A modular analysis framework for blood genomics studies: application to systemic lupus erythematosus
- PMID: 18631455
- PMCID: PMC2727981
- DOI: 10.1016/j.immuni.2008.05.012
A modular analysis framework for blood genomics studies: application to systemic lupus erythematosus
Abstract
The analysis of patient blood transcriptional profiles offers a means to investigate the immunological mechanisms relevant to human diseases on a genome-wide scale. In addition, such studies provide a basis for the discovery of clinically relevant biomarker signatures. We designed a strategy for microarray analysis that is based on the identification of transcriptional modules formed by genes coordinately expressed in multiple disease data sets. Mapping changes in gene expression at the module level generated disease-specific transcriptional fingerprints that provide a stable framework for the visualization and functional interpretation of microarray data. These transcriptional modules were used as a basis for the selection of biomarkers and the development of a multivariate transcriptional indicator of disease progression in patients with systemic lupus erythematosus. Thus, this work describes the implementation and application of a methodology designed to support systems-scale analysis of the human immune system in translational research settings.
Figures
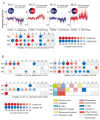
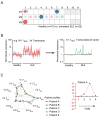
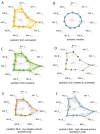
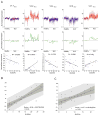
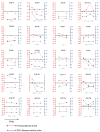
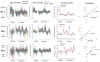
Comment in
-
Bottom up: a modular view of immunology.Immunity. 2008 Jul 18;29(1):9-11. doi: 10.1016/j.immuni.2008.07.002. Immunity. 2008. PMID: 18631452 Free PMC article.
Similar articles
-
Key genes and functional coexpression modules involved in the pathogenesis of systemic lupus erythematosus.J Cell Physiol. 2018 Nov;233(11):8815-8825. doi: 10.1002/jcp.26795. Epub 2018 May 28. J Cell Physiol. 2018. PMID: 29806703
-
Gene expression studies in systemic lupus erythematosus.Lupus. 2006;15(7):451-6. doi: 10.1191/0961203306lu2332oa. Lupus. 2006. PMID: 16898181 Review.
-
An interactive web application for exploring systemic lupus erythematosus blood transcriptomic diversity.Database (Oxford). 2024 May 28;2024:baae045. doi: 10.1093/database/baae045. Database (Oxford). 2024. PMID: 38805754 Free PMC article.
-
Microarray analysis of microRNA expression in peripheral blood cells of systemic lupus erythematosus patients.Lupus. 2007;16(12):939-46. doi: 10.1177/0961203307084158. Lupus. 2007. PMID: 18042587
-
Genomic Investigation of Lupus in the Skin.J Investig Dermatol Symp Proc. 2017 Oct;18(2):S75-S80. doi: 10.1016/j.jisp.2016.09.002. J Investig Dermatol Symp Proc. 2017. PMID: 28941499 Review.
Cited by
-
Transcriptomic studies of systemic lupus erythematosus.Inflamm Regen. 2021 Apr 9;41(1):11. doi: 10.1186/s41232-021-00161-y. Inflamm Regen. 2021. PMID: 33836832 Free PMC article. Review.
-
Double-stranded RNA induces molecular and inflammatory signatures that are directly relevant to COPD.Mucosal Immunol. 2013 May;6(3):474-84. doi: 10.1038/mi.2012.86. Epub 2012 Sep 19. Mucosal Immunol. 2013. PMID: 22990623 Free PMC article.
-
Oral neutrophil transcriptome changes result in a pro-survival phenotype in periodontal diseases.PLoS One. 2013 Jul 11;8(7):e68983. doi: 10.1371/journal.pone.0068983. Print 2013. PLoS One. 2013. PMID: 23874838 Free PMC article.
-
Signature MicroRNA expression patterns identified in humans with 22q11.2 deletion/DiGeorge syndrome.Clin Immunol. 2013 Apr;147(1):11-22. doi: 10.1016/j.clim.2013.01.011. Epub 2013 Jan 30. Clin Immunol. 2013. PMID: 23454892 Free PMC article.
-
MX2: Identification and systematic mechanistic analysis of a novel immune-related biomarker for systemic lupus erythematosus.Front Immunol. 2022 Aug 18;13:978851. doi: 10.3389/fimmu.2022.978851. eCollection 2022. Front Immunol. 2022. PMID: 36059547 Free PMC article.
References
-
- Alizadeh AA, Eisen MB, Davis RE, Ma C, Lossos IS, Rosenwald A, Boldrick JC, Sabet H, Tran T, Yu X, et al. Distinct types of diffuse large B-cell lymphoma identified by gene expression profiling. Nature. 2000;403:503–511. - PubMed
-
- Bae SC, Koh HK, Chang DK, Kim MH, Park JK, Kim SY. Reliability and validity of systemic lupus activity measure-revised (SLAM-R) for measuring clinical disease activity in systemic lupus erythematosus. Lupus. 2001;10:405–409. - PubMed
-
- Bammler T, Beyer RP, Bhattacharya S, Boorman GA, Boyles A, Bradford BU, Bumgarner RE, Bushel PR, Chaturvedi K, Choi D, et al. Standardizing global gene expression analysis between laboratories and across platforms. Nat Methods. 2005;2:351–356. - PubMed
Publication types
MeSH terms
Associated data
- Actions
Grants and funding
- U19 AI057234-04/AI/NIAID NIH HHS/United States
- R01 CA078846-07/CA/NCI NIH HHS/United States
- R01 CA078846-02/CA/NCI NIH HHS/United States
- R01 AR050770-04/AR/NIAMS NIH HHS/United States
- U19 AI057234-010005/AI/NIAID NIH HHS/United States
- P01 CA084512-069004/CA/NCI NIH HHS/United States
- U19 AI057234-02/AI/NIAID NIH HHS/United States
- U19 AI057234-05S10012/AI/NIAID NIH HHS/United States
- P50 AR054083-020001/AR/NIAMS NIH HHS/United States
- P50 AR054083-019001/AR/NIAMS NIH HHS/United States
- P01 CA084512-060005/CA/NCI NIH HHS/United States
- R01 CA078846-04/CA/NCI NIH HHS/United States
- R01 AR050770-03/AR/NIAMS NIH HHS/United States
- U19 AI057234-01/AI/NIAID NIH HHS/United States
- R01 AR050770-06/AR/NIAMS NIH HHS/United States
- P01 CA084512-01A10003/CA/NCI NIH HHS/United States
- R01 AI068842/AI/NIAID NIH HHS/United States
- P50 AR054083-010001/AR/NIAMS NIH HHS/United States
- P01 CA084512-05A29004/CA/NCI NIH HHS/United States
- P50 AR054083-030001/AR/NIAMS NIH HHS/United States
- P50 AR055503/AR/NIAMS NIH HHS/United States
- P50 AR055503-010004/AR/NIAMS NIH HHS/United States
- R01 AI068842-04/AI/NIAID NIH HHS/United States
- P01 CA084512-030003/CA/NCI NIH HHS/United States
- P01 CA084512-020003/CA/NCI NIH HHS/United States
- R01 AR050770-02/AR/NIAMS NIH HHS/United States
- P01 CA084512-05A2/CA/NCI NIH HHS/United States
- R01 CA078846-05/CA/NCI NIH HHS/United States
- R01 CA078846-08/CA/NCI NIH HHS/United States
- P01 CA084512-079004/CA/NCI NIH HHS/United States
- R01 AR050770-01/AR/NIAMS NIH HHS/United States
- U19 AI057234-05/AI/NIAID NIH HHS/United States
- R01 AI068842-03/AI/NIAID NIH HHS/United States
- P01 CA084512/CA/NCI NIH HHS/United States
- R01 CA078846-10/CA/NCI NIH HHS/United States
- R01 AI068842-02/AI/NIAID NIH HHS/United States
- P01 CA084512-06/CA/NCI NIH HHS/United States
- R01 AR050770/AR/NIAMS NIH HHS/United States
- R01 CA078846-06/CA/NCI NIH HHS/United States
- U19 AI057234-010006/AI/NIAID NIH HHS/United States
- R01 CA078846-01A1/CA/NCI NIH HHS/United States
- P50 AR055503-020004/AR/NIAMS NIH HHS/United States
- P01 CA084512-079002/CA/NCI NIH HHS/United States
- P01 CA084512-069002/CA/NCI NIH HHS/United States
- U19 AI057234-05S19003/AI/NIAID NIH HHS/United States
- U19 AI057234-06/AI/NIAID NIH HHS/United States
- R01 CA078846-09/CA/NCI NIH HHS/United States
- U19 AI057234-05S1/AI/NIAID NIH HHS/United States
- P50 AR054083-01/AR/NIAMS NIH HHS/United States
- R01 AR050770-05A1/AR/NIAMS NIH HHS/United States
- U19 AI057234/AI/NIAID NIH HHS/United States
- P01 CA084512-05A29002/CA/NCI NIH HHS/United States
- R01 AI068842-01/AI/NIAID NIH HHS/United States
- U19 AI057234-010004/AI/NIAID NIH HHS/United States
- P50 AR054083-03/AR/NIAMS NIH HHS/United States
- P50 AR054083-029001/AR/NIAMS NIH HHS/United States
- U19 AI057234-03/AI/NIAID NIH HHS/United States
- R01 I068842/PHS HHS/United States
- R01 CA078846-03/CA/NCI NIH HHS/United States
- R01 CA078846/CA/NCI NIH HHS/United States
- U19 AI057234-05S10013/AI/NIAID NIH HHS/United States
- U19 AIO57234-02/PHS HHS/United States
- P50 AR054083/AR/NIAMS NIH HHS/United States
- P50 AR054083-02/AR/NIAMS NIH HHS/United States
- P01 CA084512-070005/CA/NCI NIH HHS/United States
- P01 CA084512-07/CA/NCI NIH HHS/United States
- P01 CA084512-040003/CA/NCI NIH HHS/United States
- P01 CA084512-05A20005/CA/NCI NIH HHS/United States
LinkOut - more resources
Full Text Sources
Other Literature Sources
Medical