Pathway level analysis of gene expression using singular value decomposition
- PMID: 16156896
- PMCID: PMC1261155
- DOI: 10.1186/1471-2105-6-225
Pathway level analysis of gene expression using singular value decomposition
Abstract
Background: A promising direction in the analysis of gene expression focuses on the changes in expression of specific predefined sets of genes that are known in advance to be related (e.g., genes coding for proteins involved in cellular pathways or complexes). Such an analysis can reveal features that are not easily visible from the variations in the individual genes and can lead to a picture of expression that is more biologically transparent and accessible to interpretation. In this article, we present a new method of this kind that operates by quantifying the level of 'activity' of each pathway in different samples. The activity levels, which are derived from singular value decompositions, form the basis for statistical comparisons and other applications.
Results: We demonstrate our approach using expression data from a study of type 2 diabetes and another of the influence of cigarette smoke on gene expression in airway epithelia. A number of interesting pathways are identified in comparisons between smokers and non-smokers including ones related to nicotine metabolism, mucus production, and glutathione metabolism. A comparison with results from the related approach, 'gene-set enrichment analysis', is also provided.
Conclusion: Our method offers a flexible basis for identifying differentially expressed pathways from gene expression data. The results of a pathway-based analysis can be complementary to those obtained from one more focused on individual genes. A web program PLAGE (Pathway Level Analysis of Gene Expression) for performing the kinds of analyses described here is accessible at http://dulci.biostat.edu/pathways.
Figures
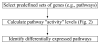
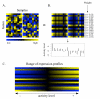
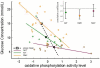
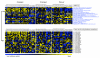
Similar articles
-
RNA-sequencing across three matched tissues reveals shared and tissue-specific gene expression and pathway signatures of COPD.Respir Res. 2019 Apr 2;20(1):65. doi: 10.1186/s12931-019-1032-z. Respir Res. 2019. PMID: 30940135 Free PMC article.
-
YGA: identifying distinct biological features between yeast gene sets.Gene. 2013 Apr 10;518(1):26-34. doi: 10.1016/j.gene.2012.11.089. Epub 2012 Dec 22. Gene. 2013. PMID: 23266802
-
Dissecting the cellular specificity of smoking effects and reconstructing lineages in the human airway epithelium.Nat Commun. 2020 May 19;11(1):2485. doi: 10.1038/s41467-020-16239-z. Nat Commun. 2020. PMID: 32427931 Free PMC article.
-
Transcriptome-wide profiling discover: PM2.5 aggravates airway dysfunction through epithelial barrier damage regulated by Stanniocalcin 2 in an OVA-induced model.Ecotoxicol Environ Saf. 2021 Sep 1;220:112408. doi: 10.1016/j.ecoenv.2021.112408. Epub 2021 Jun 8. Ecotoxicol Environ Saf. 2021. PMID: 34111662
-
Candidate genes and late-onset type 2 diabetes mellitus. Susceptibility genes or common polymorphisms?Dan Med Bull. 2003 Nov;50(4):320-46. Dan Med Bull. 2003. PMID: 14694850 Review.
Cited by
-
Single sample pathway analysis in metabolomics: performance evaluation and application.BMC Bioinformatics. 2022 Nov 14;23(1):481. doi: 10.1186/s12859-022-05005-1. BMC Bioinformatics. 2022. PMID: 36376837 Free PMC article.
-
Unbiased discovery of cancer pathways and therapeutics using Pathway Ensemble Tool and Benchmark.Nat Commun. 2024 Aug 24;15(1):7288. doi: 10.1038/s41467-024-51859-9. Nat Commun. 2024. PMID: 39179644 Free PMC article.
-
mitch: multi-contrast pathway enrichment for multi-omics and single-cell profiling data.BMC Genomics. 2020 Jun 29;21(1):447. doi: 10.1186/s12864-020-06856-9. BMC Genomics. 2020. PMID: 32600408 Free PMC article.
-
MOPA: An integrative multi-omics pathway analysis method for measuring omics activity.PLoS One. 2023 Mar 16;18(3):e0278272. doi: 10.1371/journal.pone.0278272. eCollection 2023. PLoS One. 2023. PMID: 36928437 Free PMC article.
-
Identification of diagnostic subnetwork markers for cancer in human protein-protein interaction network.BMC Bioinformatics. 2010 Oct 7;11 Suppl 6(Suppl 6):S8. doi: 10.1186/1471-2105-11-S6-S8. BMC Bioinformatics. 2010. PMID: 20946619 Free PMC article.
References
-
- Efron B, Tibshirani R, Storey JD, Tusher V. Empirical Bayes analysis of a microarray experiment. J Amer Statist Assoc. 2001;96:1151–1160. doi: 10.1198/016214501753382129. - DOI
-
- Ashburner M, Ball CA, Blake JA, Botstein D, Butler H, Cherry JM, Davis AP, Dolinski K, Dwight SS, Eppig JT, Harris MA, Hill DP, Issel-Tarver L, Kasarskis A, Lewis S, Matese JC, Richardson JE, Ringwald M, Rubin GM, Sherlock G. Gene ontology: tool for the unification of biology. The Gene Ontology Consortium. Nat Genet. 2000;25:25–9. doi: 10.1038/75556. - DOI - PMC - PubMed
Publication types
MeSH terms
Substances
Grants and funding
LinkOut - more resources
Full Text Sources
Other Literature Sources
Medical