Malaria resurgence in the East African highlands: temperature trends revisited
- PMID: 16571662
- PMCID: PMC1416896
- DOI: 10.1073/pnas.0508929103
Malaria resurgence in the East African highlands: temperature trends revisited
Abstract
The incidence of malaria in the East African highlands has increased since the end of the 1970s. The role of climate change in the exacerbation of the disease has been controversial, and the specific influence of rising temperature (warming) has been highly debated following a previous study reporting no evidence to support a trend in temperature. We revisit this result using the same temperature data, now updated to the present from 1950 to 2002 for four high-altitude sites in East Africa where malaria has become a serious public health problem. With both nonparametric and parametric statistical analyses, we find evidence for a significant warming trend at all sites. To assess the biological significance of this trend, we drive a dynamical model for the population dynamics of the mosquito vector with the temperature time series and the corresponding detrended versions. This approach suggests that the observed temperature changes would be significantly amplified by the mosquito population dynamics with a difference in the biological response at least 1 order of magnitude larger than that in the environmental variable. Our results emphasize the importance of considering not just the statistical significance of climate trends but also their biological implications with dynamical models.
Conflict of interest statement
Conflict of interest statement: No conflicts declared.
Figures
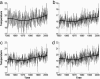
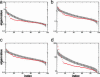
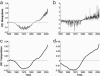
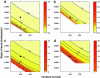
Comment in
-
Malaria risk and temperature: influences from global climate change and local land use practices.Proc Natl Acad Sci U S A. 2006 Apr 11;103(15):5635-6. doi: 10.1073/pnas.0601493103. Epub 2006 Apr 4. Proc Natl Acad Sci U S A. 2006. PMID: 16595623 Free PMC article. No abstract available.
Similar articles
-
Climate change and the resurgence of malaria in the East African highlands.Nature. 2002 Feb 21;415(6874):905-9. doi: 10.1038/415905a. Nature. 2002. PMID: 11859368 Free PMC article.
-
Malaria in the African highlands: past, present and future.Bull World Health Organ. 1998;76(1):33-45. Bull World Health Organ. 1998. PMID: 9615495 Free PMC article. Review.
-
Hot topic or hot air? Climate change and malaria resurgence in East African highlands.Trends Parasitol. 2002 Dec;18(12):530-4. doi: 10.1016/s1471-4922(02)02374-7. Trends Parasitol. 2002. PMID: 12482536 Free PMC article.
-
Climate variability and malaria epidemics in the highlands of East Africa.Trends Parasitol. 2005 Feb;21(2):54-6. doi: 10.1016/j.pt.2004.11.002. Trends Parasitol. 2005. PMID: 15664525 Review.
-
Association between climate variability and malaria epidemics in the East African highlands.Proc Natl Acad Sci U S A. 2004 Feb 24;101(8):2375-80. doi: 10.1073/pnas.0308714100. Proc Natl Acad Sci U S A. 2004. PMID: 14983017 Free PMC article.
Cited by
-
Bayesian spatio-temporal modelling of environmental, climatic, and socio-economic influences on malaria in Central Vietnam.Malar J. 2024 Aug 24;23(1):258. doi: 10.1186/s12936-024-05074-y. Malar J. 2024. PMID: 39182127 Free PMC article.
-
Estimating the effects of temperature on transmission of the human malaria parasite, Plasmodium falciparum.Nat Commun. 2024 Apr 22;15(1):3230. doi: 10.1038/s41467-024-47265-w. Nat Commun. 2024. PMID: 38649361 Free PMC article.
-
Spatial spillovers of violent conflict amplify the impacts of climate variability on malaria risk in sub-Saharan Africa.Proc Natl Acad Sci U S A. 2024 Apr 9;121(15):e2309087121. doi: 10.1073/pnas.2309087121. Epub 2024 Apr 1. Proc Natl Acad Sci U S A. 2024. PMID: 38557184 Free PMC article.
-
Analysing the six-year malaria trends at Metehara Health Centre in Central Ethiopia: the impact of resurgence on the 2030 elimination goals.Malar J. 2024 Jan 23;23(1):32. doi: 10.1186/s12936-024-04854-w. Malar J. 2024. PMID: 38263087 Free PMC article.
-
Ecological Niche Modeling of Aedes and Culex Mosquitoes: A Risk Map for Chikungunya and West Nile Viruses in Zambia.Viruses. 2023 Sep 8;15(9):1900. doi: 10.3390/v15091900. Viruses. 2023. PMID: 37766306 Free PMC article.
References
-
- Intergovernmental Panel on Climate Change. Climate Change 2001: Third Assessment Report. Vol. II. Cambridge, U.K.: Cambridge Univ. Press; 2001.
-
- Charron D. F., Thomas M. K., Waltner-Toews D., Aramini J. J., Edge T., Kent R. A., Maarouf A.R., Wilson J. J. Toxicol. Environ. Health. 2004;67:1667–1677. - PubMed
-
- Githeko A. K., Woodward A. In: Climate Change and Human Health. McMichael A. J., Campbell-Lendrum D. H., Corvalán C. F., Ebi K. L., Githeko A., Scheraga J. D., Woodward A., editors. Geneva: W.H.O.; 2003. pp. 43–60.
Publication types
MeSH terms
Grants and funding
LinkOut - more resources
Full Text Sources
Medical