Capturing heterogeneity in gene expression studies by surrogate variable analysis
- PMID: 17907809
- PMCID: PMC1994707
- DOI: 10.1371/journal.pgen.0030161
Capturing heterogeneity in gene expression studies by surrogate variable analysis
Abstract
It has unambiguously been shown that genetic, environmental, demographic, and technical factors may have substantial effects on gene expression levels. In addition to the measured variable(s) of interest, there will tend to be sources of signal due to factors that are unknown, unmeasured, or too complicated to capture through simple models. We show that failing to incorporate these sources of heterogeneity into an analysis can have widespread and detrimental effects on the study. Not only can this reduce power or induce unwanted dependence across genes, but it can also introduce sources of spurious signal to many genes. This phenomenon is true even for well-designed, randomized studies. We introduce "surrogate variable analysis" (SVA) to overcome the problems caused by heterogeneity in expression studies. SVA can be applied in conjunction with standard analysis techniques to accurately capture the relationship between expression and any modeled variables of interest. We apply SVA to disease class, time course, and genetics of gene expression studies. We show that SVA increases the biological accuracy and reproducibility of analyses in genome-wide expression studies.
Conflict of interest statement
Competing interests. The authors have declared that no competing interests exist.
Figures
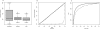
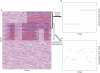
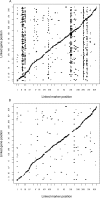
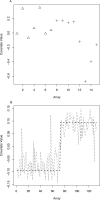
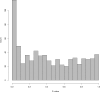
Similar articles
-
SVAw - a web-based application tool for automated surrogate variable analysis of gene expression studies.Source Code Biol Med. 2013 Mar 11;8(1):8. doi: 10.1186/1751-0473-8-8. Source Code Biol Med. 2013. PMID: 23497726 Free PMC article.
-
Surrogate variable analysis using partial least squares (SVA-PLS) in gene expression studies.Bioinformatics. 2012 Mar 15;28(6):799-806. doi: 10.1093/bioinformatics/bts022. Epub 2012 Jan 11. Bioinformatics. 2012. PMID: 22238271
-
Use of expression data and the CGEMS genome-wide breast cancer association study to identify genes that may modify risk in BRCA1/2 mutation carriers.Breast Cancer Res Treat. 2008 Nov;112(2):229-36. doi: 10.1007/s10549-007-9848-5. Epub 2007 Dec 20. Breast Cancer Res Treat. 2008. PMID: 18095154
-
Gene analysis techniques and susceptibility gene discovery in non-BRCA1/BRCA2 familial breast cancer.Surg Oncol. 2015 Jun;24(2):100-9. doi: 10.1016/j.suronc.2015.04.003. Epub 2015 Apr 13. Surg Oncol. 2015. PMID: 25936246 Review.
-
Histopathology of BRCA1- and BRCA2-associated breast cancer.Crit Rev Oncol Hematol. 2006 Jul;59(1):27-39. doi: 10.1016/j.critrevonc.2006.01.006. Epub 2006 Mar 10. Crit Rev Oncol Hematol. 2006. PMID: 16530420 Review.
Cited by
-
GBAT: a gene-based association test for robust detection of trans-gene regulation.Genome Biol. 2020 Aug 24;21(1):211. doi: 10.1186/s13059-020-02120-1. Genome Biol. 2020. PMID: 32831138 Free PMC article.
-
Oncogenic Features in Histologically Normal Mucosa: Novel Insights Into Field Effect From a Mega-Analysis of Colorectal Transcriptomes.Clin Transl Gastroenterol. 2020 Jul;11(7):e00210. doi: 10.14309/ctg.0000000000000210. Clin Transl Gastroenterol. 2020. PMID: 32764205 Free PMC article.
-
Multivariate meta-analysis reveals global transcriptomic signatures underlying distinct human naive-like pluripotent states.PLoS One. 2021 May 13;16(5):e0251461. doi: 10.1371/journal.pone.0251461. eCollection 2021. PLoS One. 2021. PMID: 33984026 Free PMC article.
-
Alzheimer's disease CSF biomarkers correlate with early pathology and alterations in neuronal and glial gene expression.Alzheimers Dement. 2024 Oct;20(10):7090-7103. doi: 10.1002/alz.14194. Epub 2024 Aug 27. Alzheimers Dement. 2024. PMID: 39192661 Free PMC article.
-
Perturbations in Neuroinflammatory Pathways Are Associated With a Worst Pain Profile in Oncology Patients Receiving Chemotherapy.J Pain. 2023 Jan;24(1):84-97. doi: 10.1016/j.jpain.2022.08.007. Epub 2022 Sep 15. J Pain. 2023. PMID: 36115520 Free PMC article.
References
-
- Klebanov L, Yakovlev A. Treating expression levels of different genes as a sample in microarray data analysis: is it worth a risk? Stat Appl Genet Mol Biol. 2006;5:art9. - PubMed
-
- Kerr MK, Martin M, Churchill GA. Analysis of variance for gene expression microarray data. J Comput Biol. 2000;7:819–837. - PubMed
-
- Kerr MK, Churchill GA. Experimental design for gene expression microarrays. Biostatistics. 2001;2:183–201. - PubMed
Publication types
MeSH terms
Grants and funding
LinkOut - more resources
Full Text Sources
Other Literature Sources
Molecular Biology Databases