A comparative study of different machine learning methods on microarray gene expression data
- PMID: 18366602
- PMCID: PMC2386055
- DOI: 10.1186/1471-2164-9-S1-S13
A comparative study of different machine learning methods on microarray gene expression data
Abstract
Background: Several classification and feature selection methods have been studied for the identification of differentially expressed genes in microarray data. Classification methods such as SVM, RBF Neural Nets, MLP Neural Nets, Bayesian, Decision Tree and Random Forrest methods have been used in recent studies. The accuracy of these methods has been calculated with validation methods such as v-fold validation. However there is lack of comparison between these methods to find a better framework for classification, clustering and analysis of microarray gene expression results.
Results: In this study, we compared the efficiency of the classification methods including; SVM, RBF Neural Nets, MLP Neural Nets, Bayesian, Decision Tree and Random Forrest methods. The v-fold cross validation was used to calculate the accuracy of the classifiers. Some of the common clustering methods including K-means, DBC, and EM clustering were applied to the datasets and the efficiency of these methods have been analysed. Further the efficiency of the feature selection methods including support vector machine recursive feature elimination (SVM-RFE), Chi Squared, and CSF were compared. In each case these methods were applied to eight different binary (two class) microarray datasets. We evaluated the class prediction efficiency of each gene list in training and test cross-validation using supervised classifiers.
Conclusions: We presented a study in which we compared some of the common used classification, clustering, and feature selection methods. We applied these methods to eight publicly available datasets, and compared how these methods performed in class prediction of test datasets. We reported that the choice of feature selection methods, the number of genes in the gene list, the number of cases (samples) substantially influence classification success. Based on features chosen by these methods, error rates and accuracy of several classification algorithms were obtained. Results revealed the importance of feature selection in accurately classifying new samples and how an integrated feature selection and classification algorithm is performing and is capable of identifying significant genes.
Figures
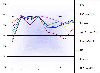
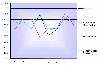
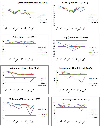
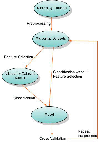
Similar articles
-
Recursive cluster elimination (RCE) for classification and feature selection from gene expression data.BMC Bioinformatics. 2007 May 2;8:144. doi: 10.1186/1471-2105-8-144. BMC Bioinformatics. 2007. PMID: 17474999 Free PMC article.
-
Recursive gene selection based on maximum margin criterion: a comparison with SVM-RFE.BMC Bioinformatics. 2006 Dec 25;7:543. doi: 10.1186/1471-2105-7-543. BMC Bioinformatics. 2006. PMID: 17187691 Free PMC article.
-
Development of two-stage SVM-RFE gene selection strategy for microarray expression data analysis.IEEE/ACM Trans Comput Biol Bioinform. 2007 Jul-Sep;4(3):365-81. doi: 10.1109/TCBB.2007.70224. IEEE/ACM Trans Comput Biol Bioinform. 2007. PMID: 17666757
-
Filter versus wrapper gene selection approaches in DNA microarray domains.Artif Intell Med. 2004 Jun;31(2):91-103. doi: 10.1016/j.artmed.2004.01.007. Artif Intell Med. 2004. PMID: 15219288 Review.
-
Optimal features selection in the high dimensional data based on robust technique: Application to different health database.Heliyon. 2024 Sep 2;10(17):e37241. doi: 10.1016/j.heliyon.2024.e37241. eCollection 2024 Sep 15. Heliyon. 2024. PMID: 39296019 Free PMC article. Review.
Cited by
-
Personal Health Information Inference Using Machine Learning on RNA Expression Data from Patients With Cancer: Algorithm Validation Study.J Med Internet Res. 2020 Aug 10;22(8):e18387. doi: 10.2196/18387. J Med Internet Res. 2020. PMID: 32773372 Free PMC article.
-
Machine Learning Classifiers for Endometriosis Using Transcriptomics and Methylomics Data.Front Genet. 2019 Sep 4;10:766. doi: 10.3389/fgene.2019.00766. eCollection 2019. Front Genet. 2019. PMID: 31552087 Free PMC article.
-
MSC-CSMC: A multi-objective semi-supervised clustering algorithm based on constraints selection and multi-source constraints for gene expression data.Front Genet. 2023 Feb 27;14:1135260. doi: 10.3389/fgene.2023.1135260. eCollection 2023. Front Genet. 2023. PMID: 36923794 Free PMC article.
-
A comparison of machine learning methods for classification using simulation with multiple real data examples from mental health studies.Stat Methods Med Res. 2016 Oct;25(5):1804-1823. doi: 10.1177/0962280213502437. Epub 2013 Sep 18. Stat Methods Med Res. 2016. PMID: 24047600 Free PMC article.
-
Automated machine learning for endemic active tuberculosis prediction from multiplex serological data.Sci Rep. 2021 Sep 9;11(1):17900. doi: 10.1038/s41598-021-97453-7. Sci Rep. 2021. PMID: 34504228 Free PMC article.
References
-
- Guyon I, Weston J, Barnhill S, Vapnik V. Gene selection for cancer classification using support vector machines. Machine Learning. 2001;46:389–422.
-
- Liu H., Setiono R. Chi2: Feature Selection and Discretization of Numeric Attributes. Proceedings of the IEEE 7th International Conference on Tools with Artificial Intelligence. 1995. pp. 338–391.
-
- Hall M. A. Correlation-based feature selection for machine learning. PhD thesis, University of Waikato, Department of Computer Science, Hamilton, New Zealand. 1998.
Publication types
MeSH terms
Grants and funding
LinkOut - more resources
Full Text Sources
Other Literature Sources