Evaluation of features for catalytic residue prediction in novel folds
- PMID: 17189479
- PMCID: PMC2203287
- DOI: 10.1110/ps.062523907
Evaluation of features for catalytic residue prediction in novel folds
Abstract
Structural genomics projects are determining the three-dimensional structure of proteins without full characterization of their function. A critical part of the annotation process involves appropriate knowledge representation and prediction of functionally important residue environments. We have developed a method to extract features from sequence, sequence alignments, three-dimensional structure, and structural environment conservation, and used support vector machines to annotate homologous and nonhomologous residue positions based on a specific training set of residue functions. In order to evaluate this pipeline for automated protein annotation, we applied it to the challenging problem of prediction of catalytic residues in enzymes. We also ranked the features based on their ability to discriminate catalytic from noncatalytic residues. When applying our method to a well-annotated set of protein structures, we found that top-ranked features were a measure of sequence conservation, a measure of structural conservation, a degree of uniqueness of a residue's structural environment, solvent accessibility, and residue hydrophobicity. We also found that features based on structural conservation were complementary to those based on sequence conservation and that they were capable of increasing predictor performance. Using a family nonredundant version of the ASTRAL 40 v1.65 data set, we estimated that the true catalytic residues were correctly predicted in 57.0% of the cases, with a precision of 18.5%. When testing on proteins containing novel folds not used in training, the best features were highly correlated with the training on families, thus validating the approach to nonhomologous catalytic residue prediction in general. We then applied the method to 2781 coordinate files from the structural genomics target pipeline and identified both highly ranked and highly clustered groups of predicted catalytic residues.
Figures
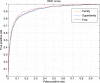
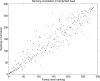
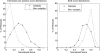
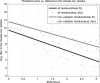
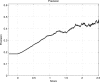
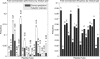
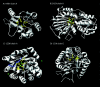
Similar articles
-
Prediction of beta-turns at over 80% accuracy based on an ensemble of predicted secondary structures and multiple alignments.BMC Bioinformatics. 2008 Oct 10;9:430. doi: 10.1186/1471-2105-9-430. BMC Bioinformatics. 2008. PMID: 18847492 Free PMC article.
-
CSmetaPred: a consensus method for prediction of catalytic residues.BMC Bioinformatics. 2017 Dec 22;18(1):583. doi: 10.1186/s12859-017-1987-z. BMC Bioinformatics. 2017. PMID: 29273005 Free PMC article.
-
Accurate sequence-based prediction of catalytic residues.Bioinformatics. 2008 Oct 15;24(20):2329-38. doi: 10.1093/bioinformatics/btn433. Epub 2008 Aug 18. Bioinformatics. 2008. PMID: 18710875
-
Structural protein descriptors in 1-dimension and their sequence-based predictions.Curr Protein Pept Sci. 2011 Sep;12(6):470-89. doi: 10.2174/138920311796957711. Curr Protein Pept Sci. 2011. PMID: 21787299 Review.
-
Advances and pitfalls in protein structure prediction.Curr Protein Pept Sci. 2008 Dec;9(6):567-77. doi: 10.2174/138920308786733958. Curr Protein Pept Sci. 2008. PMID: 19075747 Review.
Cited by
-
Predicting protein ligand binding sites by combining evolutionary sequence conservation and 3D structure.PLoS Comput Biol. 2009 Dec;5(12):e1000585. doi: 10.1371/journal.pcbi.1000585. Epub 2009 Dec 4. PLoS Comput Biol. 2009. PMID: 19997483 Free PMC article.
-
Sequence conservation in the prediction of catalytic sites.Protein J. 2011 Apr;30(4):229-39. doi: 10.1007/s10930-011-9324-2. Protein J. 2011. PMID: 21465136
-
Protein structure based prediction of catalytic residues.BMC Bioinformatics. 2013 Feb 22;14:63. doi: 10.1186/1471-2105-14-63. BMC Bioinformatics. 2013. PMID: 23433045 Free PMC article.
-
Enhanced performance in prediction of protein active sites with THEMATICS and support vector machines.Protein Sci. 2008 Feb;17(2):333-41. doi: 10.1110/ps.073213608. Epub 2007 Dec 20. Protein Sci. 2008. PMID: 18096640 Free PMC article.
-
Protein meta-functional signatures from combining sequence, structure, evolution, and amino acid property information.PLoS Comput Biol. 2008 Sep 26;4(9):e1000181. doi: 10.1371/journal.pcbi.1000181. PLoS Comput Biol. 2008. PMID: 18818722 Free PMC article.
References
-
- Aloy, P., Querol, E., Aviles, F.X., and Sternberg, M.J. 2001. Automated structure-based prediction of functional sites in proteins: Applications to assessing the validity of inheriting protein function from homology in genome annotation and to protein docking. J. Mol. Biol. 311: 395–408. - PubMed
-
- Bartlett, G.J., Porter, C.T., Borkakoti, N., and Thornton, J.M. 2002. Analysis of catalytic residues in enzyme active sites. J. Mol. Biol. 324: 105–121. - PubMed
Publication types
MeSH terms
Substances
Grants and funding
LinkOut - more resources
Full Text Sources