DNA familial binding profiles made easy: comparison of various motif alignment and clustering strategies
- PMID: 17397256
- PMCID: PMC1848003
- DOI: 10.1371/journal.pcbi.0030061
DNA familial binding profiles made easy: comparison of various motif alignment and clustering strategies
Abstract
Transcription factor (TF) proteins recognize a small number of DNA sequences with high specificity and control the expression of neighbouring genes. The evolution of TF binding preference has been the subject of a number of recent studies, in which generalized binding profiles have been introduced and used to improve the prediction of new target sites. Generalized profiles are generated by aligning and merging the individual profiles of related TFs. However, the distance metrics and alignment algorithms used to compare the binding profiles have not yet been fully explored or optimized. As a result, binding profiles depend on TF structural information and sometimes may ignore important distinctions between subfamilies. Prediction of the identity or the structural class of a protein that binds to a given DNA pattern will enhance the analysis of microarray and ChIP-chip data where frequently multiple putative targets of usually unknown TFs are predicted. Various comparison metrics and alignment algorithms are evaluated (a total of 105 combinations). We find that local alignments are generally better than global alignments at detecting eukaryotic DNA motif similarities, especially when combined with the sum of squared distances or Pearson's correlation coefficient comparison metrics. In addition, multiple-alignment strategies for binding profiles and tree-building methods are tested for their efficiency in constructing generalized binding models. A new method for automatic determination of the optimal number of clusters is developed and applied in the construction of a new set of familial binding profiles which improves upon TF classification accuracy. A software tool, STAMP, is developed to host all tested methods and make them publicly available. This work provides a high quality reference set of familial binding profiles and the first comprehensive platform for analysis of DNA profiles. Detecting similarities between DNA motifs is a key step in the comparative study of transcriptional regulation, and the work presented here will form the basis for tool and method development for future transcriptional modeling studies.
Conflict of interest statement
Figures
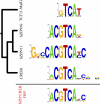
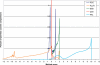
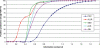
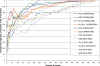
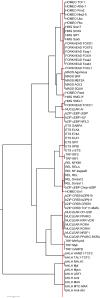
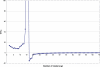
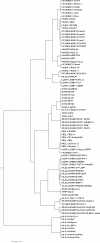
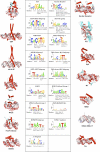
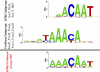
Similar articles
-
STAMP: a web tool for exploring DNA-binding motif similarities.Nucleic Acids Res. 2007 Jul;35(Web Server issue):W253-8. doi: 10.1093/nar/gkm272. Epub 2007 May 3. Nucleic Acids Res. 2007. PMID: 17478497 Free PMC article.
-
Informative priors based on transcription factor structural class improve de novo motif discovery.Bioinformatics. 2006 Jul 15;22(14):e384-92. doi: 10.1093/bioinformatics/btl251. Bioinformatics. 2006. PMID: 16873497
-
Sequence features of DNA binding sites reveal structural class of associated transcription factor.Bioinformatics. 2006 Jan 15;22(2):157-63. doi: 10.1093/bioinformatics/bti731. Epub 2005 Nov 2. Bioinformatics. 2006. PMID: 16267080
-
A survey of DNA motif finding algorithms.BMC Bioinformatics. 2007 Nov 1;8 Suppl 7(Suppl 7):S21. doi: 10.1186/1471-2105-8-S7-S21. BMC Bioinformatics. 2007. PMID: 18047721 Free PMC article. Review.
-
DNA Motif Databases and Their Uses.Curr Protoc Bioinformatics. 2015 Sep 3;51:2.15.1-2.15.6. doi: 10.1002/0471250953.bi0215s51. Curr Protoc Bioinformatics. 2015. PMID: 26334922 Review.
Cited by
-
A Monte Carlo-based framework enhances the discovery and interpretation of regulatory sequence motifs.BMC Bioinformatics. 2012 Nov 27;13:317. doi: 10.1186/1471-2105-13-317. BMC Bioinformatics. 2012. PMID: 23181585 Free PMC article.
-
A reference map of murine cardiac transcription factor chromatin occupancy identifies dynamic and conserved enhancers.Nat Commun. 2019 Oct 28;10(1):4907. doi: 10.1038/s41467-019-12812-3. Nat Commun. 2019. PMID: 31659164 Free PMC article.
-
Gene expression profile of the hippocampus of rats subjected to chronic immobilization stress.PLoS One. 2013;8(3):e57621. doi: 10.1371/journal.pone.0057621. Epub 2013 Mar 27. PLoS One. 2013. PMID: 23544040 Free PMC article.
-
High resolution genome wide binding event finding and motif discovery reveals transcription factor spatial binding constraints.PLoS Comput Biol. 2012;8(8):e1002638. doi: 10.1371/journal.pcbi.1002638. Epub 2012 Aug 9. PLoS Comput Biol. 2012. PMID: 22912568 Free PMC article.
-
Sequential in cis mutagenesis in vivo reveals various functions for CTCF sites at the mouse HoxD cluster.Genes Dev. 2021 Nov 1;35(21-22):1490-1509. doi: 10.1101/gad.348934.121. Epub 2021 Oct 28. Genes Dev. 2021. PMID: 34711654 Free PMC article.
References
-
- Stormo GD. DNA binding sites: Representation and discovery. Bioinformatics. 2000;16:16–23. - PubMed
-
- Rudolph MJ, Gergen JP. DNA-binding by Ig-fold proteins. Nat Struct Biol. 2001;8:384–386. - PubMed
-
- Pabo CO, Peisach E, Grant RA. Design and selection of novel Cys2His2 zinc finger proteins. Annu Rev Biochem. 2001;70:313–340. - PubMed
-
- Auron PE. DNA sequence-specific transcription factors. In: Lotze MT, Thomson AW, editors. Measuring immunity: Basic science and clinical practice. 1st edition. London: Elsevier; 2004. pp. 91–109.
Publication types
MeSH terms
Substances
Associated data
- Actions
- Actions
- Actions
- Actions
- Actions
- Actions
- Actions
- Actions
- Actions
- Actions
- Actions
- Actions
- Actions
Grants and funding
LinkOut - more resources
Full Text Sources
Research Materials
Miscellaneous