Balance diagnostics for comparing the distribution of baseline covariates between treatment groups in propensity-score matched samples
- PMID: 19757444
- PMCID: PMC3472075
- DOI: 10.1002/sim.3697
Balance diagnostics for comparing the distribution of baseline covariates between treatment groups in propensity-score matched samples
Abstract
The propensity score is a subject's probability of treatment, conditional on observed baseline covariates. Conditional on the true propensity score, treated and untreated subjects have similar distributions of observed baseline covariates. Propensity-score matching is a popular method of using the propensity score in the medical literature. Using this approach, matched sets of treated and untreated subjects with similar values of the propensity score are formed. Inferences about treatment effect made using propensity-score matching are valid only if, in the matched sample, treated and untreated subjects have similar distributions of measured baseline covariates. In this paper we discuss the following methods for assessing whether the propensity score model has been correctly specified: comparing means and prevalences of baseline characteristics using standardized differences; ratios comparing the variance of continuous covariates between treated and untreated subjects; comparison of higher order moments and interactions; five-number summaries; and graphical methods such as quantile-quantile plots, side-by-side boxplots, and non-parametric density plots for comparing the distribution of baseline covariates between treatment groups. We describe methods to determine the sampling distribution of the standardized difference when the true standardized difference is equal to zero, thereby allowing one to determine the range of standardized differences that are plausible with the propensity score model having been correctly specified. We highlight the limitations of some previously used methods for assessing the adequacy of the specification of the propensity-score model. In particular, methods based on comparing the distribution of the estimated propensity score between treated and untreated subjects are uninformative.
Figures
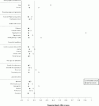
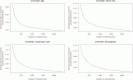
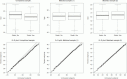
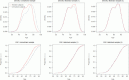
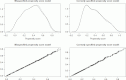
Similar articles
-
Assessing balance in measured baseline covariates when using many-to-one matching on the propensity-score.Pharmacoepidemiol Drug Saf. 2008 Dec;17(12):1218-25. doi: 10.1002/pds.1674. Pharmacoepidemiol Drug Saf. 2008. PMID: 18972455
-
Goodness-of-fit diagnostics for the propensity score model when estimating treatment effects using covariate adjustment with the propensity score.Pharmacoepidemiol Drug Saf. 2008 Dec;17(12):1202-17. doi: 10.1002/pds.1673. Pharmacoepidemiol Drug Saf. 2008. PMID: 18972454
-
The concept of the marginally matched subject in propensity-score matched analyses.Pharmacoepidemiol Drug Saf. 2009 Jun;18(6):469-82. doi: 10.1002/pds.1733. Pharmacoepidemiol Drug Saf. 2009. PMID: 19319923
-
Moving towards best practice when using inverse probability of treatment weighting (IPTW) using the propensity score to estimate causal treatment effects in observational studies.Stat Med. 2015 Dec 10;34(28):3661-79. doi: 10.1002/sim.6607. Epub 2015 Aug 3. Stat Med. 2015. PMID: 26238958 Free PMC article. Review.
-
A critical appraisal of propensity-score matching in the medical literature between 1996 and 2003.Stat Med. 2008 May 30;27(12):2037-49. doi: 10.1002/sim.3150. Stat Med. 2008. PMID: 18038446 Review.
Cited by
-
Nociception level index-directed superficial parasternal intercostal plane block vs erector spinae plane block in open-heart surgery: a propensity matched non-inferiority clinical trial.J Clin Monit Comput. 2024 Oct 29. doi: 10.1007/s10877-024-01236-0. Online ahead of print. J Clin Monit Comput. 2024. PMID: 39470954
-
Venous aneurysms in unruptured supratentorial brain arteriovenous malformations: a protective factor against hemorrhagic stroke and insights into hemodynamic mechanisms.Eur Radiol. 2024 Oct 29. doi: 10.1007/s00330-024-11137-0. Online ahead of print. Eur Radiol. 2024. PMID: 39470793
-
Microfibrillar-associated protein 4 as a predictive biomarker of treatment response in patients with chronic inflammatory diseases initiating biologics: secondary analyses based on the prospective BELIEVE cohort study.Rheumatol Int. 2024 Oct 28. doi: 10.1007/s00296-024-05744-9. Online ahead of print. Rheumatol Int. 2024. PMID: 39465398
-
When does adjusting covariate under randomization help? A comparative study on current practices.BMC Med Res Methodol. 2024 Oct 26;24(1):250. doi: 10.1186/s12874-024-02375-3. BMC Med Res Methodol. 2024. PMID: 39462370 Free PMC article.
-
Epidemiological Evidence for Upper Respiratory Infections as a Potential Risk Factor for Meniere's Disease: A Korean National Health Sample Cohort Study.Microorganisms. 2024 Oct 10;12(10):2047. doi: 10.3390/microorganisms12102047. Microorganisms. 2024. PMID: 39458356 Free PMC article.
References
-
- Rosenbaum PR, Rubin DB. The central role of the propensity score in observational studies for causal effects. Biometrika. 1983;70:41–55.
-
- Rosenbaum PR, Rubin DB. Reducing bias in observational studies using subclassification on the propensity score. Journal of the American Statistical Association. 1984;79:516–524.
-
- Austin PC, Mamdani MM. A comparison of propensity score methods: a case-study estimating the effectiveness of post-AMI statin use. Statistics in Medicine. 2006;25:2084–2106. - PubMed
-
- Weitzen S, Lapane KL, Toledano AY, Hume AL, Mor V. Principles for modeling propensity scores in medical research: a systematic literature review. Pharmacoepidemiology and Drug Safety. 2004;13:841–853. - PubMed
-
- Shah BR, Laupacis A, Hux JE, Austin PC. Propensity score methods give similar results to traditional regression modeling in observational studies: a systematic review. Journal of Clinical Epidemiology. 2005;58:550–559. - PubMed
Publication types
MeSH terms
Substances
LinkOut - more resources
Full Text Sources
Other Literature Sources