Genomic analysis reveals a tight link between transcription factor dynamics and regulatory network architecture
- PMID: 19690563
- PMCID: PMC2736650
- DOI: 10.1038/msb.2009.52
Genomic analysis reveals a tight link between transcription factor dynamics and regulatory network architecture
Abstract
Although several studies have provided important insights into the general principles of biological networks, the link between network organization and the genome-scale dynamics of the underlying entities (genes, mRNAs, and proteins) and its role in systems behavior remain unclear. Here we show that transcription factor (TF) dynamics and regulatory network organization are tightly linked. By classifying TFs in the yeast regulatory network into three hierarchical layers (top, core, and bottom) and integrating diverse genome-scale datasets, we find that the TFs have static and dynamic properties that are similar within a layer and different across layers. At the protein level, the top-layer TFs are relatively abundant, long-lived, and noisy compared with the core- and bottom-layer TFs. Although variability in expression of top-layer TFs might confer a selective advantage, as this permits at least some members in a clonal cell population to initiate a response to changing conditions, tight regulation of the core- and bottom-layer TFs may minimize noise propagation and ensure fidelity in regulation. We propose that the interplay between network organization and TF dynamics could permit differential utilization of the same underlying network by distinct members of a clonal cell population.
Conflict of interest statement
The authors declare that they have no conflict of interest.
Figures
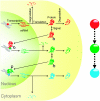
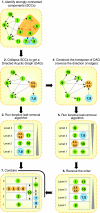
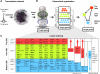
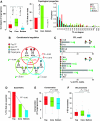
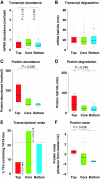
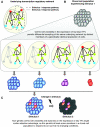
Similar articles
-
Genomic analysis of the hierarchical structure of regulatory networks.Proc Natl Acad Sci U S A. 2006 Oct 3;103(40):14724-31. doi: 10.1073/pnas.0508637103. Epub 2006 Sep 26. Proc Natl Acad Sci U S A. 2006. PMID: 17003135 Free PMC article.
-
Inferring condition-specific modulation of transcription factor activity in yeast through regulon-based analysis of genomewide expression.PLoS One. 2008 Sep 3;3(9):e3112. doi: 10.1371/journal.pone.0003112. PLoS One. 2008. PMID: 18769540 Free PMC article.
-
Mapping functional transcription factor networks from gene expression data.Genome Res. 2013 Aug;23(8):1319-28. doi: 10.1101/gr.150904.112. Epub 2013 May 1. Genome Res. 2013. PMID: 23636944 Free PMC article.
-
A conserved role for transcription factor sumoylation in binding-site selection.Curr Genet. 2019 Dec;65(6):1307-1312. doi: 10.1007/s00294-019-00992-w. Epub 2019 May 15. Curr Genet. 2019. PMID: 31093693 Review.
-
Transcription factors: Bridge between cell signaling and gene regulation.Proteomics. 2021 Dec;21(23-24):e2000034. doi: 10.1002/pmic.202000034. Epub 2021 Aug 9. Proteomics. 2021. PMID: 34314098 Review.
Cited by
-
Porcine tissue-specific regulatory networks derived from meta-analysis of the transcriptome.PLoS One. 2012;7(9):e46159. doi: 10.1371/journal.pone.0046159. Epub 2012 Sep 26. PLoS One. 2012. PMID: 23049964 Free PMC article.
-
The fitness cost and benefit of phase-separated protein deposits.Mol Syst Biol. 2019 Apr 8;15(4):e8075. doi: 10.15252/msb.20178075. Mol Syst Biol. 2019. PMID: 30962358 Free PMC article.
-
How directed is a directed network?R Soc Open Sci. 2020 Sep 9;7(9):201138. doi: 10.1098/rsos.201138. eCollection 2020 Sep. R Soc Open Sci. 2020. PMID: 33047061 Free PMC article.
-
Teasing apart translational and transcriptional components of stochastic variations in eukaryotic gene expression.PLoS Comput Biol. 2012;8(8):e1002644. doi: 10.1371/journal.pcbi.1002644. Epub 2012 Aug 30. PLoS Comput Biol. 2012. PMID: 22956896 Free PMC article.
-
Integrative analysis of cancer genes in a functional interactome.Sci Rep. 2016 Jun 30;6:29228. doi: 10.1038/srep29228. Sci Rep. 2016. PMID: 27356765 Free PMC article.
References
-
- Acar M, Mettetal JT, van Oudenaarden A (2008) Stochastic switching as a survival strategy in fluctuating environments. Nat Genet 40: 471–475 - PubMed
-
- Alon U (2007) Network motifs: theory and experimental approaches. Nat Rev Genet 8: 450–461 - PubMed
-
- Arias AM, Hayward P (2006) Filtering transcriptional noise during development: concepts and mechanisms. Nat Rev Genet 7: 34–44 - PubMed
-
- Babu MM, Luscombe NM, Aravind L, Gerstein M, Teichmann SA (2004) Structure and evolution of transcriptional regulatory networks. Curr Opin Struct Biol 14: 283–291 - PubMed
-
- Balaban NQ, Merrin J, Chait R, Kowalik L, Leibler S (2004) Bacterial persistence as a phenotypic switch. Science 305: 1622–1625 - PubMed
Publication types
MeSH terms
Substances
Grants and funding
LinkOut - more resources
Full Text Sources
Molecular Biology Databases
Miscellaneous