Imputation-based analysis of association studies: candidate regions and quantitative traits
- PMID: 17676998
- PMCID: PMC1934390
- DOI: 10.1371/journal.pgen.0030114
Imputation-based analysis of association studies: candidate regions and quantitative traits
Abstract
We introduce a new framework for the analysis of association studies, designed to allow untyped variants to be more effectively and directly tested for association with a phenotype. The idea is to combine knowledge on patterns of correlation among SNPs (e.g., from the International HapMap project or resequencing data in a candidate region of interest) with genotype data at tag SNPs collected on a phenotyped study sample, to estimate ("impute") unmeasured genotypes, and then assess association between the phenotype and these estimated genotypes. Compared with standard single-SNP tests, this approach results in increased power to detect association, even in cases in which the causal variant is typed, with the greatest gain occurring when multiple causal variants are present. It also provides more interpretable explanations for observed associations, including assessing, for each SNP, the strength of the evidence that it (rather than another correlated SNP) is causal. Although we focus on association studies with quantitative phenotype and a relatively restricted region (e.g., a candidate gene), the framework is applicable and computationally practical for whole genome association studies. Methods described here are implemented in a software package, Bim-Bam, available from the Stephens Lab website http://stephenslab.uchicago.edu/software.html.
Conflict of interest statement
Competing interests. The authors have declared that no competing interests exist.
Figures
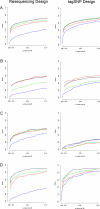
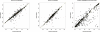
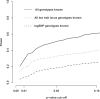
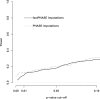
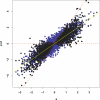
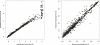
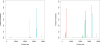
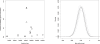
Similar articles
-
Practical issues in imputation-based association mapping.PLoS Genet. 2008 Dec;4(12):e1000279. doi: 10.1371/journal.pgen.1000279. Epub 2008 Dec 5. PLoS Genet. 2008. PMID: 19057666 Free PMC article.
-
Exploiting biological priors and sequence variants enhances QTL discovery and genomic prediction of complex traits.BMC Genomics. 2016 Feb 27;17:144. doi: 10.1186/s12864-016-2443-6. BMC Genomics. 2016. PMID: 26920147 Free PMC article.
-
Tag SNP selection for candidate gene association studies using HapMap and gene resequencing data.Eur J Hum Genet. 2007 Oct;15(10):1063-70. doi: 10.1038/sj.ejhg.5201875. Epub 2007 Jun 13. Eur J Hum Genet. 2007. PMID: 17568388
-
MLR-tagging: informative SNP selection for unphased genotypes based on multiple linear regression.Bioinformatics. 2006 Oct 15;22(20):2558-61. doi: 10.1093/bioinformatics/btl420. Epub 2006 Aug 7. Bioinformatics. 2006. PMID: 16895924
-
Tag SNP selection for association studies.Genet Epidemiol. 2004 Dec;27(4):365-74. doi: 10.1002/gepi.20028. Genet Epidemiol. 2004. PMID: 15372618 Review.
Cited by
-
Structural variants contribute to phenotypic variation in maize.bioRxiv [Preprint]. 2024 Dec 3:2024.06.14.599082. doi: 10.1101/2024.06.14.599082. bioRxiv. 2024. PMID: 38948717 Free PMC article. Preprint.
-
Estimation of inbreeding and kinship coefficients via latent identity-by-descent states.Bioinformatics. 2024 Feb 1;40(2):btae082. doi: 10.1093/bioinformatics/btae082. Bioinformatics. 2024. PMID: 38364309 Free PMC article.
-
Accurate and adaptive imputation of summary statistics in mixed-ethnicity cohorts.Bioinformatics. 2018 Sep 1;34(17):i687-i696. doi: 10.1093/bioinformatics/bty596. Bioinformatics. 2018. PMID: 30423082 Free PMC article.
-
Novel associations of multiple genetic loci with plasma levels of factor VII, factor VIII, and von Willebrand factor: The CHARGE (Cohorts for Heart and Aging Research in Genome Epidemiology) Consortium.Circulation. 2010 Mar 30;121(12):1382-92. doi: 10.1161/CIRCULATIONAHA.109.869156. Epub 2010 Mar 15. Circulation. 2010. PMID: 20231535 Free PMC article.
-
The Bayesian lens and Bayesian blinkers.Philos Trans A Math Phys Eng Sci. 2023 May 15;381(2247):20220144. doi: 10.1098/rsta.2022.0144. Epub 2023 Mar 27. Philos Trans A Math Phys Eng Sci. 2023. PMID: 36970830 Free PMC article. Review.
References
-
- SeattleSNPs. Seattle (Washington): NHLBI Program for Genomic Applications; Available: http://pga.gs.washington.edu. Accessed 12 June 2007.
-
- Kraft P, Pharoah P, Chanock SJ, Albanes D, Kolonel LN, et al. Genetic variation in the HSD17B1 gene and risk of prostate cancer. PLoS Genet. 2005;1:e68. doi: 10.1371/journal.pgen.0010068. - DOI - PMC - PubMed
Publication types
MeSH terms
Grants and funding
LinkOut - more resources
Full Text Sources
Miscellaneous