar articles
-
Coexpression Network Analysis of Benign and Malignant Phenotypes of SIV-Infected Sooty Mangabey and Rhesus Macaque.PLoS One. 2016 Jun 9;11(6):e0156170. doi: 10.1371/journal.pone.0156170. eCollection 2016. PLoS One. 2016. PMID: 27280726 Free PMC article.
-
Increased stability and limited proliferation of CD4+ central memory T cells differentiate nonprogressive simian immunodeficiency virus (SIV) infection of sooty mangabeys from progressive SIV infection of rhesus macaques.J Virol. 2014 Apr;88(8):4533-42. doi: 10.1128/JVI.03515-13. Epub 2014 Feb 5. J Virol. 2014. PMID: 24501416 Free PMC article.
-
Reduced Simian Immunodeficiency Virus Replication in Macrophages of Sooty Mangabeys Is Associated with Increased Expression of Host Restriction Factors.J Virol. 2015 Oct;89(20):10136-44. doi: 10.1128/JVI.00710-15. Epub 2015 Jul 22. J Virol. 2015. PMID: 26202248 Free PMC article.
-
A case for innate immune effector mechanisms as contributors to disease resistance in SIV-infected sooty mangabeys.Curr HIV Res. 2009 Jan;7(1):12-22. doi: 10.2174/157016209787048465. Curr HIV Res. 2009. PMID: 19149550 Review.
-
Naturally SIV-infected sooty mangabeys: are we closer to understanding why they do not develop AIDS?J Med Primatol. 2005 Oct;34(5-6):243-52. doi: 10.1111/j.1600-0684.2005.00122.x. J Med Primatol. 2005. PMID: 16128919 Review.
Cited by
-
Epigenetic silencing of CD4 expression in nonpathogenic SIV infection in African green monkeys.JCI Insight. 2020 Sep 17;5(18):e139043. doi: 10.1172/jci.insight.139043. JCI Insight. 2020. PMID: 32841214 Free PMC article.
-
Elite Control, Gut CD4 T Cell Sparing, and Enhanced Mucosal T Cell Responses in Macaca nemestrina Infected by a Simian Immunodeficiency Virus Lacking a gp41 Trafficking Motif.J Virol. 2015 Oct;89(20):10156-75. doi: 10.1128/JVI.01134-15. Epub 2015 Jul 29. J Virol. 2015. PMID: 26223646 Free PMC article.
-
The oral mucosa immune environment and oral transmission of HIV/SIV.Immunol Rev. 2013 Jul;254(1):34-53. doi: 10.1111/imr.12078. Immunol Rev. 2013. PMID: 23772613 Free PMC article. Review.
-
Type I IFNs and CD8 T cells increase intestinal barrier permeability after chronic viral infection.J Exp Med. 2020 Dec 7;217(12):e20192276. doi: 10.1084/jem.20192276. J Exp Med. 2020. PMID: 32880630 Free PMC article.
-
Genetic identity and biological phenotype of a transmitted/founder virus representative of nonpathogenic simian immunodeficiency virus infection in African green monkeys.J Virol. 2010 Dec;84(23):12245-54. doi: 10.1128/JVI.01603-10. Epub 2010 Sep 29. J Virol. 2010. PMID: 20881048 Free PMC article.
References
Publication types
MeSH terms
Substances
Grants and funding
LinkOut - more resources
Full Text Sources
Other Literature Sources
Molecular Biology Databases