Balanced amplification: a new mechanism of selective amplification of neural activity patterns
- PMID: 19249282
- PMCID: PMC2667957
- DOI: 10.1016/j.neuron.2009.02.005
Balanced amplification: a new mechanism of selective amplification of neural activity patterns
Erratum in
- Neuron. 2016 Jan 6;89(1):235
Abstract
In cerebral cortex, ongoing activity absent a stimulus can resemble stimulus-driven activity in size and structure. In particular, spontaneous activity in cat primary visual cortex (V1) has structure significantly correlated with evoked responses to oriented stimuli. This suggests that, from unstructured input, cortical circuits selectively amplify specific activity patterns. Current understanding of selective amplification involves elongation of a neural assembly's lifetime by mutual excitation among its neurons. We introduce a new mechanism for selective amplification without elongation of lifetime: "balanced amplification." Strong balanced amplification arises when feedback inhibition stabilizes strong recurrent excitation, a pattern likely to be typical of cortex. Thus, balanced amplification should ubiquitously contribute to cortical activity. Balanced amplification depends on the fact that individual neurons project only excitatory or only inhibitory synapses. This leads to a hidden feedforward connectivity between activity patterns. We show in a detailed biophysical model that this can explain the cat V1 observations.
Figures
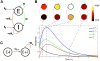
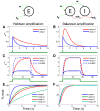
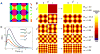
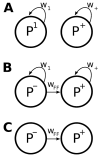
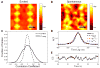
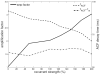
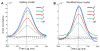
Comment in
-
Feedforward to the past: the relation between neuronal connectivity, amplification, and short-term memory.Neuron. 2009 Feb 26;61(4):499-501. doi: 10.1016/j.neuron.2009.02.006. Neuron. 2009. PMID: 19249270
Similar articles
-
Recurrent excitation in neocortical circuits.Science. 1995 Aug 18;269(5226):981-5. doi: 10.1126/science.7638624. Science. 1995. PMID: 7638624
-
Differential depression of inhibitory synaptic responses in feedforward and feedback circuits between different areas of mouse visual cortex.J Comp Neurol. 2004 Jul 26;475(3):361-73. doi: 10.1002/cne.20164. J Comp Neurol. 2004. PMID: 15221951
-
Asymmetric synaptic depression in cortical networks.Cereb Cortex. 2008 Apr;18(4):771-88. doi: 10.1093/cercor/bhm119. Epub 2007 Aug 9. Cereb Cortex. 2008. PMID: 17693394
-
Understanding layer 4 of the cortical circuit: a model based on cat V1.Cereb Cortex. 2003 Jan;13(1):73-82. doi: 10.1093/cercor/13.1.73. Cereb Cortex. 2003. PMID: 12466218 Review.
-
Bottom-up and top-down dynamics in visual cortex.Prog Brain Res. 2005;149:65-81. doi: 10.1016/S0079-6123(05)49006-8. Prog Brain Res. 2005. PMID: 16226577 Review.
Cited by
-
Stimulus-dependent variability and noise correlations in cortical MT neurons.Proc Natl Acad Sci U S A. 2013 Aug 6;110(32):13162-7. doi: 10.1073/pnas.1300098110. Epub 2013 Jul 22. Proc Natl Acad Sci U S A. 2013. PMID: 23878209 Free PMC article.
-
Single neuron firing properties impact correlation-based population coding.J Neurosci. 2012 Jan 25;32(4):1413-28. doi: 10.1523/JNEUROSCI.3735-11.2012. J Neurosci. 2012. PMID: 22279226 Free PMC article.
-
Network dynamics underlying OFF responses in the auditory cortex.Elife. 2021 Mar 24;10:e53151. doi: 10.7554/eLife.53151. Elife. 2021. PMID: 33759763 Free PMC article.
-
A layered microcircuit model of somatosensory cortex with three interneuron types and cell-type-specific short-term plasticity.Cereb Cortex. 2024 Sep 3;34(9):bhae378. doi: 10.1093/cercor/bhae378. Cereb Cortex. 2024. PMID: 39344196 Free PMC article.
-
How many neurons are sufficient for perception of cortical activity?Elife. 2020 Oct 26;9:e58889. doi: 10.7554/eLife.58889. Elife. 2020. PMID: 33103656 Free PMC article.
References
-
- Anderson JS, Carandini M, Ferster D. Orientation tuning of input conductance, excitation, and inhibition in cat primary visual cortex. J Neurophysiol. 2000a;84:909–926. - PubMed
-
- Anderson JS, Lampl I, Gillespie D, Ferster D. The contribution of noise to contrast invariance of orientation tuning in cat visual cortex. Science. 2000b;290:1968–1972. - PubMed
-
- Arieli A, Sterkin A, Grinvald A, Aertsen A. Dynamics of ongoing activity: explanation of the large variability in evoked cortical responses. Science. 1996;273:1868–1871. - PubMed
-
- Berger T, Borgdorff A, Crochet S, Neubauer F, Lefort S, Fauvet B, Ferezou I, Carleton A, Lscher H, Petersen C. Combined voltage and calcium epifiuorescence imaging in vitro and in vivo reveals subthreshold and suprathreshold dynamics of mouse barrel cortex. J Neurophysiol. 2007;97:3751–3762. - PubMed
Publication types
MeSH terms
Substances
Grants and funding
LinkOut - more resources
Full Text Sources
Other Literature Sources
Miscellaneous